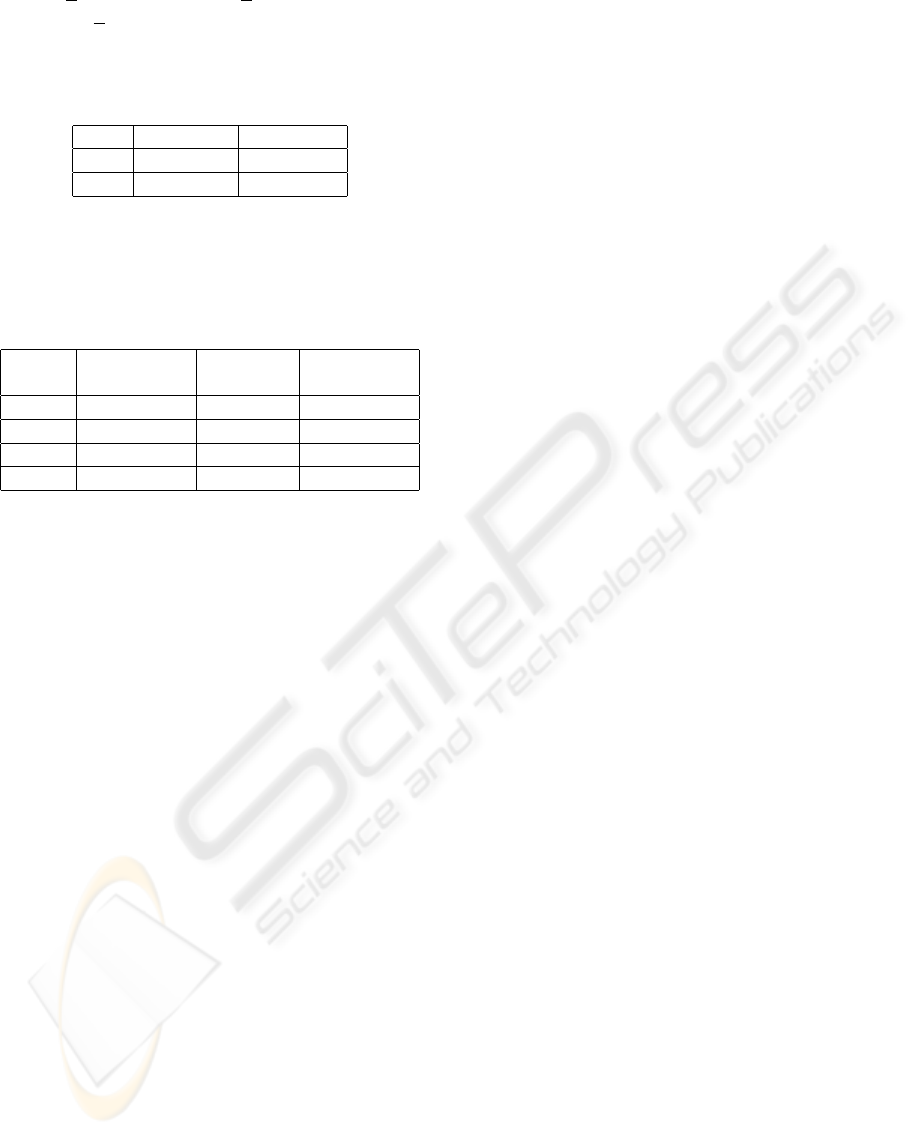
squared ρ
2
. We can see that ρ
2
in NL1 (0.4448) is
higher than
ρ
2
(0.6145) in the EM model. This is re-
lated to the results in Table 5. Table 9 summarizes the
Table 8: Adjusted rho squared.
NL model EM model
NL1 0.4448 0.6145
NL2 0.5757 0.5954
results for the four models. It confirms that the EM
model is the most appropriate of these models.
Table 9: Nesting coefficient of NL2 in the EM algorithm.
