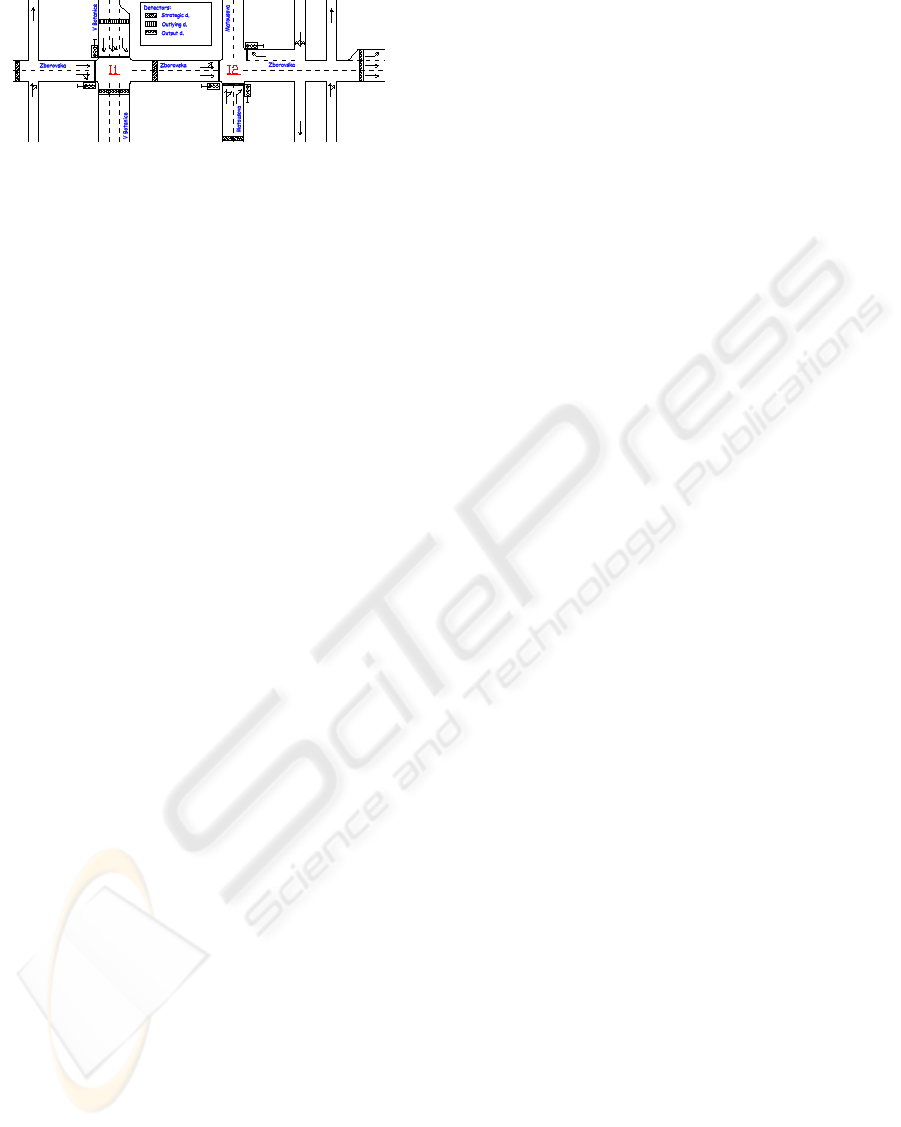
Figure 5: The micro-region: two four-arm controlled and
three uncontrolled intersections.
Prague including two four-arm controlled intersec-
tions and three uncontrolled ones. The arms are one-
way and they consist of several lanes, see Figure 5.
Two input arms are equipped by strategic detectors
and one input arm by outlying detector. Output detec-
tors are in two arms.
For queue estimation, the state space model (3)
and (4) without any approximation was used. The ex-
tended state is dim( ˜x
t
) = 50.
