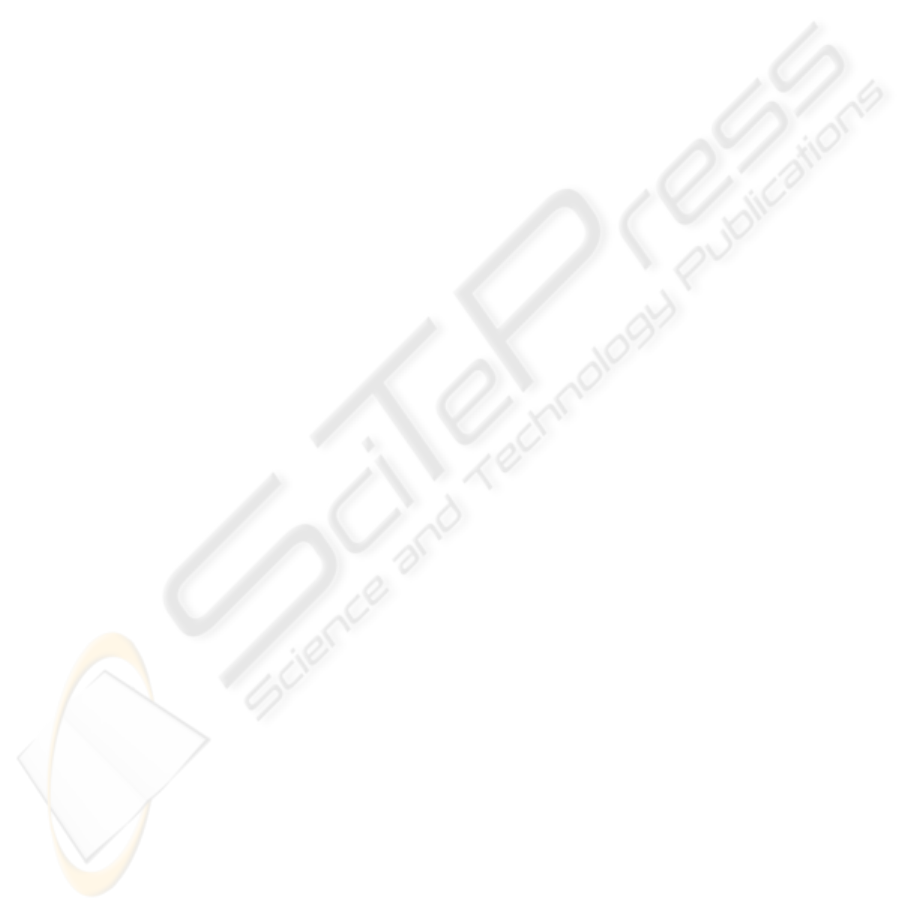
7 CONCLUSION
In this paper, we presented a complete Dirac mixture
filter that is based on the approximation of the poste-
rior density. The filter makes use of the properties of
the Dirac mixture approximation wherever they are
required, but does not deny the continuous charac-
ter of the true density. This can especially be seen
after each prediction step, where the full continuous
density representation is used.
The new approach is natural, mathematically rig-
orous, and based on an efficient algorithms (Schrempf
et al., 2006a)(Schrempf et al., 2006b) for the op-
timal approximation of arbitrary densities by Dirac
mixtures with respect to a given distance.
Compared to a particle filter, the proposed method
has several advantages. First, the Dirac components
are systematically placed in order to minimize a given
distance measure, which is selected in such a way that
the future evolution of approximate densities is al-
ways close to the true density while also considering
the actual measurements. As a result, very few sam-
ples are sufficient for achieving an excellent estima-
tion quality. Second, the optimization does not only
include the parameters of the Dirac mixture approxi-
mation, i.e., weights and locations, but also the num-
ber of components. As a result, the number of compo-
nents is automatically adjusted according to the com-
plexity of the underlying true distribution and the sup-
port area of a given likelihood. Third, as the approxi-
mation is fully deterministic, it guarantees repeatable
results.
