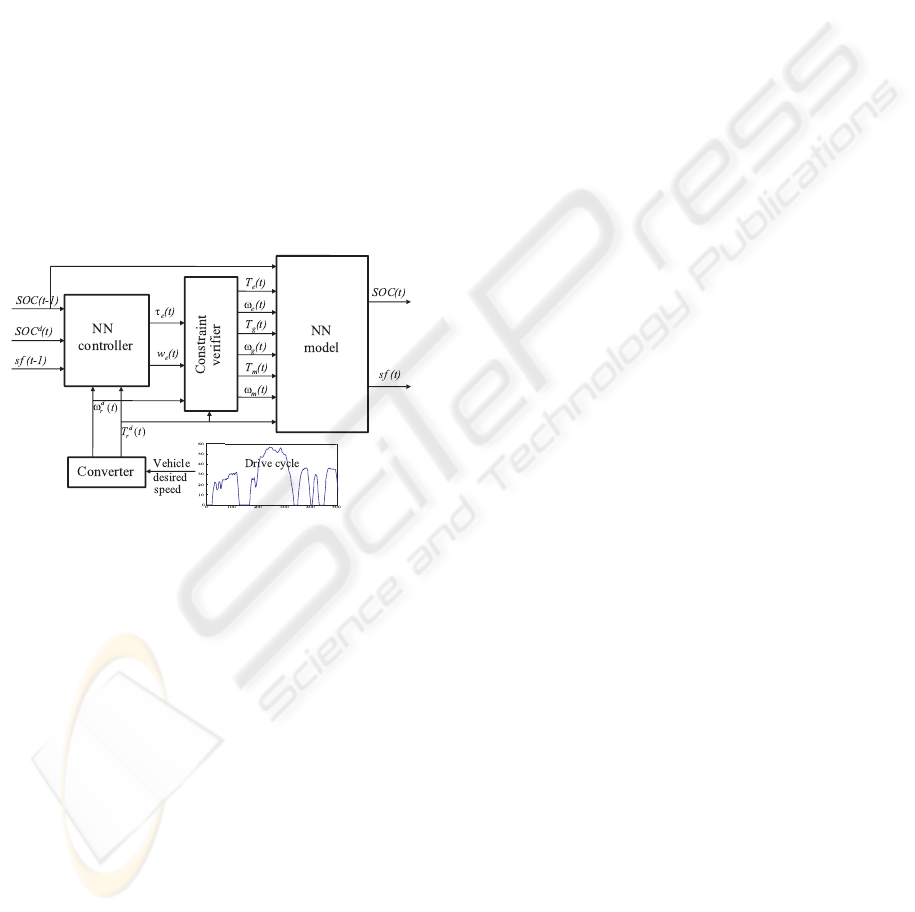
in the low-SOC region for long. Thus, λ
2
(t) = 10 if
SOC(t) ≥ SOC
d
, and λ
2
(t) = 50 if SOC(t) < SOC
d
.
Ultimately, we would also like to minimize emis-
sions of the harmful gases. In this study we attempt to
reduce emissions indirectly through reducing the fuel
consumption because they are often correlated.
Our RNN controller has 5-5R-2 architecture, i.e.,
five inputs, five recurrent nodes in the fully recur-
rent hidden layer, and two bipolar sigmoids as output
nodes. The RNN receives as inputs the required out-
put drive speed ω
d
r
and torque T
d
r
, the current engine
fuel rate s f, the current SOC and the desired SOC
SOC
d
(see Figure 2; the desired fuel rate is implicit,
and it is set to zero). The RNN produces two con-
trol signals in the range of ±1. The first output is the
engine torque τ
e
, and the second output is the engine
speed w
e
which become T
e
and ω
e
, respectively, after
passing through the constraint verifier.
Figure 2: Block diagram of the closed-loop system for train-
ing the NN controller. The converter determines the re-
quired values of speed ω
d
r
and torque T
d
r
at the ring gear
of the planetary mechanism to achieve the desired vehicle
speed profile. The constraint verifier makes sure not only
that the torques and speeds are within their specified physi-
cal limits but also that they are consistent with constraints of
the planetary gear mechanism. The trained NN model takes
care of the remaining complicated dynamics of the plant.
The feedback loop is closed via SOC and the fuel rate sf,
but the required ω
d
r
and T
d
r
are guaranteed to be achieved
through the appropriate design of the constraint verifier.
Our RNN controller is trained off-line using the
multi-stream EKF algorithm described in Section 2.
When training of our NN controller from Figure 2 is
finished, we can deploy it inside the high-fidelity sim-
ulator which approximates well behavior of the real
Prius and all its powertrain components. As expected,
we observed some differences between the neurocon-
troller performance in the closed loop with the NN
model and its performance in the high-fidelity simu-
lator because the NN model and the verifier only ap-
proximate the simulator’s behavior. Our results below
pertain to the simulator, rather than its NN approxi-
mation.
The basic idea of the current Prius HEV control
logic is discussed in (Hermance, 1999). When the
power demand is low and the battery SOC is suffi-
ciently high, the motor powers the vehicle. As the
power demand and vehicle speed increase, or the SOC
reduces below a threshold, the engine is started (the
generator may help the motor start the engine). The
engine power is split between propelling the vehi-
cle and charging the battery through the generator.
As the power demand continues to grow, the engine
might not be able to stay within its efficiency limits.
In those cases the motor can provide power assist by
driving the wheels to keep the engine efficiency rea-
sonably high, as long as the battery can supply the re-
quired power. During decelerations the motor is com-
manded to operate as a generator to recharge the bat-
tery, thereby implementing regenerative braking.
It is hard to make this rule-based strategy opti-
mal for such a complex powertrain. Significant aver-
aging over drive cycles with quite different behavior
compromising the best achievable performance is un-
avoidable. We believe that a strategy based on a data-
driven learning system should be able to beat the rule-
based strategy because of its ability to discern differ-
ences in driving patterns and take advantage of them
for improved performance.
We compare our RNN controller trained for ro-
bustness with the rule-based control strategy of the
Prius on 20 drive cycles including both standard
cycles (required by government agencies) and non-
standard cycles (e.g., random driving patterns). Our
RNN controller is better by 15% on average than the
rule-based controller in terms of fuel efficiency, and it
appears to be slightly better than the rule-based con-
troller in terms of its emissions on long drive cycles.
It also reduces variance of the SOC over the drive cy-
cle by at least 20%.
Figure 3 shows an example of our results. It is
a fragment of a long drive cycle (the total length is
12, 700 seconds). Our advantage appears to be in the
more efficient usage of the engine. The engine effi-
ciency is 32% vs. 29% for the rule-based controller.
We also achieved a big improvement in the genera-
tor efficiency: 77% vs. 32%, with other component
efficiencies remaining basically unchanged.
ICINCO 2007 - International Conference on Informatics in Control, Automation and Robotics
374