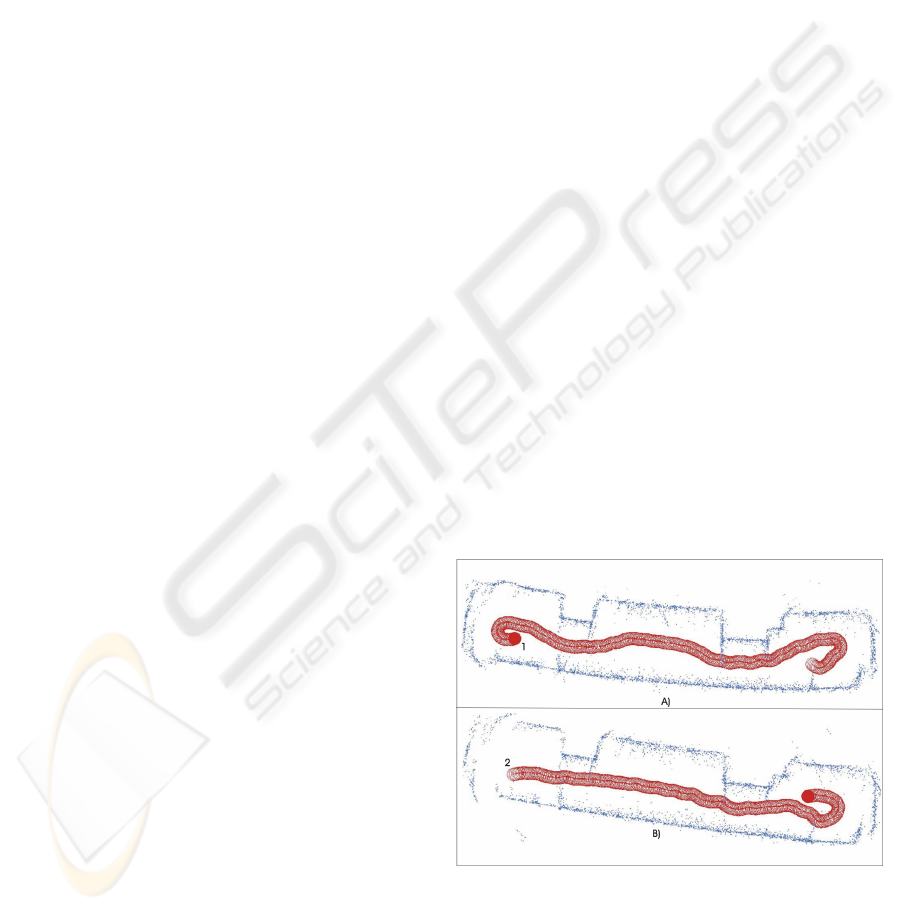
to carry out at each state, s: π(s). The population
of chromosomes is evaluated according to an objec-
tive function called fitness function, which reflects for
how long a chromosome is able to properly control
the movement of the robot – figure 1.b –. Once a
population of chromosomes has been evaluated, the
sequence of states, actions, and rewards the robot re-
ceived under the control of the best chromosome, is
replicated off-line several times to speed up the con-
vergence of the Q-values.
2.3 Generation of a New Population of
Solutions (Chromosomes)
The population of chromosomes has to be evolved
according to the fitness values. In order to do this,
certain genetic operators like mutation –which carries
out random changes in the chromosomes–, or chro-
mosome crossover –which combines chromosomes to
raise new solutions– have to be applied. We use the
Q-values to bias the genetic operators and thus reduce
the number of chromosomes which are required to
find a solution. Given a particular chromosome π, the
probability that mutation changes the action that this
chromosome suggests for a particular state s: π(s),
depends on how many actions look better or worse
than π(s) according to the Q-values.
On the other hand, one of the chromosomes
should always be the greedy policy because it brings
together all that has been already learnt by the robot,
and it represents the best chance to have a fast conver-
gence towards the desired solution.
Finally, when the robot is looking for a new start-
ing position and the greedy policy is being used to
control it, if the robot moves properly during M steps
before it receives negative reinforcements, only the
states involved in the last K robot’s movements are
susceptible of being changed through the GA, while
the states involved in the initial M-K actions are la-
belled as learnt, so that neither chromosome selection
nor chromosome crossover can alter them.
The population of chromosomes is resized after
its evaluation according to how close the GA is to the
desired solution.
2.4 Dynamic Representation of States
We use the properties of the regular Markov chains
(Bertsekas and Tsitsiklis, 1996) to reduce the num-
ber of states which are considered during the learn-
ing process. The transition matrix and what is called
steady vector are estimated, so that only those states
with a non-cero entry in the steady vector are con-
sidered in the learning procedure. The steady vector
contains the probability of finding the robot in each
possible state in the long-term.
3 EXPERIMENTAL RESULTS
We applied our approach to teach a mobile robot two
common tasks: “wall following” and “door traver-
sal”. We have used a Nomad200 robot equipped with
16 ultrasound sensors encircling its upper part and
bumpers. In all the experiments the linear velocity
of the robot was kept constant (15.24 cm/s), and the
robot received the commands it had to execute every
300ms.
We used a set of two layered Kohonen networks to
translate the large number of different situations that
the ultrasound sensors located on the front and right
side of the robot may detect, into a finite set of 220
neurones – states – (R. Iglesias and Barro, 1998).
3.1 Wall Following
To teach the robot how to follow a wall located on
its right at a certain distance interval, we used a rein-
forcement signal that is negative whenever the robot
goes too far or too close from the wall being followed.
The robot was taught how to perform the task in a sim-
ulated training environment, but its performance was
tested in a different one. Convergence was detected
when the greedy policy was able to properly control
the movement of the robot for an interval of 10 min-
utes.
Figure 3: Real robot’s trajectory along a corridor when a
control policy learnt through our approach was used. For
a clear view of the trajectory, figure a) shows the robot’s
movement in one direction and b) shows the movement
along the opposite direction. Points 1 and 2 in both graphs
correspond to the same robot position. The small dots rep-
resent the ultrasound readings.
ICINCO 2007 - International Conference on Informatics in Control, Automation and Robotics
294