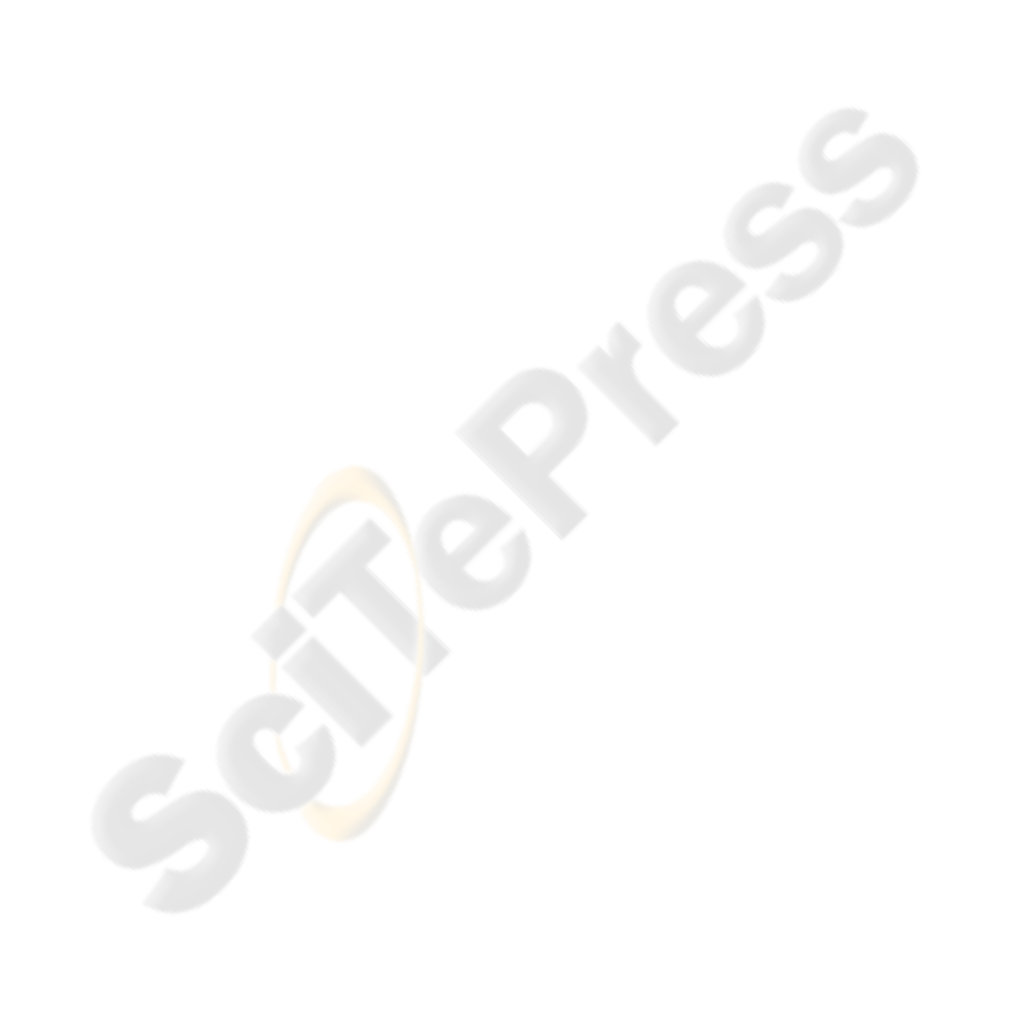
and PCA25-D the curves for real and synthetic follow
the variation very closely.
From Figures 3(a) and (b), it is evident that with
real data 25-D performs better than PCA25-D and
148-D for all values of relevant class size. The infe-
rior performance of PCA25-D can be attributed to the
lack of consideration of the within-class and between-
class variation of data in PCA. As R increases from
10 to 20, precision with 25-D increases by 15.5%
whereas precision with 148-D increases by only 10%.
When R changes from 40 to 50, the improvement in
precision is 5.23% with 25-D but 4.37% with 148-D.