The input and output intensities, occupancies and
green times were measured on the real traffic net dur-
ing several months with sample period 90 sec. The
“true” queue length was again determined with sim-
ulation software AIMSUN. Comparing of the esti-
mated states and simulated ones shows that the esti-
mation depends on the type of input detector. In lanes,
which are equipped by the strategic detector, was usu-
ally MSE ≈ 17, the error is about 10% with respect
to the maximal queues. On the other hand, in lanes,
equipped by the outlying detectors only, the good re-
sults was only in cases where the queue did not exceed
the outlying detector. This is residual queue and for
evaluation of traffic situation is not interesting.
The experiments show that the nonlinear estima-
tion methods are a sufficient tool for estimation of the
queue lengths, even in a real network. The dimension
of the state, which is extended due to the estimation of
parameters or intensities, increases the computation
time, however, the computational demands remains
still feasible (namely the DD1 and model variant (B)).
6 CONCLUSION
The problem of the queue length estimation, which
is hardly measurable quantity, was considered in this
paper. For the queue estimation, the mathematical
model was presented, which describes the micro-
region including its physical properties and taking
into account behaviour of drivers. The disadvan-
tage of the model was highlighted and two possi-
ble solutions of that were proposed. The theoreti-
cal results were illustrated by the numerical examples
based on the synthetic or real data. It was shown that
the Kalman Filter is suitable for situations where all
quantities are measured. In other cases, it is advanta-
geous to use a nonlinear filters for concurrent estima-
tion of the state and parameters or possibly other un-
measurable quantities together with improved model.
ACKNOWLEDGEMENTS
The work was supported by the Ministry of Educa-
tion, Youth and Sports of the Czech Republic, project
No. 1M0572 and by the Ministry of Transport of the
Czech Republic, project No. 1F43A/003/120.
REFERENCES
Anderson, B. D. O. and Moore, S. B. (1979). Optimal Fil-
tering. Englewood Cliffs, New Jersey: Prentice Hall
Ins.
Dun
´
ık, J.,
ˇ
Simandl, M., Straka, O., and Kr
´
al, L. (2005).
Performance analysis of derivative-free filters. In
Proceedings of the 44th IEEE Conference on Deci-
sion and Control, and European Control Conference
ECC’05, pages 1941–1946, Seville, Spain. ISBN: 0-
7803-9568-9, ISSN: 0191-2216.
Homolov
´
a, J. and Nagy, I. (2005). Traffic model of a
microregion. In Preprints of the 16th IFAC World
Congress, pages 1–6, Prague, Czech Republic.
Julier, S. J., Uhlmann, J. K., and Durrant-White, H. F.
(2000). A new method for the nonlinear transforma-
tion of means and covariances in filters and estimators.
IEEE Transactions On AC, 45(3):477–482.
Kratochv
´
ılov
´
a, J. and Nagy, I. (2004). Traffic control of mi-
croregion. In Andr
´
ysek, J., K
´
arn
´
y, M., and Krac
´
ık,
J., editors, CMP’04: Multiple Participant Decision
Making, Theory, algorithms, software and app., pages
161–171, Adelaide. Advanced Knowledge Int.
Ljung, L. (1999). System identification: theory for the user.
UpperSaddle River, NJ: Prentice-Hall.
Nørgaard, M., Poulsen, N. K., and Ravn, O. (2000). New
developments in state estimation for nonlinear sys-
tems. Automatica, 36(11):1627–1638.
S
¨
oderstr
¨
om, T. and Stoica, P. (2002). Instrumental variable
methods for system identification. Circuits, Systems,
and Signal Processing, 21(1):1–9.
Sorenson, H. W. (1974). On the development of practical
nonlinear filters. Inf. Sci., 7:230–270.
Viberg, M. (2002). Subspace-based state-space system
identification. Circuits, Systems, and Signal Process-
ing, 21(1):23–37.
ˇ
Simandl, M. and Dun
´
ık, J. (2007). Multi-step prediction
and its application for estimation of state and mea-
surement noise covariance matrices. Technical report.
University of West Bohemia in Pilsen, Department of
Cybernetics.
ICINCO 2007 - International Conference on Informatics in Control, Automation and Robotics
228