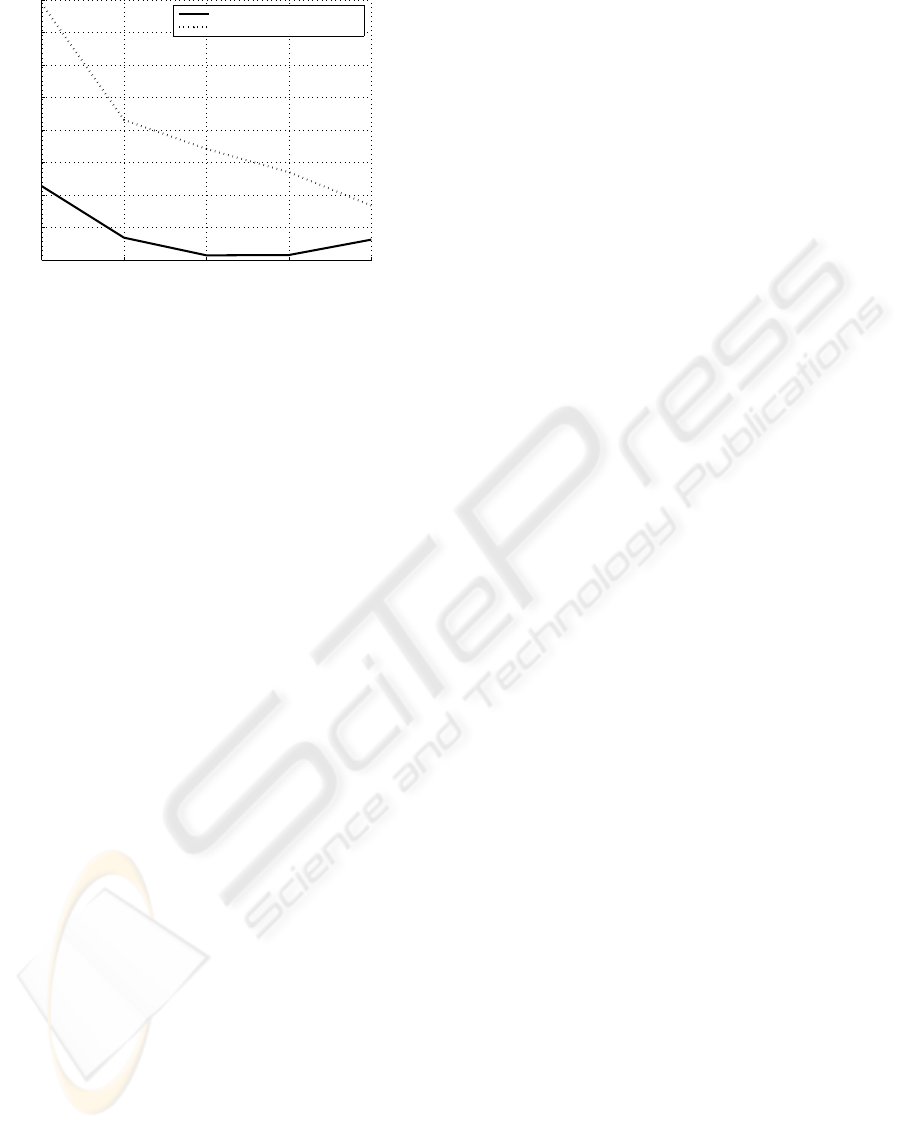
19 33 44 55 Inf
30
35
40
45
50
55
60
65
70
SNR in dB of signal to reverse
MSE
2
*epochs
Comparison of the training efforts for differents SNR values
Black−box neural network
Semi−physical neural network
Figure 10: Curves of learning effort on the test set.
that, it is the black-box which is slightly more effec-
tive. On figure 10, the tendencies are the same for the
black-box model. The gray-box model is penalized
by the constraint imposed by the neural framework
and the more reduced number of connections. Within
the framework of a traditional second order ODE, the
semi-physical model seems to require a greater effort
with the test set than the black-box model. Indeed,
within the framework of this example, the number of
coefficients remaining free for the gray-box model be-
ing relatively low, it leads to a loss of the generaliza-
tion capacity compared to the black-box model. How-
ever, this supremacy is quite relative since only 76%
of the tests carried out have been conclusive for the
black-box model and 100% for the gray-box model.
7 CONCLUSION
We have examined the performances of an inverse dy-
namic model by fusion of statistical learning and de-
terministic modeling. For the study, our choices have
gone toward the design of an inverse semi-physical
model using a looped neural network. We have com-
pared the latter with an inverse fully connected neu-
ral network. Experimental results on a second order
system have shown that the inverse gray-box neural
model is more parsimonious and presents better per-
formances in term of learning effort than the inverse
black-box neural model, because of knowledge in-
duced by the deterministic model. The performances
in term of inverse modeling precisions are visible
since the input restoration errors are weak. The neu-
ral training plays the part of statistical regressor and of
regularization operator. Finally, a higher order model
increases the number of neurons and then improves
the robust effect to the noise of the gay-box model.
REFERENCES
Cherkassky, V., Krasnopolsky, V. M., Solomatine, D., and
Valdes, J. (2006). Computational intelligence in earth
sciences and environmental applications: Issues and
challenges. Neural Networks, 19, issue 2:113–121.
Dreyfus, G., Martinez, J. M., Samuelides, M., Gordon,
M. B., Badran, F., Thiria, S., and H
´
erault, L. (2004).
R
´
eseaux de Neurones: M
´
ethodologies et Applications.
Eyrolles, 2
`
eme
´
edition, Paris.
Groetsch, C. W. (1993). Inverse Problems in the Mathemat-
ical Sciences. Vieweg Sohn, Wiesbaden.
Hornik, K., Stinchcombe, M., and White, H. (1989). Multi-
layer feedforward networks are universal approxima-
tors. Neural Networks, 2:359–366.
Idier, J. (2001). Approche Bayesienne pour les Probl
`
emes
Inverses. Trait
´
e IC2, S
´
erie Traitement du Signal et de
l’Image, Herm
`
es, Paris.
Krasnopolsky, V. M. and Fox-Rabinovitz, S. F. (2006).
Complex hybrid models combining deterministic and
machine learning components for numerical climate
modeling and weather prediction. Neural Networks,
19:122–134.
Krasnopolsky, V. M. and Schillerb, H. (2003). Some neural
network applications in environmental sciences. part i:
Forward and inverse problems in geophysical remote
measurements. Neural Networks, 16:321–334.
Ljung, L. (1999). System Identification, Theory for the
User. Prentice Hall, N. J.
Mohammad-Djafari, A., Giovannelli, J. F., Demoment, G.,
and Idier, J. (2002). Regularization, maximum en-
tropy and probabilistic methods in mass spectrometry
data processing problems. Int. Journal of Mass Spec-
trometry, 215, issue 1:175–193.
Nguyen, D. and Widrow, B. (1990). Improving the learning
speed of 2-layer neural networks by choosing initial
values of the adaptive weigths. Proceedings of the
International Joint Conference on Neural Networks,
3:21–26.
Oussar, Y. and Dreyfus, G. (2001). How to be a gray box
: Dynamic semi-physical modeling. Neurocomputing,
14:1161–1172.
Sontag, E. D. (1996). Recurrent neural networks : Some
systems-theoretic aspects. Technical Report NB, Dept
of mathematics, Rutgers University, U.S.A.
Thikhonov, A. N. and Arsenin, V. Y. (1977). Solutions of ill
Posed Problems. John Wiley, New York.
INVERSION OF A SEMI-PHYSICAL ODE MODEL
371