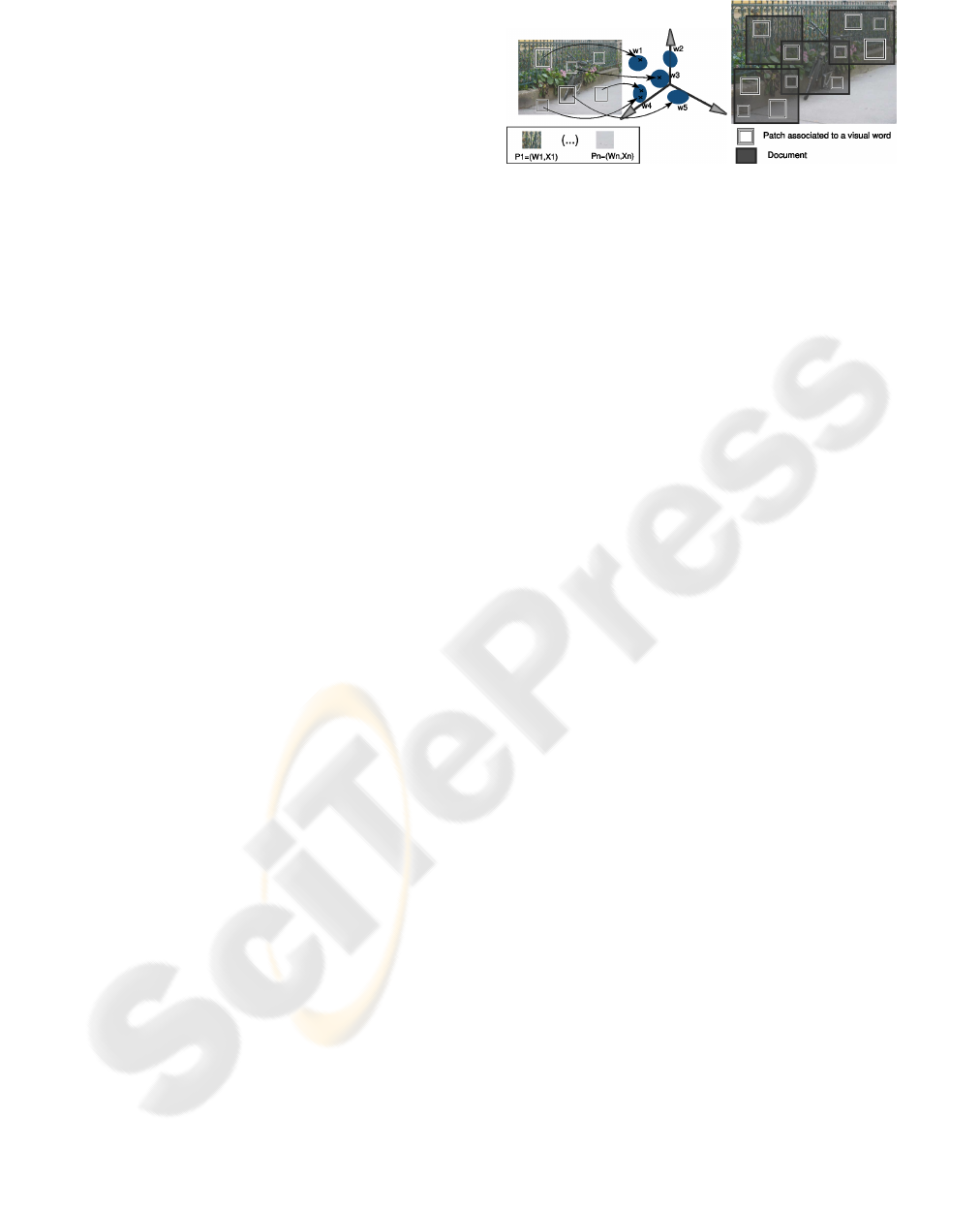
to obtain a category-specific figure-ground segmenta-
tion. Training images are used to build a visual vocab-
ulary of interest points, containing information about
their relative positions as well as their corresponding
segmentation masks.
