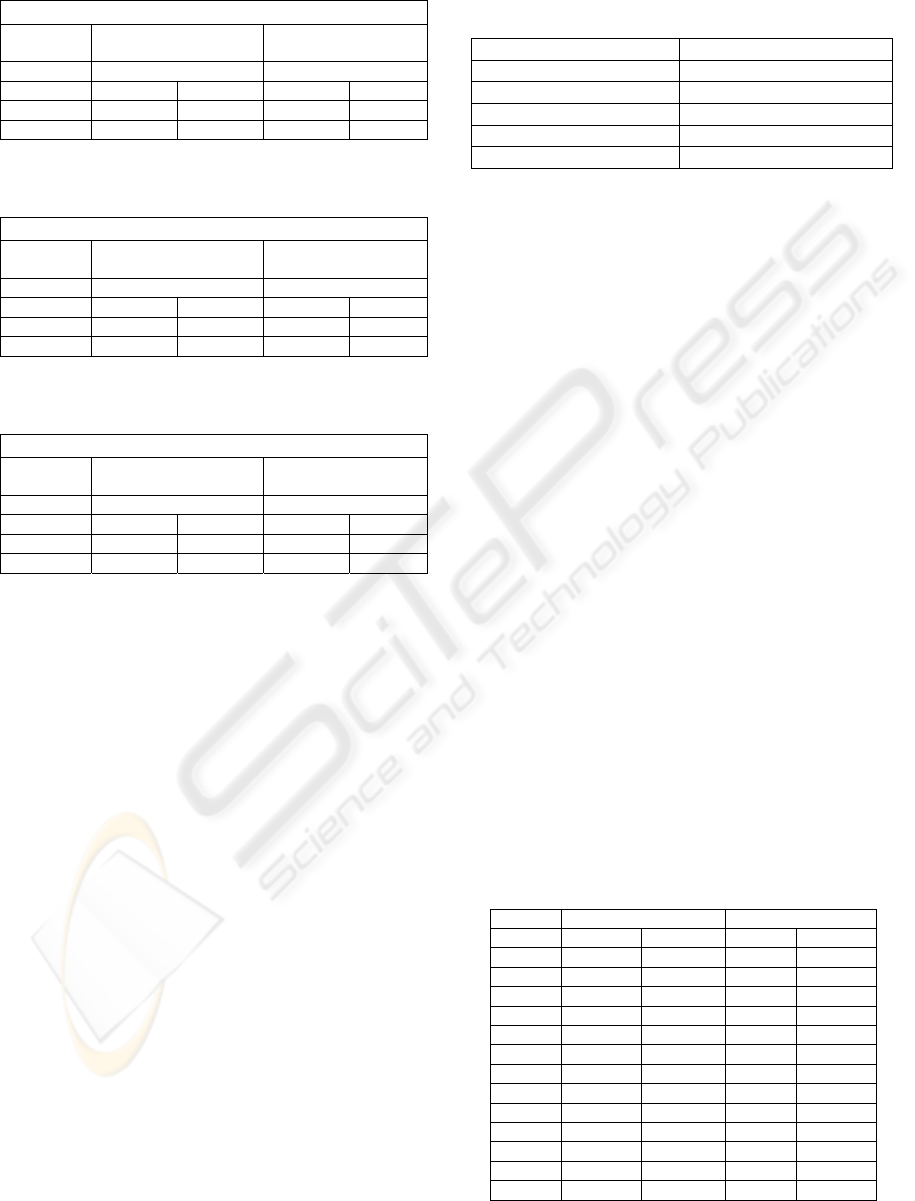
Table 9: Average results and standard deviation for
problem 3.
Problem 3
Average
Standard Deviation
Not Using Intensive. Using Intensive
V1 954,01 948,12 19,60 16,95
V2 903,63 901,17 14,50 17,84
V1,V2 879,90 870,10 15,05 20,73
Table 10: Average results and standard deviation for
problem 4.
