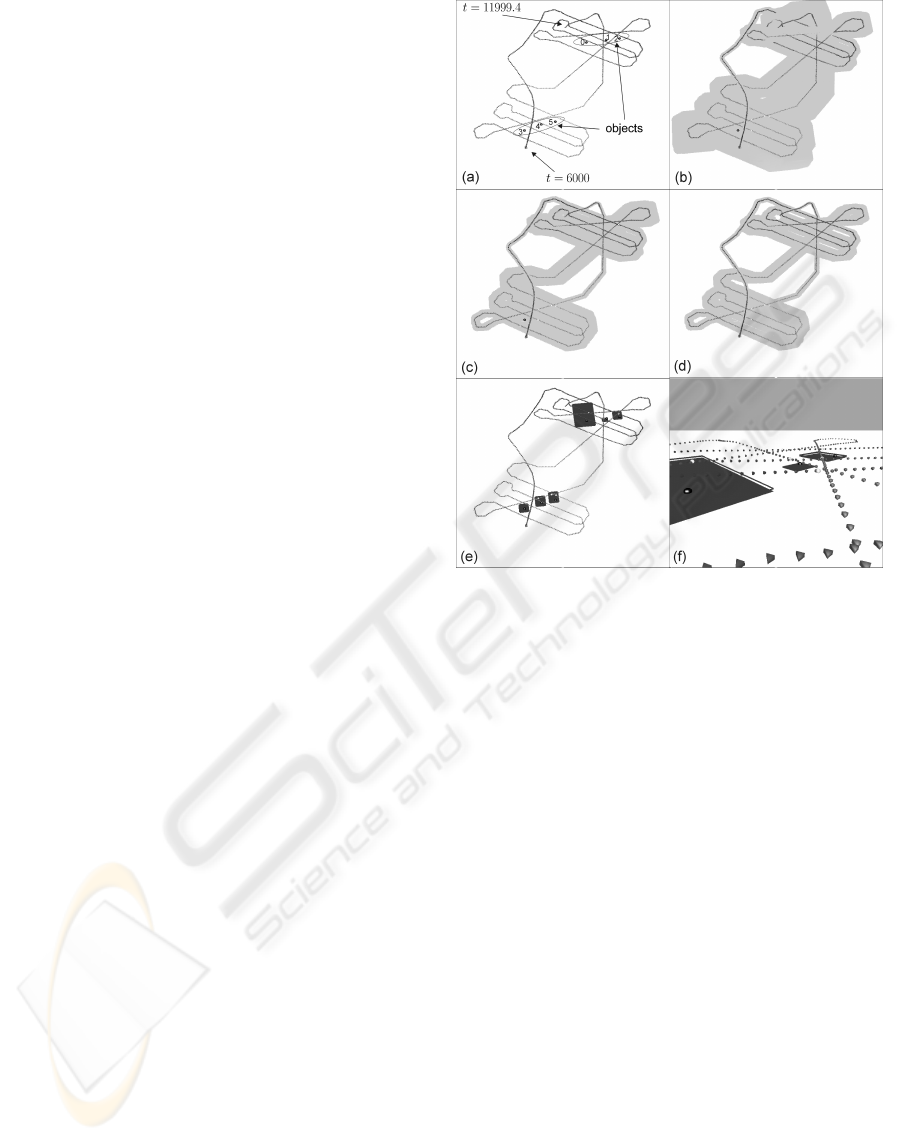
box, painted black. In Subfigure (f), a zooming per-
spective of the trajectory and the enveloping boxes
for the detected objects have been represented. The
computing time to get all these envelopes in less than
one minute with a Pentium III. About ten forward-
backward interval propagations have been performed
to get the steady box of the CSP.
In the case where the position of the marks is
approximately known, the SLAM problem translates
into a state estimation problem. The envelope for the
trajectory becomes very thin and a short computation
time is needed. The capabilities of interval propaga-
tion methods for state estimation in a bounded error
context have already been demonstrated in several ap-
plications (see e.g., (Gning, 2006), (Bouron, 2002),
(Baguenard, 2005), (Gning and Bonnifait, 2006),
(Jaulin et al., 2001)).
Figure 3 contains the reconstructed waterfall
(above) and one zoom (below). Each line corresponds
to one of the six seamarks (i = 0, .. . , 5) that have been
detected. The gray areas contains the set of all fea-
sible pairs (t,
k
p −m
i
k
), associated to the migration
hyperbola. The twelve small black disks correspond
to the detected marks. From each disk, we can get
the time t at which the mark has been detected (x-
axis), the number of the mark (corresponding to the
line), and the distance r
i
between the robot and the
mark ( y-axis). Black areas correspond to all feasible
(t,r
i
). Some of these areas are tiny and are covered
by a black disk. Some are larger and do not contain
any black disk. In such a case, an existing mark may
have been missed by the operator during the scrolling
of the waterfall. As a consequence, with the help of
Figure 3, the operator could scan again the waterfall
and find undetected marks much more efficiently. If
the operator (which could be a human or a program) is
able to detect at least one more mark, then, the prop-
agation algorithm could be thrown once more to get a
thinner envelope for the trajectory, thinner black areas
in the reconstructed waterfall and thus a higher prob-
ability to detect new marks on the waterfall, . . . The
operator can thus be seen as a contractor ((Jaulin et
al., 2001)) inside a constraint propagation process.
5 CONCLUSION
In this paper, we have shown that interval con-
straints propagation could be applied to solve effi-
ciently SLAM problems. The approach has been
demonstrated on an experiment made with an actual
underwater robot (the Redermor). The experiment
lasted two hours and involved thousands of data. If
all assumptions on the bounds of the sensors, on the
Figure 2: Results obtained by the interval constraint propa-
gation.
flat bottom, on the model of the robot, . . . are satis-
fied, then their exists always at least one solution of
our problem: that corresponding to the actual trajec-
tory of the robot.
When outliers occur during the experiment, our
approach is not reliable anymore and one should
take care about any false interpretation of the results.
Consider now three different situation that should be
known by any user of our approach for SLAM.
Situation 1 . The solution set is empty and an
empty set is returned by the propagation procedure.
Our approach detects that their exists at least one out-
lier but it is not able to return any estimation of the
trajectory and the positions of the marks. It is also
not able to detect which sensor is responsible for the
failure.
Situation 2. The solution set is empty but
nonempty thin intervals for the variables are re-
turned by the propagation. Our approach is not ef-
ficient enough to detect that outliers exist and we can
wrongly interpret that an accurate and guaranteed es-
timation of the trajectory of the robot has been done.
Other more efficient algorithms could be able to prove
that no solution exists which would lead us to the sit-
uation 1.
A SET APPROACH TO THE SIMULTANEOUS LOCALIZATION AND MAP BUILDING - Application to Underwater
Robots
67