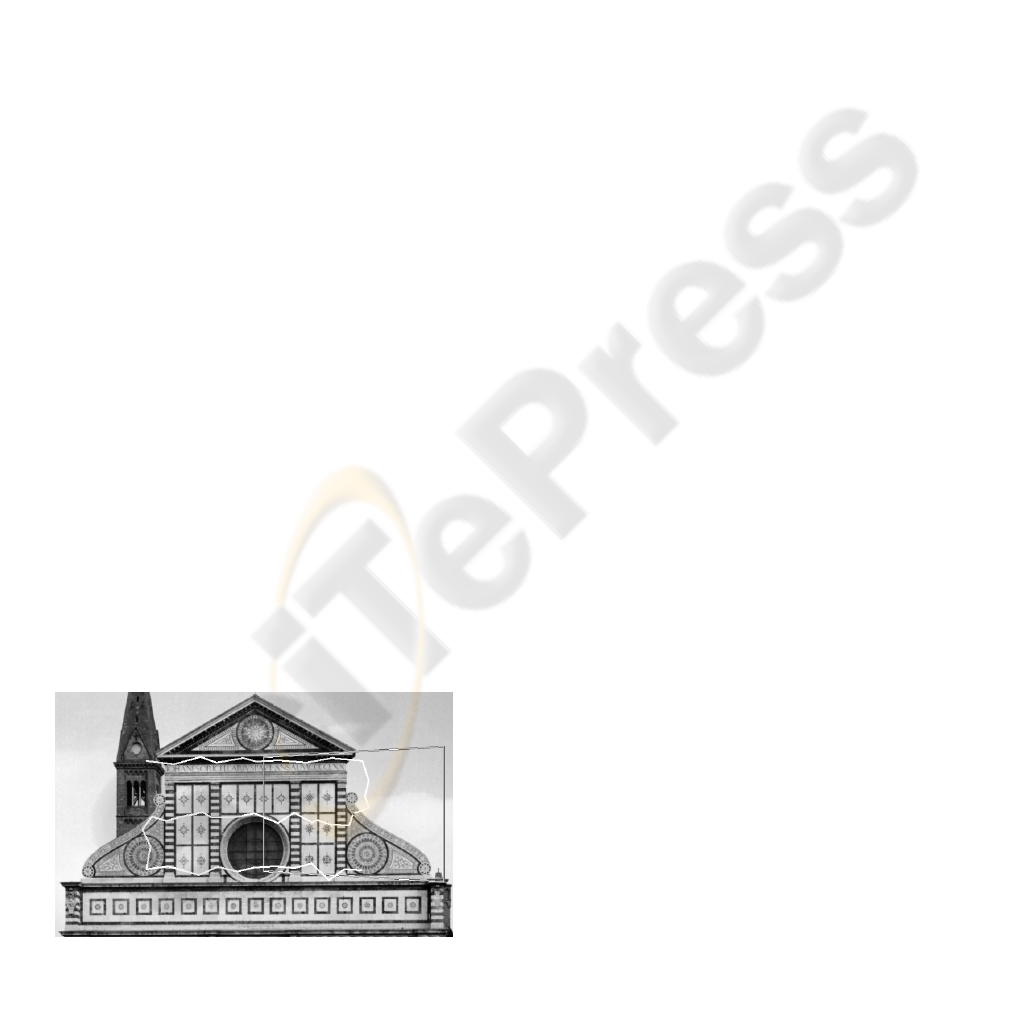
of the quality measurement. According to the con-
ducted tests, the accuracy and robustness of unwarpJ
are suitable for the purpose.
An example of the registration process can be seen
in Figure 6. The topmost image is a mosaic image
created by a stitching algorithm. The middle image
in Figure 6 shows the registered version of the mosaic
above, and the image in the bottom shows the corre-
sponding similarity map (See next subsection). No-
tice how slight stitching errors have prevented flaw-
less matching in the bottom-right corner of the image.
3.3 Similarity Calculation
Our method uses the recent approach of Wang (Wang
et al., 2004) to estimate the quality of the registered
mosaic. The approach of Wang estimates the similar-
ity of two images and gives a single similarity index
value that tells how much alike the two images are.
This method fits very well to the requirements of mo-
saic evaluation, since it pays attention on distortions
that are clearly visible for the human vision system.
This includes blurring and structural changes that are
common problems in mosaics. Wang’s method does
not penalize for slight changes in the image intensity.
This arrangement will notice blurring and discon-
tinuity -flaws in the mosaic images that are created
from test videos. With the current test setup it is not
possible to simulate situations that would cause object
clipping.
4 PRACTICAL TESTS
We used three different stitching algorithms to test the
functionality of our test method: Autostitch (Brown
and Lowe, 2003), Surveillance Stitcher (Heikkil
¨
a and
Pietik
¨
ainen, 2005) and a still unpublished algorithm
Figure 3: Camera motion pattern over a reference image
and a quadrangle depicting the area included in an arbitrary
source image.
called Mobile Stitcher. The algorithms were tested by
three different video sequences that were created from
the images shown in Figure 5.
Each algorithm was tested with the three se-
quences. Results of subjective mosaic evaluation and
quality indexes provided by our algorithm are visible
in Table 2. A detailed analysis concerning one of the
sequences can be found in the caption of Figure 7.
The other results are only shown as pictures due to
space constraints.
We can notice from the results that the acquired
quality indexes are not comparable from one se-
quence to another. The focus of this testing was not to
sort the tested algorithms to some order of quality, but
to simply show what kinds of results can be achieved
with our testing method. When the similarity indexes
were calculated, 50 pixels from each image border
were omitted, since the non-linear registration algo-
rithm was often a bit inaccurate near image borders.
UBC Autostitch acquired the best results from
each test, which can also be detected visually, since
the results are practically absent of discontinuities.
The Surveillance Stitcher acquired second best re-
sults, although most of its results had slight disconti-
nuities. The Mobile Stitcher performed worst in these
tests, which is easily explained by the fact that the al-
gorithm is the only one of the three that uses an area-
based registration (Zitov
´
a and Flusser, 2003) method
and thus is unable to correct perspective distortions.
Figure 8 shows a mosaic that was created by Au-
tostitch along with some modified versions of the mo-
saic. The figure depicts how different kinds of mo-
saicking errors affect the similarity map and the nu-
merical quality of the mosaic. A more detailed expla-
nation can be found in the caption of Figure 8.
A slight setback in the testing was to notice that
the currently used registration method proved to be
inaccurate in one occasion. In Figure 8 each similarity
map indicates that something is wrong in the right end
of the building. However, visual inspection reveals no
problems. The reason behind the indicated difference
is effectively a misalignment of a few pixels. This
should obviously be corrected in the future.
5 CONCLUSION
We have presented a novel way to measure the perfor-
mance of stitching algorithms. The method is directly
applicable to computer-based algorithms that can cre-
ate mosaic images from source image sequences.
The method could be improved by using depth-
varying 3D models, which would test the algorithms’
abilities to cope with effects of occlusion and paral-
EVALUATING STITCHING QUALITY
13