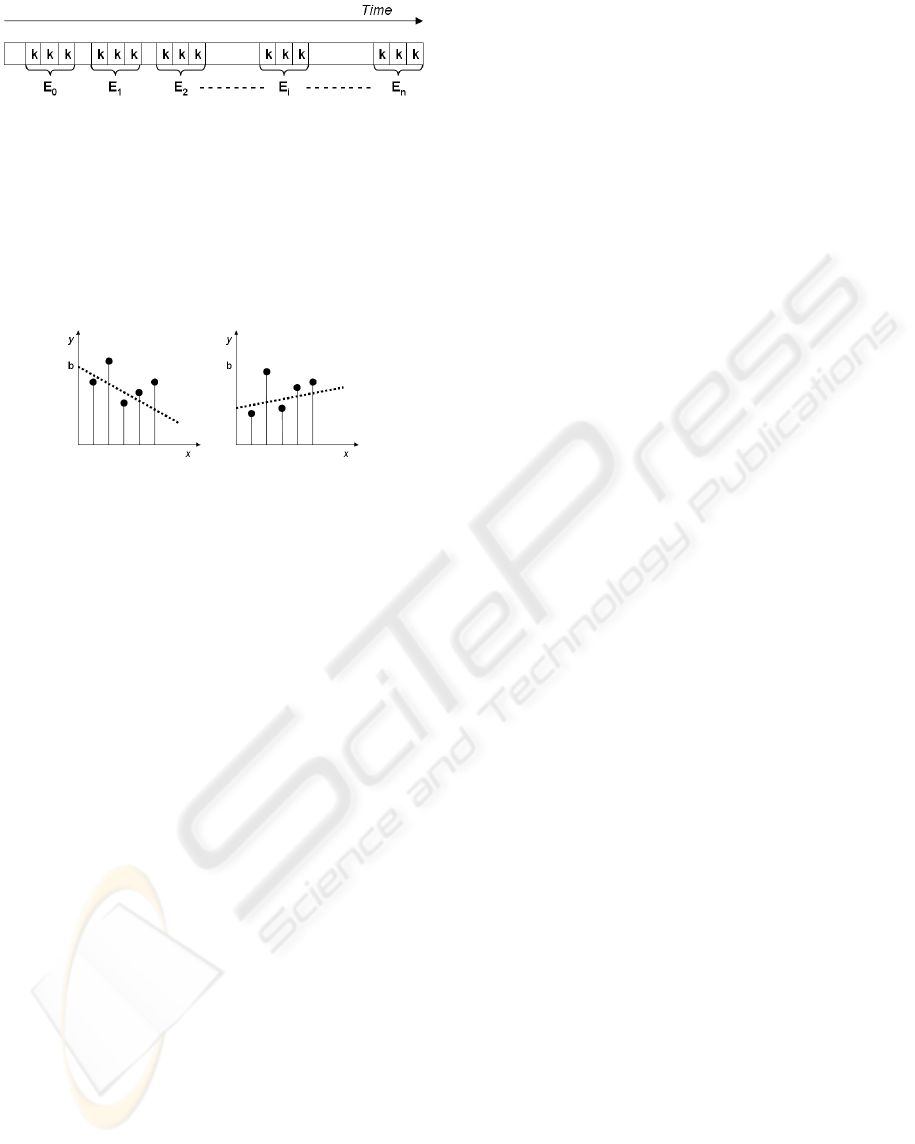
Figure 1: Collect of samples by triplet in a sound file.
On each sample k of each triplet, we compute the
distribution of frequencies within the meaning of
Fourier and the directing coefficient p of the straight
regression line binding the level (y) to each class of
the spectrum frequency (y). This straight regression
line is expressed in the following way: y = px + b.
Figure 2: The slope of the spectrum of 2 elements of a
triplet.
The analysis of the behaviour of p (slope of the
line side of figure 2) will contribute to evaluate the
rhythmic behaviour by measuring the swinging of
the spectrum over one period, and in average value
on the various samples. By reference to mechanics,
this swinging, its speed and its acceleration are
evaluated as follows.
The first stage consists in identifying the number
of triplets as well as their position in the signal. On a
fraction of the sound file, we extract the first triplet
on which we calculate the 3 spectra then the
directing coefficients of the straight regression lines.
Thus 3 values of slope (p11, p12, p13) are obtained.
The speed of swinging is obtained by calculating the
difference between 2 consecutive slopes. We obtain
2 values of speed (v11, v12) for each triplet.
Acceleration a1, single by triplet, is evaluated on
speeds variation. We recompute these data on the
following triplet and so on until the end of the file.
At the end of the operation we have a set of values
of coefficients [(p11, p12, p13), (p21, p22, p23), …,
(pn1, pn2, pn3)], speeds [(v11, v12), (v21, v21), … ,
(vn1, vn2)] and accelerations (a1, a2,…, an) for n
triplets representative of the piece of music. The
behaviour of swinging (position, speed and
acceleration) is obtained by a combination of the
average values and standard deviation of all these
data (pi, vi, ai).
The analysis of the behaviour of p (slope of the
line side of figure 2) will contribute to evaluate the
rhythmic behaviour by measuring the swinging of
the spectrum over one period, and in average value
on the various samples. By reference to mechanics,
this swinging, its speed and its acceleration are
evaluated as follows.
The first stage consists in identifying the number
of triplets as well as their position in the signal. On a
fraction of the sound file, we extract the first triplet
on which we calculate the 3 spectra then the
directing coefficients of the straight regression lines.
Thus 3 values of slope (p11, p12, p13) are obtained.
The speed of swinging is obtained by calculating the
difference between 2 consecutive slopes. We obtain
2 values of speed (v11, v12) for each triplet.
Acceleration a1, single by triplet, is evaluated on
speeds variation. We recompute these data on the
following triplet and so on until the end of the file.
At the end of the operation we have a set of values
of coefficients [(p11, p12, p13), (p21, p22, p23), …,
(pn1, pn2, pn3)], speeds [(v11, v12), (v21, v21), … ,
(vn1, vn2)] and accelerations (a1, a2,…, an) for n
triplets representative of the piece of music. The
behaviour of swinging (position, speed and
acceleration) is obtained by a combination of the
average values and standard deviation of all these
data (pi, vi, ai).
3 STATE OF THE ART
The process described in this document is
distinguishable from former work by a better
descriptive capacity compared to resources
necessary for calculation and storage. The
descriptive capacity is related to the rhythmic
evaluation by the analysis of the swinging structure.
These elements do not need to be obtained on the
whole sound file; a limited statistical sampling is
enough. The signature requires a priori only the
storage of a very limited quantity of numerical data.
In addition, the signature will be almost independent
of the format or the sound quality of the piece, even
if this last one is incomplete.
The existing techniques for the characterization
of musical files and research of similarities (MIR -
Music Information Retrieval) are various. There are
three principal approaches: those based on signal
processing, collaborative filtering, and data mining.
The approaches based on signal processing consist
in analyzing directly the content of the audio file
(signal and spectrum). In general, these
characteristics are modelled by learning systems,
and comparisons are carried out for the research of
similarities (McKay, 2004, Tzanetakis, 2001).
Another example of technology as regards acoustic
SIGMAP 2007 - International Conference on Signal Processing and Multimedia Applications
150