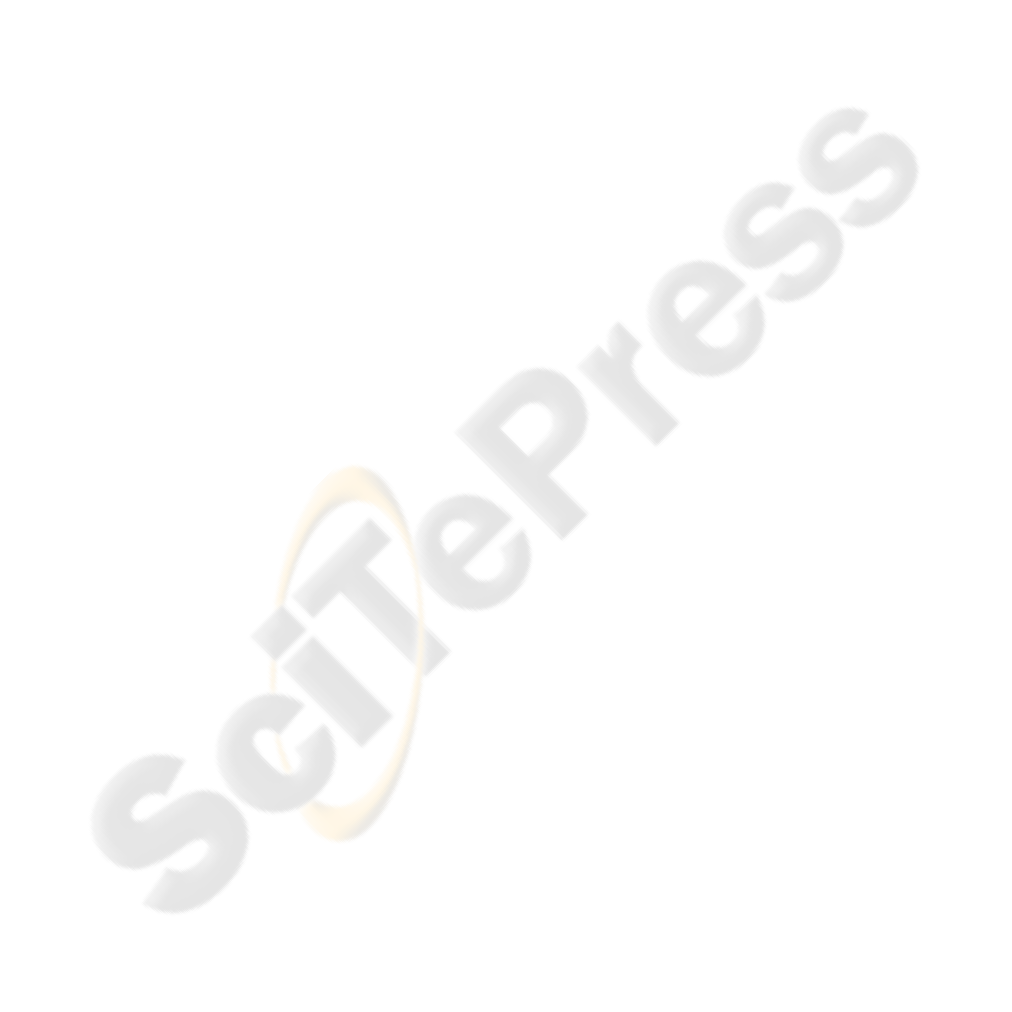
4 CONCLUSIONS
This research is working towards creating an illumi-
nation invariance filter for colour camera input. This
can be used to better identify or correlate objects re-
gardless of changes in lighting conditions or viewing
angle. While white balancing and illumination invari-
ant colour models are on the way to achieving this,
they come across large amounts of noise when trying
to identify colours that have intensities outside of the
sensitive range of the camera. This research has reme-
died this by showing that an equation can be used to
predict how reliable colour values are across an im-
age. In this way correlation between a persistent rep-
resentation and the camera input can be made more
reliably.
5 FUTURE WORK
Now with colour error being accurately predicted in
video, further applications of this research can take
into account this noise and operate more robustly for
it. The current two directions of research following
on from this are image enhancement and frame cor-
relation. Using the overlaps of variance for neigh-
bouring pixels, a pixels colour and variance estimates
are narrowed to become more accurate. This means
slight variations in colour across an object due to cam-
era noise are minimised resulting in a cleaner image
for viewing or vision systems. Frame correlation be-
comes simpler as the computed variance of the pixel
will generally encompass the detected colour of same
physical point in following frames.
REFERENCES
Fintzel, K., Bendahan, R., Vestri, C., Bougnoux, S., Ya-
mamoto, S., and Kakinami, T. (2003). 3d vision sys-
tem for vehicles. In Intelligent Vehicles Symposium,
2003. Proceedings. IEEE, pages 174–179.
Grant, R. N. and Green, R. D. (2004). Tracking colour
movement through colour space for real time human
motion capture to drive an avatar. In Proceedings of
Image and Vision Computing New Zealand.
Lam, H.-K., Au, O. C., and Wong, C.-W. (2004). Automatic
white balancing using standard deviation of rgb com-
ponents. In International Symposium on Circuits and
Systems, volume 3, pages 921–924.
Nummiaro, K., Koller-Meier, E., and Gool, L. J. V. (2002).
Object tracking with an adaptive color-based particle
filter. In Annual Symposium of the German Associa-
tion for Pattern Recognition, page 353 ff.
Salvador, E., Cavallaro, A., and Ebrahimi, T. (2001).
Shadow identification and classification using invari-
ant color models. In IEEE International Conference
on Acoustics, Speech, and Signal Processing, vol-
ume 3, pages 1545–1548.
Takeno, J. and Hachiyama, S. (1992). A collision-avoidance
robot mounting ldm stereo vision. In Proceedings of
the IEEE International Conference on Robotics and
Automation, 1992., volume 2, pages 1740–1752.
Tsuji, T., Hattori, H., Watanabe, M., and Nagaoka, N.
(2002). Development of night-vision system. In IEEE
Transactions on Intelligent Transportation Systems,
volume 3, pages 203–209.
Vergs-Llah, J., Aranda, J., and Sanfeliu, A. (2001). Object
tracking system using colour histograms. In Proceed-
ings of the 9th Spanish Symposium on Pattern Recog-
nition and Image Analysis, pages 225–230.
Wang, J. M., Chung, Y. C., Chang, C. L., and Chen, S. W.
(2004). Shadow detection and removal for traffic im-
ages. In IEEE International Conference on Network-
ing, Sensing and Control, volume 1, pages 649–654.
Yao, J. and Zhang, Z. (2004). Systematic static shadow
detection. In International Conference on IPattern
Recognition, volume 2, pages 76–79.
HUE VARIANCE PREDICTION - An Empirical Estimate of the Variance within the Hue of an Image
9