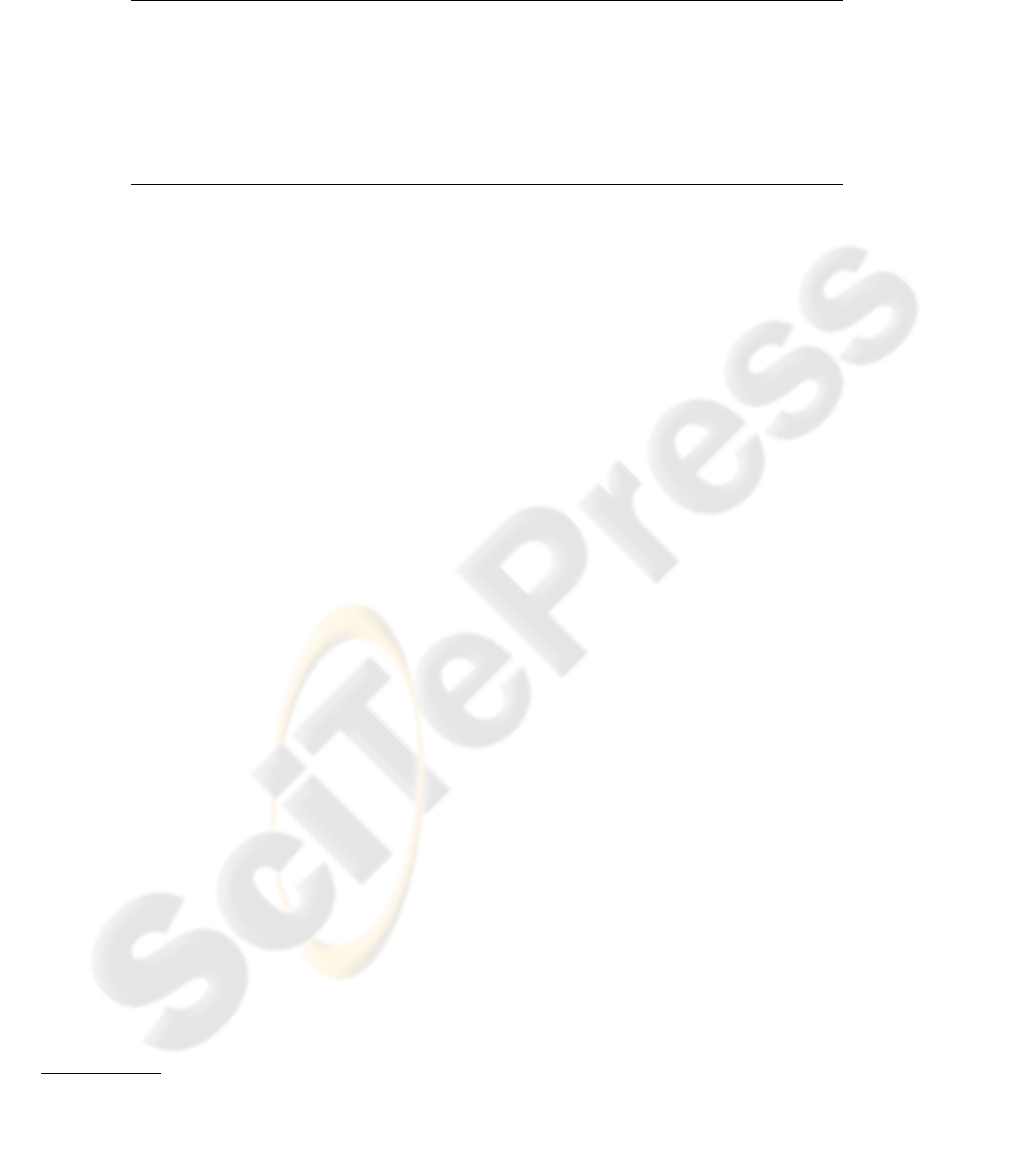
Table 3: Contingency table showing the distribution of the classification of zones of a particular type in percent. (The total
number of errors equals 201 within 13811 tests.) The labels M, L, T, A, D, H, R, S correspond to the types math, logo, text,
table, drawing, halftone, ruling, and speckles, respectively.
