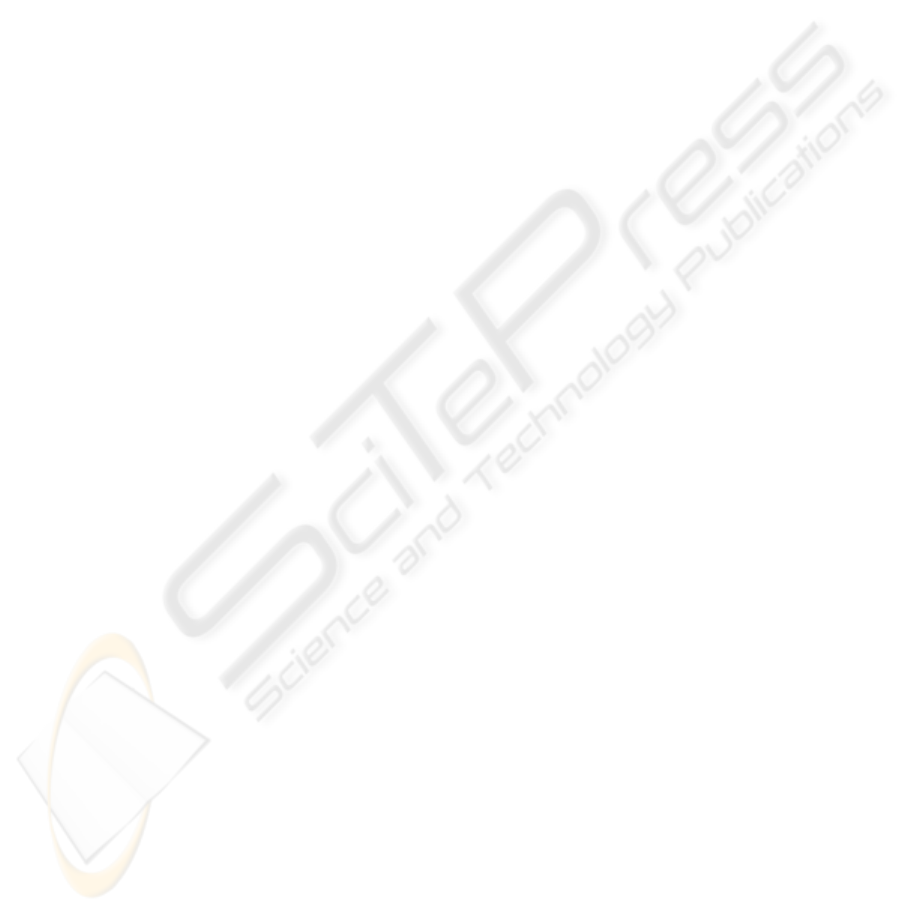
and it determines what transformations
i
C
W
and
i
C
are more suitable. Afterwards, a robot PA-10
from Mitsubishi, with 7 degrees of freedom, moves
the camera mounted at its end to more suitable
computed pose.
R
W
t
6 CONCLUSIONS
The presented work provides an input to an object
recognition process. Thus, a method based on
extraction of characteristics in image, which is
based on the evaluation of the distances among these
characteristics, is used to determine when an
occlusion can appear. In addition, the method
evaluates the camera pose of a virtual way from the
back-projections of the characteristics detected in a
real image. The back-projections determine how the
characteristics are projected in virtual images
defined by different camera poses without the
necessity of camera is really moved. The
experimental results have shown that the proposed
estimation can successfully be used to determine the
camera pose that is not too sensitive to occlusions.
However, the approach proposed does not provide
an optimal solution. This could be solved by
applying visual control techniques which are
currently under investigation.
Our future work will extend this approach to
incorporate visual servoing in camera pose, allowing
for a robust positioning camera. A visual servoing
system with a configuration ‘eye-in-hand’ can be
used to evaluate each camera pose (Pomares, 2006).
Thus, the errors can be decreased and the trajectory
can be changed during the movement. In addition,
the information provided from a model CAD of the
objects (see Figure 6b) can be used to verify camera
poses in which it is located.
ACKNOWLEDGEMENTS
This work was funded by the Spanish MCYT project
“Diseño, implementación y experimentación de
escenarios de manipulación inteligentes para
aplicaciones de ensamblado y desensamblado
automático (DPI2005-
06222)”.
REFERENCES
Boshra, M., Ismail, M.A., 2000. Recognition of occluded
polyhedra from range images. Pattern Recognition.
Vol. 3, No. 8, 1351-1367.
Chan, C.J., Chen S.Y., 2002. Recognition Partially
Occluded Objects Using Markov Model. Int. J.
Pattern Recognition and Artificial Intelligence. Vol.
16, No. 2, 161-191.
El-Sonbaty, Y., Ismael, M.A, 2003. Matching Occluded
Objects Invariant to Rotations, Translations,
Reflections, and Scale Changes. Lecture Notes in
Computer Science. Vol. 2749, 836-843.
Fiala, M., 2005. Structure From Motion Using SIFT
Features and PH Transform with Panoramic Imagery.
Second Canadian Conference on Computer and Robot
Vision. Victoria, BC, Canada.
Gil, P., Torres, F., Ortiz, F.G., Reinoso, O., 2006.
Detection of partial occlusions of assembled
components to simplify the disassembly tasks.
International Journal of Advanced Manufacturing
Technology. No. 30, 530-539.
Gruen, A., Huang, T.S., 2001. Springer Series in
Information Sciences. Calibration and Orientation of
Cameras in Computer Vision. Springer-Verlag Berling
Heidelberg New York.
Hartley, R., Zisserman, A., 2000. Multiple View Geometry
in Computer Vision. Cambridge University Press.
Ma, Y., Soato S., Kosecka J., Shankar S., 2004. An
Invitation to 3-D Vision from Images to Geometric
Models. Springer-Verlag, New York Berlin
Heidelberg.
Ohba, K., Sato, Y., Ikeuchi, K., 2000. Appearance-based
visual learning and object recognition wirh
illumination invariance. Machine Vision and
Appplications 12, 189-196.
Ohayon, S., Rivlin, E., 2006. Robust 3D Head Tracking
Using Camera Pose Estimation. 18
th
International
Conference on Pattern Recognition. Hong Kong.
Park, B.G., Lee K.Y., Lee S.U., Lee J.H., 2003.
Recognition of partially occluded objects using
probabilistic ARG (attributed relational graph)-based
matching. Computer Vision and Image Understanding
90, 217-241.
Pomares, J., Gil, P., Garcia, G.J., Torres, F., 2006. Visual-
force control and structured Light fusion improve
object discontinuities recognition. 11
th
IEEE
International Conference on Emerging Technologies
and Factory Automation. Praga.
Silva, C., Victor, J.S., 2001. Motion from Occlusions.
Robotics and Autonomous Systems 35, 153-162.
Ying, Z., Castañon, D., 2000. Partially Occluded Object
Recognition Using Statical Models. Int. J. Computer
Vision. Vol. 49, No. 1, 57-78.
Wunsch, P., Winkler S., Hirzinger, G., 1997. Real-Time
Pose Estimation of 3-D Objects from Camera Images
Using Neural Networks. IEEE International
Conference on Robotics and Automation.Albuquerque,
New Mexico, USA.
Zhang, Z., 2000. A flexible new technique for camera
calibration. IEEE Transactions on Pattern Analysis
and Machine Intelligence, Vol 22. No. 11, 1330-1334.
ESTIMATION OF CAMERA 3D-POSITION TO MINIMIZE OCCLUSIONS
317