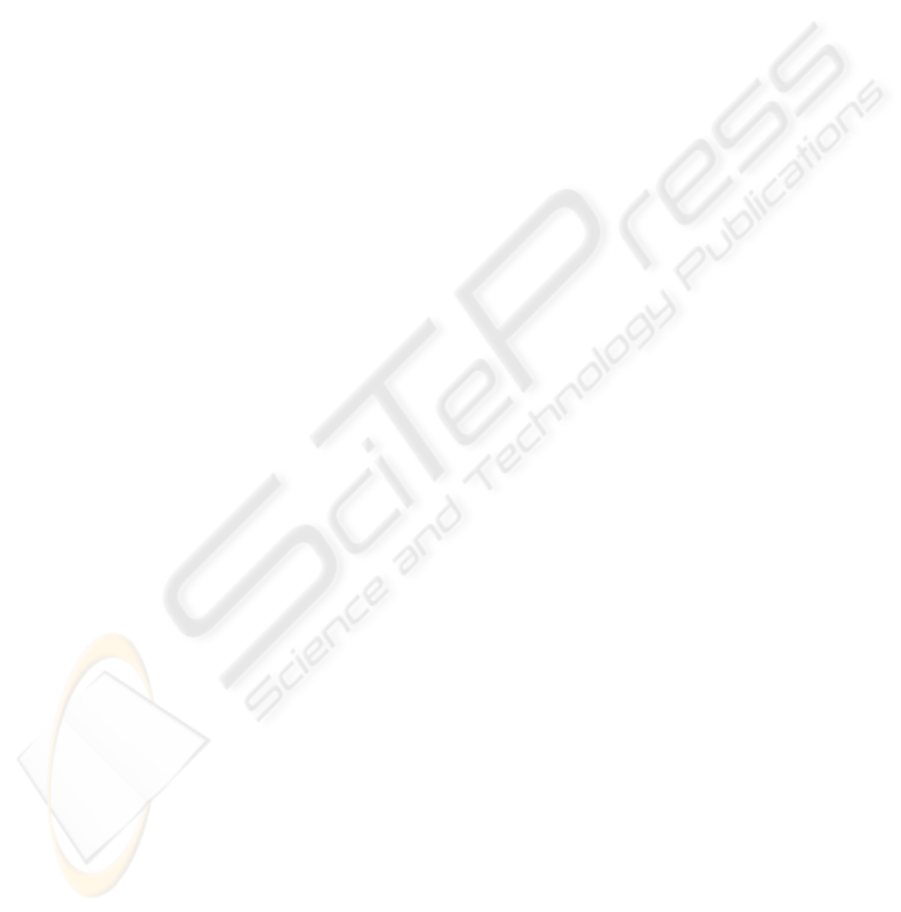
fringe on the forehead, glasses and the head
inclination disarray the system.
Based on many tests, anyone could see that each
face region geometry vary a lot, mainly on the
forehead, chin and nose regions. In these situations,
some changes were made on the adaptation model.
We saw also, that the use of glasses can distort the
geometry in the nose region, once the features are
not extracted adequately on the side image.
5 CONCLUSION
This study showed the real possibility to acquire two
images in different moments and process their to
obtain a realistic 3D model of a human face. We also
could see that the illumination homogeneity is
important, but not fundamental. We have got good
results without any additional care about it.
The real possibility to do all process
automatically could be proved. The only human
interference occurs in the initial moment to adjust
the window to limit the model head, all the other
process are made automatically and rapidly.
The time consuming for all execution procedure
is not greater than 32 seconds. This efficiency comes
from that the system knows in advance which
characteristics will be searched, decreasing the
computational effort.
In order to make the process happen without
human interference, it was developed a correction
algorithm for the head inclination, which provided
and excellent alignment between the two images,
making the work of feature research. We should
highlight that the algorithm has its limitations and,
for inclinations over 30%, it can fail, however in
normal conditions, it was showed efficient and it did
not accumulate errors to the synthetic images.
Moreover to extract the features correctly, it is
advisable that the models not present any element
that obstructs some regions of the face.
An improvement of this work would be the
identification of elements, such as, the use of
glasses, fringe and beard. With this recognition, such
occurrences could be eliminated and make the
process possible.
REFERENCES
Akimoto, T., Suenaga, Y., Richard, S.W., 1993.
Automatic creation of 3D facial models, IEEE
Computer Graphics and Applications.
Kurihara, T., Arai, K.,1991. A transformation method for
modeling and animation of the human face from
photographs. In Proceedings of Computer
Animation’91.
Lee, W., Kalra, P., Magnenat-Thalmann, N., 1997. Model
based face reconstruction for animation. In
Proceedings of MMM’97. World Scientific Press.
Lee, W., Magnenat-Thalmann, N., 2000. Fast head
modeling for animation. J. Image Vision Computer. 18
(4).
Coelho, M.M., 1999. Localização e reconhecimento de
características faciais para a codificação de vídeo
baseada em modelos. Master’s dissertation, ITA, São
José dos Campos.
Bravo, D.T., 2006. Modelagem 3D de faces humanas
baseada em informações extraídas de imagens
bidimensionais não calibradas. Master’s dissertation,
ITA, São José dos Campos.
Pandzic, I.S., Forchheimer, R., 2002. MPEG-4 Facial
Animation: the standard, implementation and
applications, John Wiley & Sons. England.
Vezhnevets, V. et al., 2004. Automatic extraction of
frontal facial features. In 6th Asian Conference on
Computer Vision.
Parke, F.I., Waters, K., 1996. Computer Facial Animation,
AK Peters. EUA.
Lindley, C., 1991. Practical image processing in C:
acquisition, manipulation, storage, Jhon Wiley &
Sons. New York.
Lanzarotti, R. et al., 2001. Automatic features detection
for overlapping face images on their 3D range models.
In 11th International Conference on Image Analysis
and Processing.
Goto, T. et al., 2001. Facial feature extraction for quick
3D face modeling. In Signal Processing: Image
Communication.
Ansari, A-N., Abdel-Mottaleb, M., 2003. 3-D face
modeling using two views and a generic face model
with application to 3-D face recognition. In IEEE
Conference on Advanced Video and Signal Based
Surveillance.
2D IMAGES CALIBRATION TO FACIAL FEATURES EXTRACTION
129