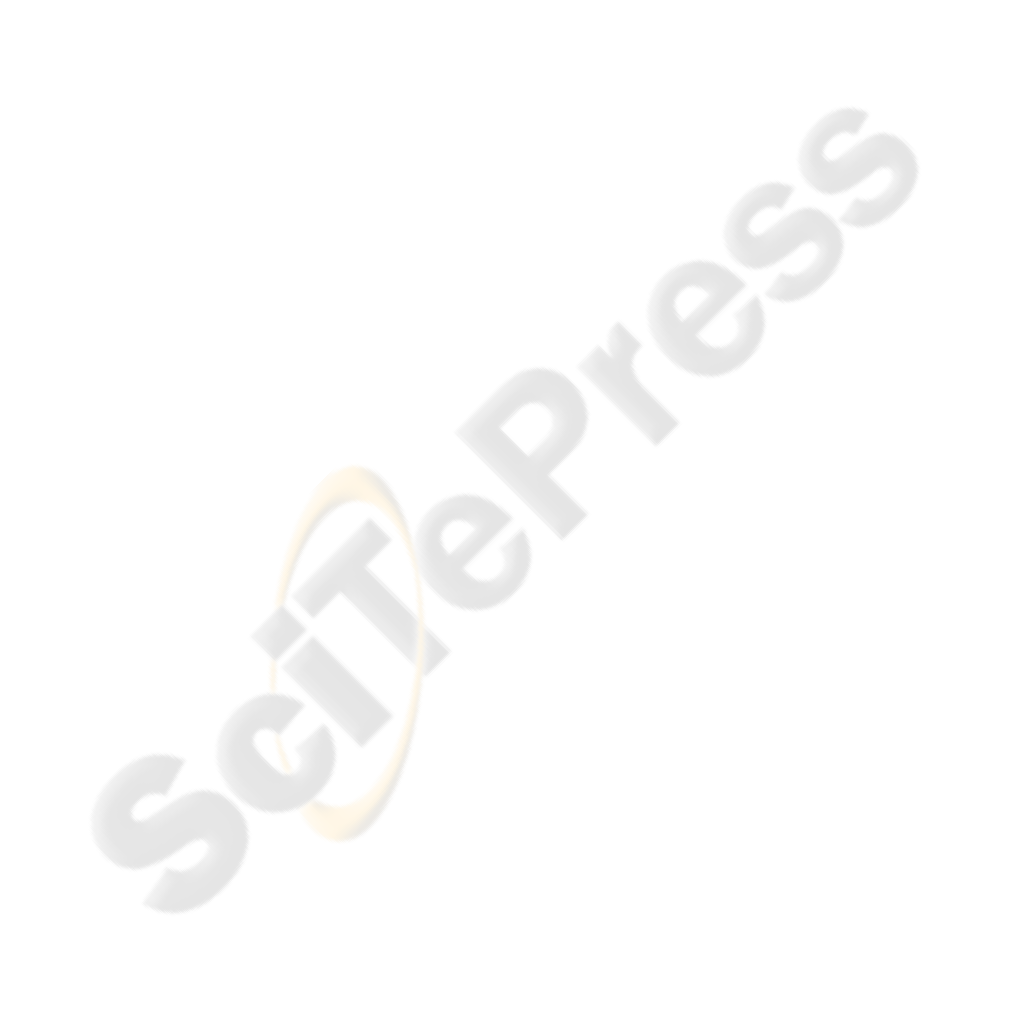
parameter of the query, the large scale factor on Q
1
,
against the reductions on Q
2
and Q
3
, denotes that the
tennis balls with a stamped black brand are of special
interest. A close look in the first four images of the
retrieved set shows that this query specification was
attended. Finally, note that the system should return
15 images in the final retrieval, but 12 images were
presented. In this example, this occurred because 3
of the 15 images in the individual retrievals (Figure
5) appeared twice. So, that repeated images had their
relevance scores summed, and appeared just once in
the final retrieval.
4 CONCLUSION
The architecture presented in this paper incorpo-
rates different techniques for visual content access:
object-based image retrieval, the ordinary global-
based image retrieval and multiple examples query.