Compared to the Unscented Kalman Filter, the
Dirac mixture filter has the advantage, that it is not
restricted to first and second order moments. Hence,
multi-modal densities, which cannot be described suf-
ficiently by using only the first two moments, can
be treated very efficiently. Such densties occur quite
often in strongly nonlinear systems. Furthermore,
no assumptions on the joint distribution of state and
measurement have to be made.
REFERENCES
Alspach, D. L. and Sorenson, H. W. (1972). Nonlinear
Bayesian Estimation Using Gaussian Sum Approxi-
mation. IEEE Transactions on Automatic Control,
AC–17(4):439–448.
Boos, D. D. (1981). Minimum Distance Estimators for Lo-
cation and Goodness of Fit. Journal of the American
Statistical association, 76(375):663–670.
Bucy, R. S. (1969). Bayes Theorem and Digital Realiza-
tions for Non-Linear Filters. Journal of Astronautical
Sciences, 17:80–94.
Doucet, A., Freitas, N. D., and Gordon, N. (2001). Se-
quential Monte Carlo Methods in Practice. Springer-
Verlag, New York.
Doucet, A., Godsill, S., and Andrieu, C. (2000). On Se-
quential Monte Carlo Sampling Methods for Bayesian
Filtering. Statistics and Computing, 10(3):197–208.
Geweke, J. (1989). Bayesian Inference in Econometric
Models using Monte Carlo Integration. Econometrica,
24:1317–1399.
Gordon, N. (1993). Bayesian Methods for Tracking. PhD
thesis, University of London.
Hanebeck, U. D., Briechle, K., and Rauh, A. (2003). Pro-
gressive Bayes: A New Framework for Nonlinear
State Estimation. In Proceedings of SPIE, volume
5099, pages 256–267, Orlando, Florida. AeroSense
Symposium.
Huber, M., Brunn, D., and Hanebeck, U. D. (2006). Closed-
Form Prediction of Nonlinear Dynamic Systems by
Means of Gaussian Mixture Approximation of the
Transition Density. In International Conference on
Multisensor Fusion and Integration for Intelligent
Systems (MFI 2006), Heidelberg, Deutschland, pages
98–103.
Huber, M. F. and Hanebeck, U. D. (2007). Hybrid Tran-
sition Density Approximation for Efficient Recursive
Prediction of Nonlinear Dynamic Systems. In In-
ternational Conference on Information Processing in
Sensor Networks (IPSN 2007), Cambridge, USA.
Julier, S. and Uhlmann, J. (1997). A New Extension of
the Kalman Filter to Nonlinear Systems. In Proceed-
ings of SPIE AeroSense, 11th International Sympo-
sium on Aerospace/Defense Sensing, Simulation, and
Controls, Orlando, FL.
Kullback, S. and Leibler, R. A. (1951). On Information
and Sufficiency. Annals of Mathematical Statistics,
22(2):79–86.
Schrempf, O. C., Brunn, D., and Hanebeck, U. D. (2006a).
Density Approximation Based on Dirac Mixtures with
Regard to Nonlinear Estimation and Filtering. In Pro-
ceedings of the 45th IEEE Conference on Decision
and Control (CDC’06), San Diego, California, USA.
Schrempf, O. C., Brunn, D., and Hanebeck, U. D. (2006b).
Dirac Mixture Density Approximation Based on Min-
imization of the Weighted Cram
´
er–von Mises Dis-
tance. In Proceedings of the International Confer-
ence on Multisensor Fusion and Integration for In-
telligent Systems (MFI 2006), Heidelberg, Germany,
pages 512–517.
Schrempf, O. C. and Hanebeck, U. D. (2007). Recursive
Prediction of Stochastic Nonlinear Systems Based on
Dirac Mixture Approximations. In Proceedings of the
American Control Conference (ACC ’07), New York
City, USA.
A STATE ESTIMATOR FOR NONLINEAR STOCHASTIC SYSTEMS BASED ON DIRAC MIXTURE
APPROXIMATIONS
61