M L T A D H R S error [%] # samples
M 90.8 0.0 8.6 0.0 0.0 0.6 0.0 0.0 9.2 476
L 9.1 27.3 36.4 0.0 9.1 9.1 0.0 9.1 72.7 11
T 0.1 0.0 99.8 0.0 0.0 0.0 0.0 0.0 0.2 10450
A 0.8 0.0 20.7 68.6 9.9 0.8 0.0 0.0 31.4 121
D 1.5 0.3 3.0 5.5 86.0 3.5 0.0 0.3 14.0 401
H 0.0 0.9 0.0 0.0 9.7 86.7 0.9 1.8 13.3 113
R 0.4 0.0 1.3 0.0 0.4 0.0 96.1 2.2 3.9 232
S 0.1 0.0 0.5 0.0 0.1 0.1 0.0 99.4 0.6 2007
the need for features based on glyphs or the Fourier
transform. By employing a fast logistic (log-linear)
classifier trained using the maximum entropy crite-
rion on these features, we arrived at a fast and ac-
curate, yet easy to implement overall classifier with
a slightly higher error rate of 2.1%. In our experi-
ments we did not use context information as done in
(Wang et al., 2006) and thus could keep the decision
rule very simple. However, context models are likely
to help in the overall classification and an inclusion
of our approach into Wang et al.’s context model is
possible. Examining the errors made by the system
makes it seem likely that further improvements sig-
nificantly below the reached error rate may be difficult
to achieve without a significantly increased effort, for
example by using a dedicated sub-classifier to distin-
guish between text and table zones.
ACKNOWLEDGEMENTS
We wish to thank Oleg Nagaitsev for help with the im-
plementation and Thomas Deselaers for making avail-
able the open source image retrieval system FIRE,
which provided us with the implementation of some
of the features used. This work was partially funded
by the BMBF (German Federal Ministry of Education
and Research), project IPeT (01 IW D03).
REFERENCES
Deselaers, T., Keysers, D., and Ney, H. (2004). Features for
image retrieval: A quantitative comparison. In DAGM
2004, Pattern Recognition, 26th DAGM Symposium,
volume 3175 of Lecture Notes in Computer Science,
pages 228–236, T
¨
ubingen, Germany.
caused by their distinction between the text classes of dif-
ferent font-sizes and the class ‘other’ with the remaining
classes. On the other hand, we add a new class ‘speckles’,
which is related to 0.15% (21/13811) error.
Guyon, I., Haralick, R. M., Hull, J. J., and Phillips, I. T.
(1997). Data sets for OCR and document image un-
derstanding research. In Bunke, H. and Wang, P.,
editors, Handbook of character recognition and doc-
ument image analysis, pages 779–799. World Scien-
tific, Singapore.
Inglis, S. and Witten, I. (1995). Document zone classifica-
tion using machine learning. In Proc Digital Image
Computing: Techniques and Applications, pages 631–
636, Brisbane, Australia.
Keysers, D., Och, F.-J., and Ney, H. (2002). Maximum en-
tropy and Gaussian models for image object recogni-
tion. In Pattern Recognition, 24th DAGM Symposium,
volume 2449 of Lecture Notes in Computer Science,
pages 498–506, Z
¨
urich, Switzerland. Springer.
Kise, K., Sato, A., and Iwata, M. (1998). Segmentation of
page images using the area Voronoi diagram. Com-
puter Vision and Image Understanding, 70(3):370–
382.
Liang, J., Phillips, I., Ha, J., and Haralick, R. (1996). Doc-
ument zone classification using the sizes of connected
components. In Proc. SPIE, volume 2660, Document
Recognition III, pages 150–157, San Jose, CA.
Okun, O., Doermann, D., and Pietikainen, M. (1999). Page
Segmentation and Zone Classification: The State of
the Art. Technical Report LAMP-TR-036, CAR-TR-
927, CS-TR-4079, University of Maryland, College
Park.
Sivaramakrishnan, R., Phillips, I. T., Ha, J., Su bramanium,
S., and Haralick, R. M. (1995). Zone classification in
a document using the method of feature vector genera-
tion. In ICDAR ’95: Proceedings of the Third Interna-
tional Conference on Document Analysis and Recog-
nition (Volume 2), page 541ff.
Wang, Y., Haralick, R., and Phillips, I. (2000). Improve-
ment of zone content classification by using back-
ground analysis. In Fourth IAPR International Work-
shop on Document Analysis Systems (DAS2000).
Wang, Y., Phillips, I. T., and Haralick, R. M. (2006). Doc-
ument zone content classification and its performance
evaluation. Pattern Recognition, 39:57–73.
Wong, K. Y., Casey, R. G., and Wahl, F. M. (1982). Doc-
ument analysis system. IBM Journal of Research and
Development, 26(6):647–656.
VISAPP 2007 - International Conference on Computer Vision Theory and Applications
50