Most CBIR systems use these approaches isolated or
weakly integrated, while here they were truly com-
bined. Moreover, by using the PRISM interface it
is possible to get explicit information from the user
about the relevance of each example image. This is
done by means of images scale. Taking these charac-
teristics together the system is able to more faithfully
capture what users have in mind when formulating a
query, as was demonstrated by experimental results.
The obtained results should encourage CBIR de-
velopers to put effort not only towards the traditional
feature extraction-distance measurement paradigm,
but also into the improvement of the architectural as-
pects concerning the capture of user query concepts.
In the next stages of this work we intend to explore
in more detail the information provided by the user
to the interface, such as the arrangement of example
images and the features of images dragged to the trash
can.
ACKNOWLEDGEMENTS
This research was partially sponsored by UOL
(www.uol.com.br), through its UOL Bolsa Pesquisa
program, process number 200503312101a and by the
Office of Naval Research (ONR) under the Center for
Coastline Security Technology grant N00014-05-C-
0031.
REFERENCES
Assfalg, J., Del Bimbo, A., and Pala, P. (2000). Using
multiple examples for content-based image retrieval.
In IEEE International Conference on Multimedia and
Expo (I), pages 335–338.
Carson, C., Belongie, S., Greenspan, H., and Malik, J.
(2002). Blobworld: Image segmentation using ex-
pectation-maximization and its application to image
querying. IEEE Transactions on PAMI, 24(8):1026–
1038.
Castelli, V. and Bergman, L. D. (2002). Image Databases:
Search and Retrieval of Digital Imagery. John Wiley
& Sons, Inc., New York, NY, USA.
Garc
´
ıa-P
´
erez, D., Mosquera, A., Berretti, S., and Del
Bimbo, A. (2006). Object-based image retrieval us-
ing active nets. In ICPR (4), pages 750–753. IEEE
Computer Society.
Itti, L., Koch, C., and Niebur, E. (1998). A model of
saliency-based visual attention for rapid scene anal-
ysis. IEEE Trans. on PAMI, 20(11):1254–1259.
Manjunath, B. S., Ohm, J. R., Vinod, V. V., , and Yamada,
A. (2001). Color and texture descriptors. IEEE Trans.
Circuits and Systems for Video Technology, Special Is-
sue on MPEG-7, 11(6):703–715.
Marques, O., Mayron, L. M., Borba, G. B., and Gamba,
H. R. (2007). An attention-driven model for group-
ing similar images with image retrieval applications.
EURASIP Journal on Applied Signal Processing (ac-
cepted).
Mayron, L. M., Borba, G. B., Nedovic, V., Marques, O.,
and Gamba, H. R. (2006). A forward-looking user
interface for cbir and cfir systems. In IEEE Inter-
national Symposium on Multimedia (ISM2006), San
Diego, CA, USA.
Renninger, L. W. and Malik, J. (2004). When is scene iden-
tification just texture recognition? Vision Research,
44(19):2301–2311.
Rui, Y., Huang, T., Ortega, M., and Mehrotra, S. (1998).
Relevance feedback: A power tool for interactive
content-based image retrieval. IEEE Transactions on
Circuits and Systems for Video Technology, 8(5):644–
655.
Santini, S. and Jain, R. (2000). Integrated browsing and
querying for image databases. IEEE MultiMedia,
7(3):26–39.
Smeulders, A., Worring, M., Santini, S., Gu pta, A., and
Jain, R. (2000). Content-based image retrieval at
the end of the early years. IEEE Trans. on PAMI,
22(12):1349–1380.
Stentiford, F. (2001). An estimator for visual attention
through competitive novelty with application to im-
age compression. In Picture Coding Symposium, pp.
25-27, Seoul, Korea.
Tang, J. Acton, S. (2003). An image retrieval algorithm us-
ing multiple query images. In ISSPA’03: Proceedings
of the 7th International Symposium on Signal Process-
ing and Its Applications, pages 193–196. IEEE.
VISAPP 2007 - International Conference on Computer Vision Theory and Applications
148