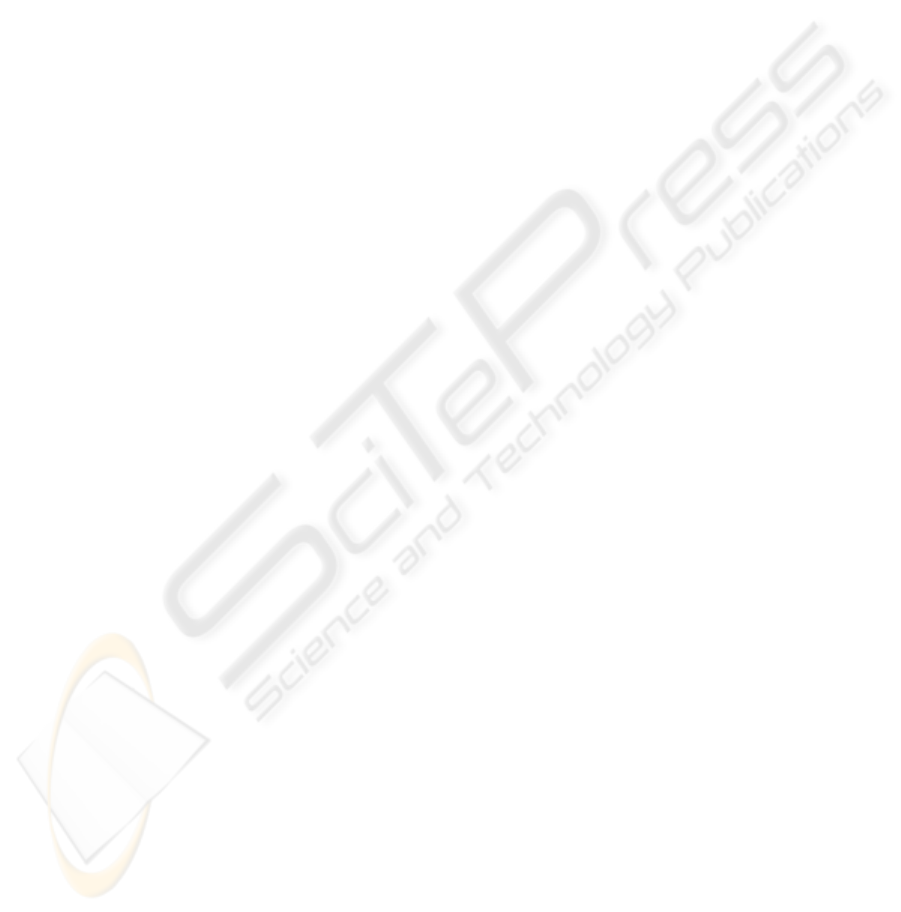
local information is reported. One of the important
aspects of the paper has been the simplicity, as well
as the easy and direct applicability of the
approaches. The proposed methodology has been
attained by using the on-robot local visual
information, acquired by a camera, and the
techniques of LMPC. The use of sensor fusion,
specially the odometer system information, is of a
great importance. The odometer system uses are not
just constrained to the control of the velocity of each
wheel. Thus, the absolute robot coordinates have
been used for planning a trajectory to the desired
global or local objectives. The local trajectory
planning has been done using the relative robot
coordinates, corresponding to the instant when the
frame was acquired. The available local visual data
provides a local map, where the feasible local
minimal goal is selected, considering obstacle
avoidance politics.
Nowadays, the research is focused to implement the
presented methods through developing flexible
software tools that should allow to test the vision
methods and to create locally readable virtual
obstacle maps. The use of virtual visual information
can be useful for testing the robot under synthetic
environments and simulating different camera
configurations. The MPC studies analyzing the
models derived from experiments as well as the
relative performance with respect to other control
laws should also be developed.
ACKNOWLEDGEMENTS
This work has been partially funded the Commission
of Science and Technology of Spain (CICYT)
through the coordinated research projects DPI-2005-
08668-C03, CTM-2004-04205-MAR and by the
government of Catalonia through SGR00296.
REFERENCES
Boyd, S., Vandenberghe, L., 2004. Convex Optimization,
Cambridge University Press.
Campbell, J., Sukthankar, R., Nourbakhsh, I., 2004.
Techniques for Evaluating Optical Flow in Extreme
Terrain, IROS.
Coué, C., Pradalier, C., Laugier, C., Fraichard, T.,
Bessière, P., 2006. Bayesian Occupancy Filtering for
Multitarget Tracking: An Automotive Application,
International Journal of Robotics Research.
Dennis, J.E., Shnabel, R.S., 1996. Numerical Methods for
Unconstrained Optimization and Nonlinear Equations,
Society for Industrial and Applied Mathematics.
DeSouza, G.N., Kak, A.C., 2002. Vision for Mobile Robot
Navigation: a survey, PAMI, 24, 237-267.
Elfes, A., 1989. Using occupancy grids for mobile robot
perception and navigation, IEEE Computer, 22, 46-
57.
Fox, D., Burgard, W., and Thun, S., 1997. The dynamic
window approach to collision avoidance, IEEE Robot.
Autom. Mag. 4, 23-33.
Gupta, G.S., Messom, C.H., Demidenko, S., 2005. Real-
time identification and predictive control of fast
mobile robots using global vision sensor, IEEE Trans.
On Instr. and Measurement, 54, 1.
Horn, B. K. P., 1998. Robot Vision, Ed. McGraw –Hill.
Küne, F., Lages, W., Da Silva, J., 2005. Point stabilization
of mobile robots with nonlinear model predictive
control, Proc. IEEE Int. Conf. On Mech. and Aut.,
1163-1168.
Lju, L., 1989. System Identification: Theory for the User,
ed., Prentice Hall.
Maciejowski, J.M., 2002. Predictive Control with
Constraints, Ed. Prentice Hall.
Nesterov, Y., Nemirovskii, A., 1994. Interior_Point
Polynomial Methods in Convex Programming, SIAM
Publications.
Norton, J. P., 1986. An Introduction to Identification, ed.,
Academic Press, New York.
Nourbakhsh, I. R., Andre, D., Tomasi, C., Genesereth, M.
R., 1997. Mobile Robot Obstacle Avoidance Via Depth
From Focus, Robotics and Aut. Systems, 22, 151-58.
Ögren, P., Leonard, N., 2005. A convergent dynamic
window approach to obstacle avoidance, IEEE T
Robotics, 21, 2.
Ortega, J. M., Rheinboldt, W.C., 2000. Iterative Solution
of Nonlinear Equations in Several Variables, Society
for Industrial and Applied Mathematics.
Rimon, E., and Koditschek, D., 1992. Exact robot
navigation using artificial potential functions, IEEE
Trans. Robot Autom., 8, 5, 501-518.
Schilling, R.J., 1990. Fundamental of Robotics, Prentice-
Hall.
Thrun, S., 2002. Robotic mapping: a survey, Exploring
Artificial Intelligence in the New Millennium, Morgan
Kaufmann, San Mateo, CA.
ICINCO 2007 - International Conference on Informatics in Control, Automation and Robotics
266