This shows the strength of our feature vector against
the variation in sample size and especially, at small
sample size. In context to CBIR, using a higher scope
means we looking for a larger neighbourhood. This
means precision will fall and recall will increase. In
Figures 4(a) and (b), for DB2, we shown the perfor-
mance of 25-D and 148-D from a different evalua-
tion angle consisting of both precision and recall. In
Figure 4(a), for R=50, at recall = 100%, precision is
18.7% for 25-D whereas it is 12.87% for 148-D. This
means a scope of 267 and 390 respectively. For R=10,
at recall 100%, 25-D shows a precision of 9.78% and
148-D shows 5.2%. This means a scope of 102 for 25-
D and 192 for 148-D. These values clearly indicate
that for lower value of sample size, 25-D performs
even better compared to 148-D.
5 CONCLUSIONS AND FUTURE
DIRECTIONS
1. The online computation with 25-D is much less
compared to 148-D. This reduction in number of
computations will be very significant in today’s
real situation where image database size is already
very big and is increasing day by day.
2. Irrespective of data sets and feature dimension,
synthetic data always performs better than real
data. This is expected as in synthetic data we did
not consider any feature correlation.
3. For 25-D and PCA25-D, with real data set, the
variation of precision with R values follows that
of synthetic data pretty closely, unlike with 148-
D. This means feature re-weighting method where
we assume the features are independent of each
other is more suitable for 25-D compared to 148-
D. We introduced a new parameter, α, to explain
the feature correlation.
4. Irrespective of data set being real or synthetic, for
all feature vectors precision is more sensitive for
smaller R values compared to higher R values.
5. For both DB1 and DB2, with real data, for varying
relevant class size 25-D performs the best.
We find that small sample issue is one of the major
bottlenecks in CBIR research. In the future, we
plan to investigate the small sample issue in more
details. Also, the experiments will be extended to
larger data sets.
REFERENCES
Das, G. and Ray, S. (2005). A compact feature representa-
tion and image indexing in content-based image re-
trieval. In Proceedings of Image and Vision Com-
puting New Zealand (IVCNZ 2005), pages 387–391,
Dunedin, New Zealand.
Duda, R. O., Hart, P. E., and Stork, D. G. (2001). Pattern
Classification, 2nd ed.
Haralick, R. M., Shanmugam, K., and Dinstein, I. (1973).
Textural features for image classification. IEEE
Transactions on Systems, Man, and Cybernetics,
SMC-3, No.6:610–621.
Huang, J. (1998). Color-spatial image indexing and appli-
cations. PhD Dissertation, Cornell University.
Hughes, G. F. (January 1968). On the mean accuracy of
statistical pattern recognizers. IEEE Transactions on
Information Theory, IT-14(1):55–63.
Huijsmans, D. P. and Sebe, N. (February 2005). How to
complete performance graphs in content-based image
retrieval: Add generality and normalize scope. IEEE
transactions on Pattern Analysis and Machine Intelli-
gence, 27(2).
Martinez, A. M. and Kak, A. C. (February 2001). PCA
versus LDA. IEEE Transactions on Pattern Analysis
and Machine Intelligence, 23(2).
Ojala, T., Rautiainen, M., Matinmikko, E., and Aittola, M.
(2001). Semantic image retrieval with hsv correlo-
grams. In Proc. 12th Scandinavian Conference on Im-
age Analysis, pages 621–627, Bergen, Norway.
Shim, S. and Choi, T. (2003). Image indexing by modified
color co-occurrence matrix. In International Confer-
ence on Image Processing .
Sinha, U. and Kangarloo, H. (2002). Principal compo-
nent analysis for content-based image retrieval. Ra-
dioGraphics, (22 (5)):1271–1289.
Spiegel, M. R. (1998). Schaum’s outline series theory and
problems of statistics. McGraw-Hill, 2nd edition,
1998.
Swets, D. and Weng, J. (1996). Using discriminant
eigenfeatures for image retrieval. IEEE Transac-
tions on Pattern Analysis and Machine Intelligence,
18(8):831–836.
Wu, P., Manjunath, B., and Shin, H. (2000). Dimensional-
ity reduction for image retrieval. In Proceeding IEEE
International Conference on Image Processing (ICIP
2000), pages 726–729, Vol. 3, Vancouver, Canada.
VISAPP 2007 - International Conference on Computer Vision Theory and Applications
246