Borenstein et al.(Borenstein et al., 2004) use the
same idea of selecting informative patches from train-
ing images and then use their segmentation masks
on new unseen images. They combine bottom-up
and top-down approaches into a single process. The
top-down approach uses object representation learned
from examples to detect an object in a new image and
provides an approximation to its segmentation. The
bottom-up approach uses image-based criteria to de-
fine coherent groups of pixels that are likely to belong
to the same part. The resulting combination benefits
from both approaches.
Several approaches propose to use Conditional
Random Field (CRF) for part-based detection (Quat-
toni et al., 2004) or segmentation (Kumar and Hebert,
2006). The previous authors extend the notion of
CRFs to Discriminative Random Fields (DRFs) by
exploiting probabilistic discriminative models instead
of the generative models generally used with CRF.
Kumar et al. (Kumar et al., 2005) propose another
methodology for combining top-down and bottom-up
cues with CRFs. They combine CRFs and pictorial
structures (PS). The PS provides good priors to CRFs
for specific shapes and provides much better results.
None of the previous approaches is able to cope
with occlusion. Win and Shotton (Winn and Shotton,
2006) were the first to address specifically this prob-
lem using an enhanced CRF. Their approach allows
the relative layout (above/below/left/right) of parts to
be modeled, as well as the propagation of long-range
spatial constraints.
1.2 Description of our Approach
Our approach shares many common features with the
previously mentioned approaches. First, it combines
bottom-up and top-down strategies.
The bottom-up process consists in sampling, and
normalizing in size, dense image patches (small
square image sub-windows), as in (Kumar and
Hebert, 2006; Winn and Shotton, 2006), represented
for subsequent processing by SIFT descriptors (Lowe,
2004). These descriptors are then vector quantized
into a discrete set of labels so called visual words.
Each patch is described by the word of the nearest
centroid. This process is illustrated Figure 2. From
this stage, images are seen as sets of visual words oc-
currences. As the process assigns figure/ground la-
bels to patches, the pixel level segmentation requires
Figure 2: The visual vocabulary is obtained by vector quan-
tizing a set of image patches descriptors.Images are mod-
eled as sets of overlapping documents, each document be-
ing a set of patches.
an additional process, responsible for combining la-
bels carried by patches into pixel hypotheses.
The top-down process embeds object models and
uses them to obtain a global coherence, by combining
local information provided by the bottom-up process.
Most of the models previously used in this context
cannot be used here, because of the strong variation
of object’s appearance. Geometric models such as
the Pictorial Structure (Kumar et al., 2005) or the Im-
plicit Shape Model (Leibe and Schiele, 2003) would
require a huge number of training images in order
to capture the large variability of appearance. Ap-
proaches based on characteristic edge patches (Boren-
stein et al., 2004) are only usable when object outlines
are sufficiently stable. As a consequence, it appears
that a more flexible model is required to address such
object categories.
For the recognition of complex object categories,
the bag-of-words model (Csurka et al., 2004) has been
shown to be one of the most effective. It was inspired
from text classification; the text framework becomes
applicable for documents corresponding to images,
once images have been transformed into sets of vi-
sual words. More recently, techniques based on la-
tent aspects were developed on top of this unordered
word based representation and were applied first for
text classification (Griffiths and Steyvers, 2004), and
then for image classification (Sivic et al., 2005). Such
models are usually coming from the probabilist La-
tent Semantic Analysis (pLSA) (Hofmann, 2001) or
its Bayesian form, the Latent Dirichlet Allocation
(LDA) (Blei et al., 2002). Visual words are consid-
ered as generated from latent aspect (or topics) and
images are combination of specific distribution of top-
ics.
Using this latent aspect based framework for seg-
menting images is appealing for several reasons. First
because object appearances (topics) can be automati-
cally discovered and learned, limiting the amount of
supervision required. Second, the flexibility of such
a framework can handle large variations in appear-
CATEGORY LEVEL OBJECT SEGMENTATION - Learning to Segment Objects with Latent Aspect Models
123