Model Number of
parameters
Rho-
squared
Adjusted
rho-squared
MNL 26 0.4460 0.4450
NL1 31 0.4460 0.4448
NL2 30 0.5770 0.5757
EM 61 0.5895 0.5870
5 CONCLUSION AND FUTURE
WORK
We tested our model and confirmed that there exist
some decision-making processes. As the result, we
were able to confirm the class NL1 by using EM al-
gorithm. The class model was constructed with the
model including individual variables. Therefore we
could find that what kinds of customers are classified
into certain class. The model is expected to be more
appropriate model when we construct the model with
the larger number of the classes. We think that the ad-
justed rho squared of the model with EM algorithm
did not show much improvement when we assume
there are two decision-making processes, because the
class model could not classify the customers well.
Moreover, the reason why the adjusted rho squared
did not take the higher value is that the number of
customers using an NL1 type decision-making pro-
cess could be small. These are future works. We
would like to consider a more appropriate model by
extracting the dominant decision-making process.
REFERENCES
Ben-Akiva, M. and Gershenfeld, S. (1998). Multi-featured
products and services : analysing pricing and bundling
strategies. Journal of Forecasting, 17:175–196.
Ben-Akiva, M. and Lerman, S. R. (1985). Discrete Choice
Analysis:Theory and Application to Travel Demand.
The MIT Press, Cambridge Massachusetts.
Bierlaire, M. (2005). Biogeme. http://roso.epfl.ch/biogeme.
Copeland, T. and Antikarov, V. (2001). Real Options : A
Practitioner’s Guide. Thomson Texere.
Fildes, R. and Kumar, V. (2002). Telecommunications de-
mand forecasting –a review. International Journal of
Forecasting, 18:489–522.
Inoue, A., Nishimatsu, K., and Takahashi, S. (2003a).
Multi-attribute learning mechanism for customer sat-
isfaction assessment. Intelligent Engineering Systems
Through Artificial Neural Networks, 13:793–800.
Inoue, A., Takahashi, S., Nishimatsu, K., and Kawano,
H. (2003b). Service demand analysis using multi-
attribute learning mechanisms. In KIMAS 2003, pages
634–639, MA Cambridge. IEEE.
Kurosawa, T., Inoue, A., and Nishimatsu, K. (2006). Tele-
phone service choice-behavior modeling using menu
choice data under competitive conditions. Intelligent
Engineering Systems Through Artificial Neural Net-
works, 16:711–720.
Kurosawa, T., Inoue, A., Nishimatsu, K., Ben-Akiva, M.,
and Bolduc, D. (2005). Customer-choice behavior
modeling with latent perceptual variables. Intelligent
Engineering Systems Through Artificial Neural Net-
works, 15:419–426.
Loomis, D. G. and Taylor, L. D., editors (1999). THE FU-
TURE OF THE TELECOMMUNICATIONS INDUS-
TRY : Forecasting and Demand Analysis. Kruwer
Academic publishers.
Loomis, D. G. and Taylor, L. D., editors (2001). FORE-
CASTING THE INTERNET : Understanding the Ex-
plosive Growth of Data Communications. Kruwer
Academic publishers.
Manski, C. F. (1977). The structure of random utility mod-
els. Theroy and Decision, 8:229–254.
Nishimatsu, K., Inoue, A., and Kurosawa, T. (2004). Traffic
profiling method for service demand analysis. Intelli-
gent Engineering Systems Through Artificial Neural
Networks, 14:647–652.
Nishimatsu, K., Inoue, A., and Kurosawa, T. (2006).
Service-demand-forecasting method using multiple
data sources. In Networks.
Nishimatsu, K., Inoue, A., Kurosawa, T., Ben-Akiva, M.,
and Bolduc, D. (2005). Service demand analysis : Im-
proved forecasting using model updating. Intelligent
Engineering Systems Through Artificial Neural Net-
works, 15:691–698.
Savage, S. J. and Waldman, D. (2004). United States de-
mand for internet access. Review of Network Eco-
nomics, 3(3).
Takahashi, S., Inoue, A., Kawano, H., and Nishimatsu, K.
(2004). Scenario simulation based on stated prefer-
ence model. In Simulation Workshop, pages 199–205.
Operational Research Society.
CUSTOMER BEHAVIOR MODELING UNDER SEVERAL DECISION-MAKING PROCESSES BY USING EM
ALGORITHM
345