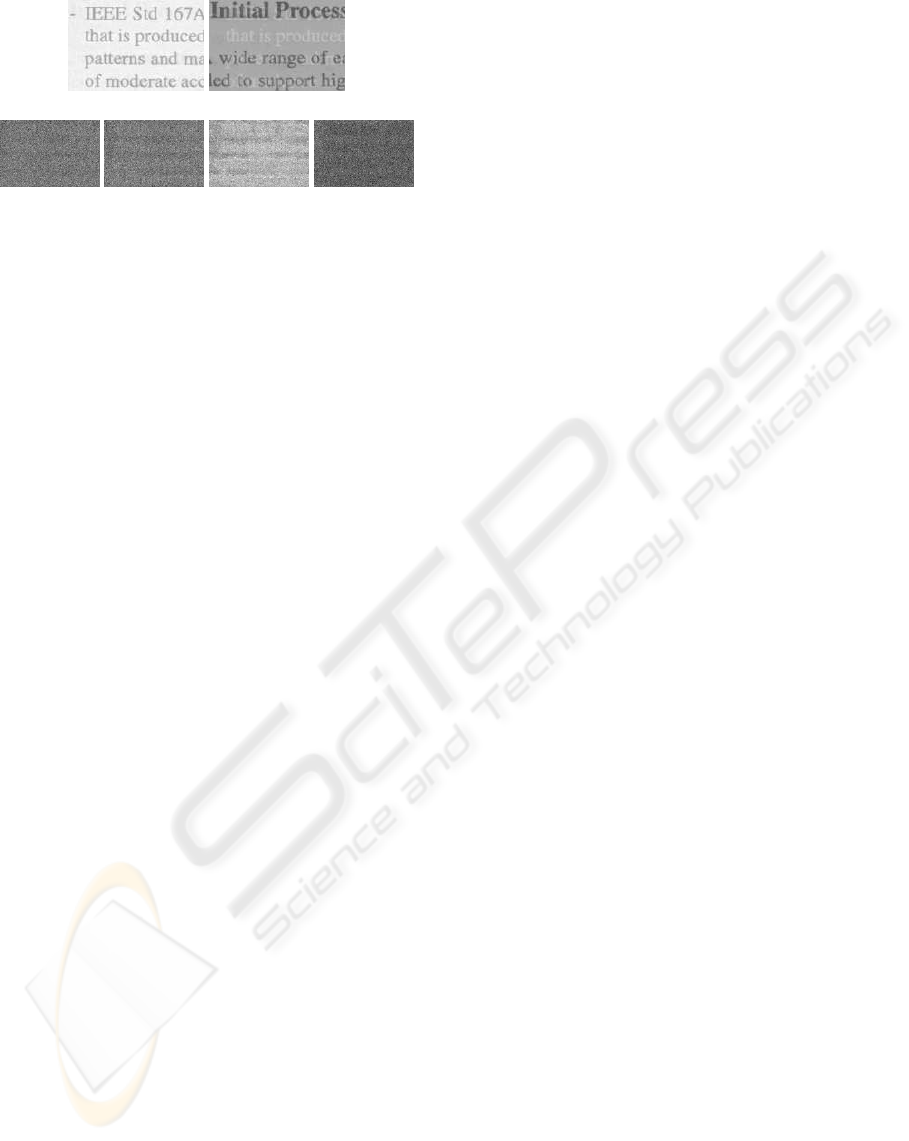
(a) two ICs corresponding to the demixed sources
(b) other ICs containing noise-like signals
Figure 4: Separation results by ICA.
mental implementation can come up to hours without
specific optimizations. However, various computing
alternatives such as Mean Field and variational ap-
proximation can be exploited to achieve higher effi-
ciency.
5 CONCLUSION
We proposed a Bayesian approach for separating
noisy linear mixture of document images. For source
images, we considered a hierarchical model with
the hidden label variable z representing the common
classification of objects among multiple color chan-
nels, and a Potts-Markov prior was employed for the
class labels imposing local regularity constraints. We
showed how Bayesian estimation of all unknowns of
interest can be computed by MCMC sampling from
their posterior distributions given the observation. We
then illustrated the feasibility of the proposed algo-
rithm on joint separation and segmentation by tests
on sample images.
REFERENCES
Almeida, L. B. (2005). Separating a real-life nonlinear im-
age mixture. Journal of Machine Learning Research,
6:1199–1232.
Bronstein, A. M., Bronstein, M. M., Zibulevsky, M., and
Zeevi, Y. Y. (2005). Sparse ICA for blind separation
of transmitted and reflected images. Intl. Journal of
Imaging Science and Technology (IJIST), 15:84–91.
Calhoun, V. D. and Adali, T. (2006). Unmixing fMRI with
independent component analysis. IEEE Engineering
in Medicine and Biology Magazine, 25(2):79–90.
Castella, M. and Pesquet, J.-C. (2004). An iterative blind
source separation method for convolutive mixtures
of images. Lecture Notes in Computer Science,
3195/2004:922–929.
Drira, F. (2006). Towards restoring historic documents
degraded over time. In Second International Con-
ference on Document Image Analysis for Libraries,
pages 350–357.
Feron, O. and Mohammad-Djafari, A. (2005). Image fusion
and unsupervised joint segmentation using HMM and
MCMC algorithms. Journal of Electronic Imaging,
14(2).
Harmeling, S. (2003). Kernel-based nonlinear blind source
separation. Neural Computation, 15(5):1089–1124.
Hyvarinen, A. (1999). Fast and robust fixed-point algo-
rithms for independent component analysis. IEEE
Transactions on Neural Networks, 10(3):626–634.
Hyvarinen, A., Karhunen, J., and Oja, E. (2001). Indepen-
dent Component Analysis. John Wiley & Sons, Inc.,
New York.
Macias-Macias, M., Garcia-Orellana, C. J., Gonzalez-
Velasco, H., and Gallardo-Caballero, R. (2003). In-
dependent component analysis for cloud screening of
meteosat images. In International Work-conference
on Artificial and Natural Neural Networks (LNCS
2687/2003), volume 2687, pages 551–558.
Parra, L., Spence, C., Ziehe, A., Muller, K.-R., and Sajda, P.
(2000). Unmixing hyperspectral data. In Advances in
Neural Information Processing Systems, volume 12,
pages 942–948.
Sharma, G. (2001). Show-through cancellation in scans of
duplex printed documents. IEEE Transactions on Im-
age Processing, 10(5):736–754.
Snoussi, H. and Calhoun, V. D. (2005). Bayesian blind
source separation for brain imaging. In IEEE Interna-
tional Conference on Image Processing (ICIP) 2005,
volume 3, pages 581–584.
Snoussi, H. and Mohammad-Djafari, A. (2004). Fast joint
separation and segmentation of mixed images. Jour-
nal of Electronic Imaging, 13:349–361.
Tonazzini, A., Bedini, L., and Salerno, E. (2006). A markov
model for blind image separation by a mean-field EM
algorithm. IEEE Transactions on Image Processing,
15(2):473–482.
Tonazzini, A., Salerno, E., Mochi, M., and Bedini, L.
(2004). Blind source separation techniques for de-
tecting hidden texts and textures in document images.
In ICIAR 2004, LNCS 3212, pages 241–248, Berlin.
Springer-Verlag.
VISAPP 2007 - International Conference on Computer Vision Theory and Applications
156