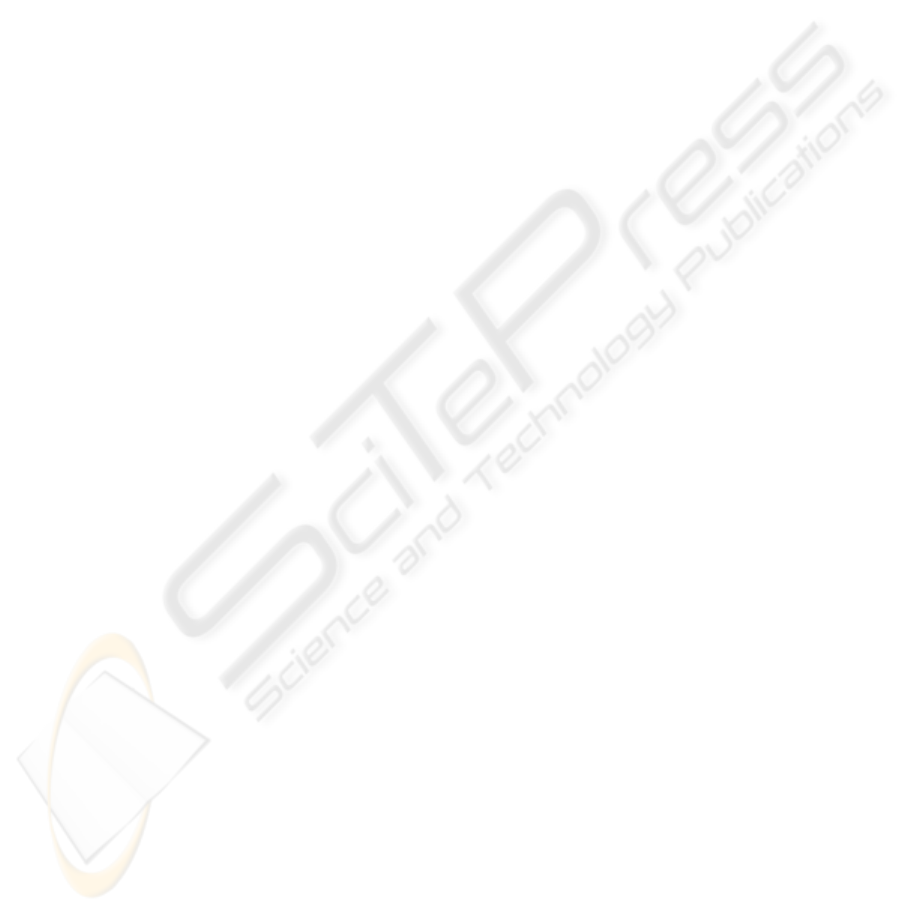
of the above features. Thus, the following 47
features were chosen to describe nuclei morphology
(Churakova, Gurevich, et.al., 2003): an area of
nucleus in pixels, 4 statistical features calculated on
nucleus brightness histogram (average, dispersion,
3rd and 4th central moments); 16 granulometric
features of nucleus; 26 features calculated on the
Fourier-spectrum of nucleus. Further steps included
statistical and factor analysis of features and cluster
analysis of nuclei on appropriate sets of features.
Statistical and qualitative analysis included:
feature correlations, feature distribution, feature
histograms and their moments; feature “robustness”
to variations of nuclei geometry. The analysis
allowed excluding unstable and high-correlated
features. The feature values distribution was
estimated by Shapiro-Wilks W-test. It turned out
that the distributions for majority of features are not
normal once.
After calculation of descriptive nonparametric
statistics (medians and quartiles) it is appeared that
values of some textural features are grouped within
3 separated areas, i.e. the considered nuclei are
divided into 3 types. In case of CLL, cytological
slides contain mainly “mature” nuclei. In slides
corresponding to TRCLL one can find “mature”
nuclei as well as “transformed” nuclei. LS is
characterized by a larger percent of transformed”
nuclei. Since well-known for experts distribution of
“mature” and “transformed” nuclei over diagnosis
coincides with its distribution over obtained types, it
is possible to conclude that these nuclei types
correspond to “mature” and “transformed” nuclei.
Cluster sets were obtained by application of
FOREL algorithm (Zagoruiko, 1999) to different
sets of features. The sets of clusters were evaluated
using different criteria: number and size of clusters
in each set (large clusters contained more than 400
nucleus), total percent of all nuclei belonging to
large clusters, the character of nuclei distribution in
large clusters. In the considered problem a taxonomy
with a few large clusters accumulating the main part
of nuclei is more preferable than a taxonomy with a
lot of small clusters where nuclei distribution over
clusters is uniform.
A new method to create feature description of a
patient was suggested. On the basis of cluster
analysis results a patient is described by a new type
of features - percentage of a patient’s nuclei
belonging to large clusters of the taxonomy.
Experiments showed that good classification results
can be obtained in such feature space.
Factor analysis was conducted for reducing of
the feature space. It was applied to several data sets:
a) all 8702 available nuclei; b) the sample
corresponding to 4 different diagnosis; c) samples
for each patient. Three techniques were used:
principal factor, centroid and maximum-likelihood.
The combination of the Kaizer criterion and the
scree-test was used to determine a number of factors,
while varimax-rotate strategy was used to calculate
factor loadings. Each method yielded the same
number of factors for each data set. The mean factor
loadings were calculated for three data sets. As a
result, the same factors were discovered. Similarity
of factors was confirmed by presence of high factor
loadings on the same features and, accordingly, by
presence of low factor loadings on the rest features.
Then, features with high factor loadings (its absolute
values exceed some threshold) were determined for
each obtained factor.
It is important that selection of the same factors
for data sets corresponding to different diagnoses
gives the corresponding sets of features with high
factor loadings differing substantially. It means that
for different diseases considered in our study there
are different significant features. It also appeared
that there are unique significant features for some
diseases that are not significant for the other
diseases.
We developed a new diagnostic procedure based
on factor patterns designed for considered deceases.
The pattern represents high-loadings-feature
distribution over factors extracted from the sample
corresponding to certain decease. Such distributions
are different for different deceases. If the factor
pattern of a new patient coincides with the pattern of
a particular decease, we could consider that this
patient has such decease.
The cluster analysis of nuclei was done on the
base of the results of factor analysis. For initial
minimization of the feature space Spearman R-
statistic was used. Further the features with high
loadings were selected from obtained set. It
appeared that the taxonomy structure (amount of
large clusters and their nuclei proportions) is
determined by only 3 features. The values of these
features are concentrated into separated areas. The
rest of features influences only on total amount and
size of clusters, however the nuclei proportions in
large clusters are the same (see Table 1, “+” means
the presence of explicit partition to malignant and
non-malignant groups).
VISAPP 2007 - International Conference on Computer Vision Theory and Applications
206