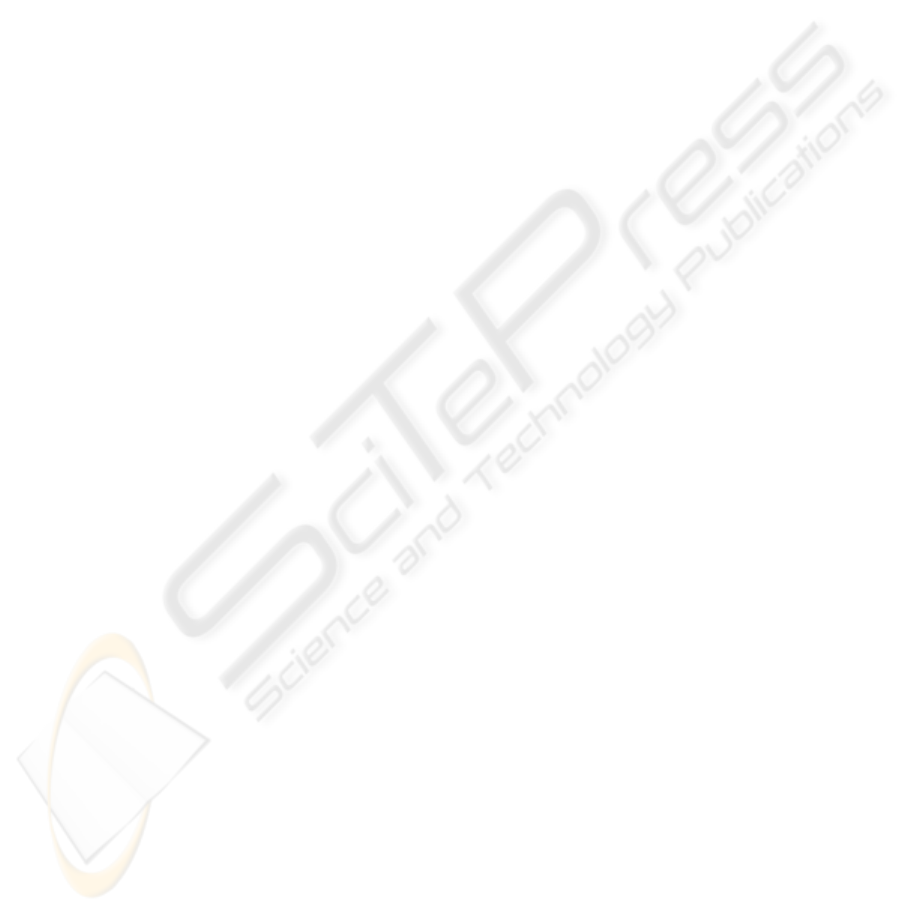
notation editor, keyboard as input tool, sound com-
municator.
Blind people do not use pointing devices. In con-
sequence, all input functions usually performed with
mouse must be mapped to computer keyboard. Mas-
sive communication with usage of keyboard requires
careful design of interface mapping to keyboard, c.f.
(Moniuszko, 2006).
Blind user usually do not know printed music no-
tation. Their perception of music notation is based on
Braille music notation format presented at Braille dis-
play or punched sheet of paper, c.f. (Krolick, 1998).
In such circumstances music information editing must
be done on Braille music notation format. Since typi-
cal Braille display is only used as output device, such
editing is usually done with keyboard as input de-
vice. In Braille Score Braille representation of music
is converted online to internal representation and dis-
played in the form of music notation in usual form.
This transparency will allow for controlling correct-
ness and consistency of Braille representation, c.f.
(Moniuszko, 2006).
Sound information is of height importance for
blind user of computer program. Wide spectrum of
visual information displayed on a screen for user with
good eyesight could be replaced by sound informa-
tion. Braille Score provides sound information of two
types. The first type of sound information collabo-
rates with screen readers, computer programs dedi-
cated to blind people. Screen readers could read con-
tents of a display and communicate it to user in the
form of synthesized speech. This type of communica-
tion is supported by contemporary programming en-
vironments. For this purpose Braille Score uses tools
provided by Microsoft .NET programming environ-
ment. The second type of sound information is based
on own Braille Score tools. Braille Score has embed-
ded mechanism of sound announcements based on its
own library of recorded utterances.
5 CONCLUSIONS
The aim of this paper is a discussion on involvement
of computational intelligence methods in implemen-
tation of user friendly computer programs focused on
disabled people. In te paper we describe a concept
of Braille Score the specialized computer program
which should help blind people to deal with music
and music notation. The use of computational intel-
ligence tolls can improve the program part devoted
to recognition and processing of music notation. The
first results with Braille Score show its to be a practi-
cal and useful tool.
REFERENCES
Bainbridge, D. and Bell, T. (2001). The challenge of optical
music recognition. Computers and the Humanities,
35:95–121.
Carter, N. P. and Bacon, R. A. (1992). Automatic Recogni-
tion of Printed Music, pages 456–465. in: Structured
Document Analysis, Analysis, H.S.Baird, H.Bunke,
K.Yamamoto (Eds). Springer Verlag.
Dannenberg, R. and Bell, T. (1993). Music representa-
tion issues, techniques, and systems. Computer Music
Journal, 17(3):20–30.
Fujinaga, I. (2001). Adaptive optical music recognition. In
16th Inter. Congress of the Inter. Musicological Soci-
ety, Oxford.
Goolsby, T. W. (1994a). Eye movement in music reading:
Effects of reading ability, notational complexity, and
encounters. Music Perception, 12(1):77–96.
Goolsby, T. W. (1994b). Profiles of processing: Eye
movements during sightreading. Music Perception,
12(1):97–123.
Homenda, W. (1996). Automatic recognition of printed mu-
sic and its conversion into playable music data. Con-
trol and Cybernetics, 25(2):353–367.
Homenda, W. (2002). Granular computing as an abstraction
of data aggregation - a view on optical music recogni-
tion. Archives of Control Sciences, 12(4):433–455.
Homenda, W. (2005). Optical music recognition: the case
study of pattern recognition. In in: Computer Recog-
nition Systems, Kurzynski et al (Eds.), pages 835–842.
Springer Verlag.
Homenda, W. and Luckner, M. (2004). Automatic recog-
nition of music notation using neural networks. In
in: Proc. of the International Conference On Artifi-
cial Intelligence and Systems, Divnomorskoye, Rus-
sia, September 3-10, pages 74–80, Moscow. Phys-
mathlit.
Homenda, W. and Mossakowski, K. (2004). Music symbol
recognition: Neural networks vs. statistical methods.
In in: EUROFUSE Workshop On Data And Knowl-
edge Engineering, Warsaw, Poland, September 22 -
25, pages 265–271, Warsaw. Physmathlit.
Krolick, B. (1998). How to Read Braille Music. Opus Tech-
nologies.
McPherson, J. R. (2002). Introducing feedback into an op-
tical music recognition system. In in: Third Internat.
Conf. on Music Information Retrieval, Paris, France.
Moniuszko, T. (2006). Design and implementation of mu-
sic processing computer program for blind people (in
polish). Master’s thesis, Warsaw University of Tech-
nology.
Pedrycz, W. (2001). Granular computing: An introduc-
tion. In in: Joint 9th IFSA World Congress and 20th
NAFIPS International Conference, Vancouver, pages
1349–1354.
Taube, H. (1993). Stella: Persistent score representation
and score editing in common music. Computer Music
Journal, 17(3):38–50.
BREAKING ACCESSIBILITY BARRIERS - Computational Intelligence in Music Processing for Blind People
39