Problem 4
Average
Standard Deviation
Not Using Intensive. Using Intensive
V1 1215,35 1210,79 12,79 10,70
V2 1124,93 1118,83 15,66 12,52
V1,V2 1087,72 1079,54 18,15 16,70
Table 11: Average results and standard deviation for
problem 5.
Problem 5
Average
Standard Deviation
Not Using Intensive. Using Intensive
V1 1569,11 1561,59 10,12 16,22
V2 1421,41 1393,56 34,40 12,75
V1,V2 1387,93 1377,82 26,64 16,37
From the results presented in tables 7 to 11 we can
see that the results generated by the movements
grouped are better than the results obtained using the
movements alone. The reason for this is that when
movements are used together the size of the
neighbourhood generated is bigger than the
neighbourhood generated by V1 or V2 alone. The
movements together also cause an increase of the
diversification of the solutions. And when the search
generates more results, it is doing a deeper search in
the space. Of course, as was shown, the
intensification strategy helps the search to produce
more qualified results.
When comparing the V1 and V2 movements, we can
see that V2 produce results more qualified. If we
analyse the policy behind the movement, we can say
that V2 is more flexible than V1. V1 needs that two
constraints are satisfied to generate one neighbour.
While in V2, just one demand capacity must be
verified (the capacity of the vehicle that serve the
route where the vertex are being allocated) in V1,
both routes must be verified to see if the vehicles
capacities aren’t exceeded.
Next table shows the best results obtained for each
problem. All the best results were obtained during
the search using movements V1 and V2 together and
with the intensification strategy.
Table 12: Best results obtained.
Problem Best Result
1 525,42
2 847,82
3 837,79
4 1061,07
5 1352,74
5.4 Comparisons
Aiming to evaluate the quality of the application
developed, some papers were selected from the
literature to compare the results. There were selected
some classical heuristic and some papers that also
used Tabu Search to solve the VRP. The paper
selected were: {WL} Willard (1989), {PF}Pureza
and França (1991), {OM1} Osman (1991), {OM2}
Osman (1993), {RG} Rego (1998), {GHL}
Gendreau, Hertz and Laporte (1994), {BO}
Barbarasoglu and Ozgur (1999), {XK} Xu and
Kelly (1996), {TV} Toth and Vigo (2003), {CW}
Clarke and Wright (1964), {GM} Gillet and Miller
(1974), {MJ} Mole and Jamenson (1976), {CMT}
Christofides, Mingozzi and Toth (1979).
Table 13 shows the comparison done with the results
from the papers. The results were obtained in
Barbarasolgu and Ozgur (Barbarasolgu and Ozgur,
1999) and in Gendreau, Hertz and Laporte
(Gendreau, Hertz and Laporte, 1994). In the first
columns the paper used is shown. Columns 2 and 4
present the best results from the paper and columns
3 and 5 shows the difference in percentage from the
results obtained in this paper to the paper compared.
This difference was called “gap”. The (+) indicate
that our result is that percentage more than the result
from the paper. The (-) indicate that our results is
that percentage minor than the result from the paper.
Table 13: Best Results and gap for problem 1 and 2.
Problem 1 Problem 2
Best %Gap Best %Gap
WL 588 11,91(-) 893 5,33(-)
RG 557,86 6,17(-) 847 0,10(+)
PF 536 2,10(-) 842 0,69(+)
OM1 524,61 0,15(+) 844 0,45(+)
OM2 524,61 0,15(+) 844 0,45(+)
GHL 524,61 0,15(+) 835,77 1,42(+)
BO 524,61 0,15(+) 836,71 1,31(+)
XK 524,61 0,15(+) 835,26 1,48(+)
TV 524,61 0,15(+) 838,60 1,09(+)
CW 578,56 10,11(-) 888,04 4,74(-)
GM 546 3,92(-) 865 2,03(-)
MJ 575 9,44(-) 910 7,33(-)
CMT 534 1,63(-) 871 2,73(-)
ICINCO 2007 - International Conference on Informatics in Control, Automation and Robotics
82