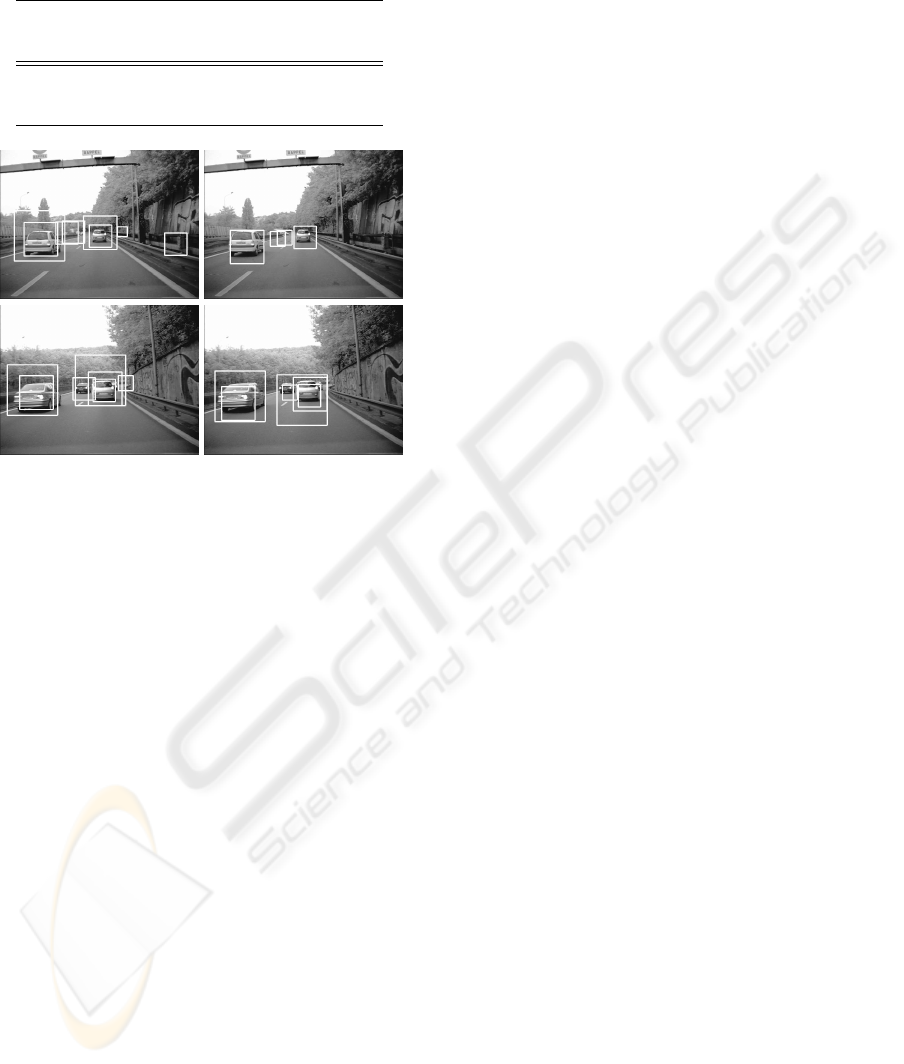
Table 2: Multi stage detection rate (Haar and HoG classi-
fiers).
Classifier Stages # Fts # Neg DR (%) FP t (seg)
Haar 12 306 1000 94.3 598 0.75
Haar 12 332 2000 94 490 0.71
Haar 12 386 3000 93,5 445 0.59
HoG 12 147 1000 96.5 935 0.51
HoG 12 176 2000 96.1 963 0.59
HoG 11 192 3000 96.6 954 0.55
(a) (b)
Figure 6: Detection results for (a) HoG and (b) Haar Multi
Stage detectors.
Table 2 shows results of cascade detectors using
Haar and HoG based features. We also tested the
effect of increasing the size of the negative set in
each training stage. The behavior of each detector is
the same as described before. HoG detector try to
construct a finer vehicle model to take into account
the new negatives. The number of features used in-
creases as the model refines. But the detection rate
and the number of false positives does not change
significantly. Haar detector refines the fronteer using
somemore features and the number of false positives
decreases while the detection keeps quite stable. Fig-
ure 5 shows the ROC curves for each detector applied
for the last stage in the cascade. For a given detection
rate, these curves show a similar behavior as the sin-
gle stage detector, where the number of false positives
is lower for the Haar classifiers than for the HoG clas-
sifiers; except for the HoG detector trained with 3000
negatives, which has a similar behavior with a half
quantity of features (see table 2). Figure 6 presents
some detection results and false alarms.
5 CONCLUSION
This communication deals with a benchmark com-
paring Haar-like features and Histograms of Oriented
Gradients features applied to vehicle detection. These
features are used in a classification algorithm based
on Adaboost. Two strategies are implemented: a sin-
gle stage detector and a multi-stage detector. The
tests - applied on realistic on-road images - show two
different results: for the HoG (generative) features,
when the number of considered features increases, the
detection rate increases while the number of false pos-
itives keeps stable; for the Haar-like (discriminative)
features, the number of false positives decreases. Fu-
ture works will be oriented to combined these behav-
iors. An approach could be build using simultane-
ously both feature types. We should also select rele-
vant features.
ACKNOWLEDGEMENTS
This research was supported by PSA Peugeot Citro
¨
en.
The authors would liko to thank Fabien Hernan-
dez from PCA Direction de la Recherche et de
l’Innovation Automobile for their help with the data
collection.
REFERENCES
Agarwal, S., Awan, A., and Roth, D. (2004). Learning to
detect objects in images via a sparse, part-based rep-
resentation. IEEE Transactions on Pattern Analysis
and Machine Intelligence, 26(11):1475–1490.
Bensrhair, A., Bertozzi, M., Broggi, A., Miche, P., Mousset,
S., and Toulminet, G. (2001). A cooperative approach
to vision-based vehicle detection. In Proceedings on
Intelligent Transportation Systems, pages 207–212.
Bertozzi, M. and Broggi, A. (1997). Vision-based vehicle
guidance. Computer, 30(7):49–55.
Bertozzi, M., Broggi, A., and Castelluccio, S. (1997). A
real-time oriented system for vehicle detection. Jour-
nal of Systems Architecture, 43(1-5):317–325.
Bucher, T., Curio, C., Edelbrunner, J., Igel, C., Kastrup,
D., Leefken, I., Lorenz, G., Steinhage, A., and von
Seelen, W. (2003). Image processing and behavior
planning for intelligent vehicles. IEEE Transactions
on Industrial Electronics, 50(1):62–75.
Cha, S. and Srihari, S. N. (2002). On measuring the
distance between histograms. Pattern Recognition,
35(6):1355–1370.
Collado, J., Hilario, C., de la Escalera, A., and Armingol, J.
(2004). Model based vehicle detection for intelligent
vehicles. In International Symposium on Intelligent
Vehicles, pages 572–577.
BENCHMARKING HAAR AND HISTOGRAMS OF ORIENTED GRADIENTS FEATURES APPLIED TO VEHICLE
DETECTION
363