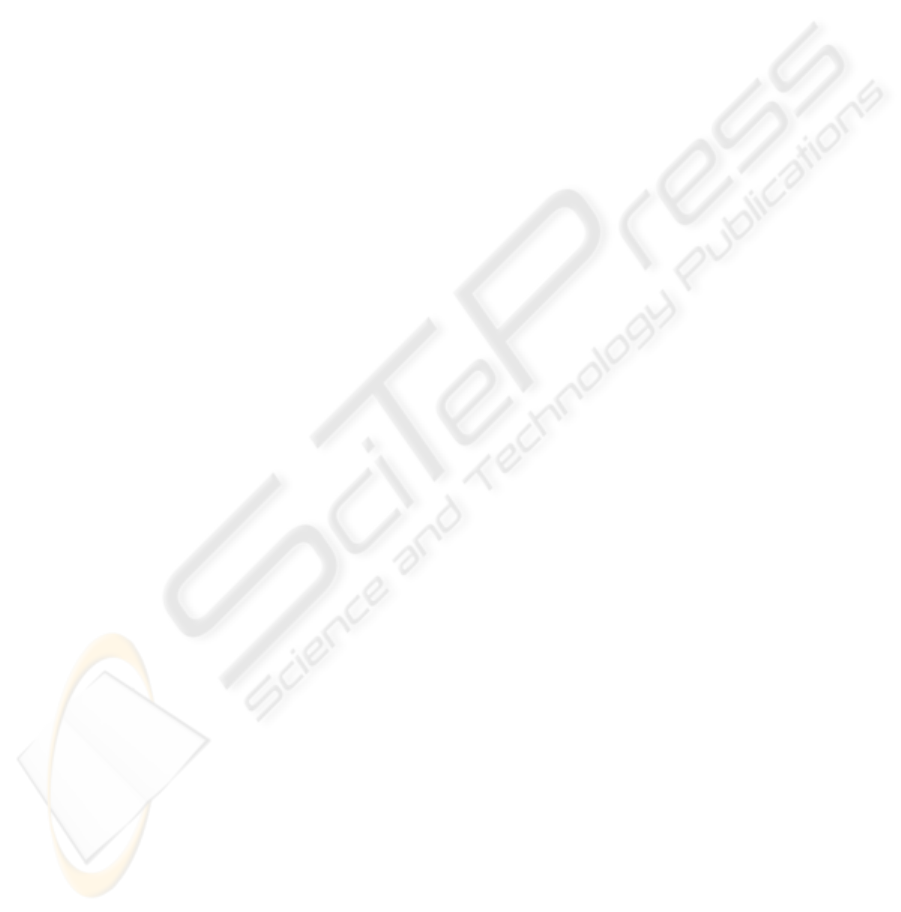
Fig.8 denotes the performance curves of PN
sequence estimation. It shows the time taken for the
NN to perfectly estimate the PN sequence for
lengths of
N=100bit and N=1000bit at T
x
/T
0
=0.4.
Under the same condition, when the longer the PN
sequence is, the better the performance is.
6 CONCLUSIONS
A modified Sanger’s GHA NN technique for blind
estimation and adaptive tracking of PN sequence of
DS signals is developed and demonstrated. The
technique, referred to here as the modified Sanger’s
GHA NN algorithm, exploits the subspace analysis
based on K-L transformation of the DS signal to
blindly estimate and adaptively track the spreading
code and can further despread the underlying
message sequence, without knowledge of the content
of the PN code or message sequences. The technique
is applicable to arbitrary spreading codes and
message sequences, and can operate in environments
containing arbitrary levels of additive white
Gaussian noise in theory.
The technique is demonstrated for the length of
PN code
N=100bit and 1000bit DS-SS signal
received in
-20 dB to 0 dB of additive white
Gaussian noise. It is shown that the technique can
blindly estimate and adaptively track the PN
sequence in the presence of strong additive white
Gaussian noise. In (Simic et al., 2005) Simic used
the method of eigen-analysis to achieve –5dB of the
SNR threshold, moreover, in (Zhan et al., 2005)
Zhan use the method of matrix to achieve –12dB
SNR
threshold, but we can realize threshold of
dBSNR 0.20−=
easily here, hence the performance
of the methods in this paper is more better. The
convergence time of the algorithm for PN sequence
perfect estimation is also shown to be competitive
with conventional despreading techniques (which
require knowledge of the spreading code) such as
delay-lock loops.
These results show that modified Sanger’s
GHA NN technique can provide a promising
alternative to existing despreading algorithms. The
algorithm can be applicable to signals with short
code lengths, such as commercial communication
signals. The algorithm can be also applicable to
signals with longer code lengths, such as military
communication signals. It can be further used in
management and scout of DS communications.
ACKNOWLEDGEMENTS
This work is supported by the National Natural
Science Foundation of China (No.60602057), the
Natural Science Foundation of Chongqing
University of Posts and Telecommunications
(CQUPT) (No.A2006-04
, No.A2006-86), the
Natural Science Foundation of Chongqing
Municipal Education Commission (No.KJ060509),
and the Natural Science Foundation of Chongqing
Science and Technology Commission (No.
CSTC2006BB2373).
REFERENCES
M. K. Simon, J. K. Omura, R. A. Scholtz, and B. K.
Levitt,
Spread Spectrum Communications Handbook.
New York: McGraw-Hill, 1994.
C. A. French and W. A. Gardner, “Spread spectrum
despreading without the code,” IEEE Trans.
Cornmun.,
vol. COM-34, pp. 404-408, Apr. 1986.
Tianqi Zhang, Xiaokang Lin and Zhengzhong Zhou,
“Blind Estimation of the PN Sequence in Lower SNR
DS/SS Signals , ”
IEICE Transaction On
Communications
, Vol.E88-B, No.7, JULY, 2005, pp.
3087-3089.
Simic, S. and Zejak, A., “Blind Estimation of the Code
Sequence in Spread Spectrum Radar,”
the 7th
International Conference on Telecommunications in
Modern Satellite, Cable and Broadcasting Services,
IEEE-TELSIKS, 2005
. Vol.2, 28-30 Sept. 2005, pp:
485 – 490.
Zhan, Y., Cao Z., and Lu J., “Spread-spectrum sequence
estimation for DSSS signal in non-cooperative
communication systems,”
IEE Proc.-Commun,
Vol.152, No.4, 2005, pp.476-480.
T.D. Sanger, “Optimal unsupervised learning in a single-
layer linear feedforward neural networks,”
Neural
Networks
, vol.3, pp.459-473, 1989.
S. Haykin,
Neural Networks—A Comprehensive
Foundation
. Prentice Hall PTR, Upper Saddle River,
NJ, USA, 1999.
T.W. Anderson. Asymptotic theory for principal
component analysis.
Ann. Math. Statist., 1963, 35:
1296-1303.
ICINCO 2007 - International Conference on Informatics in Control, Automation and Robotics
384