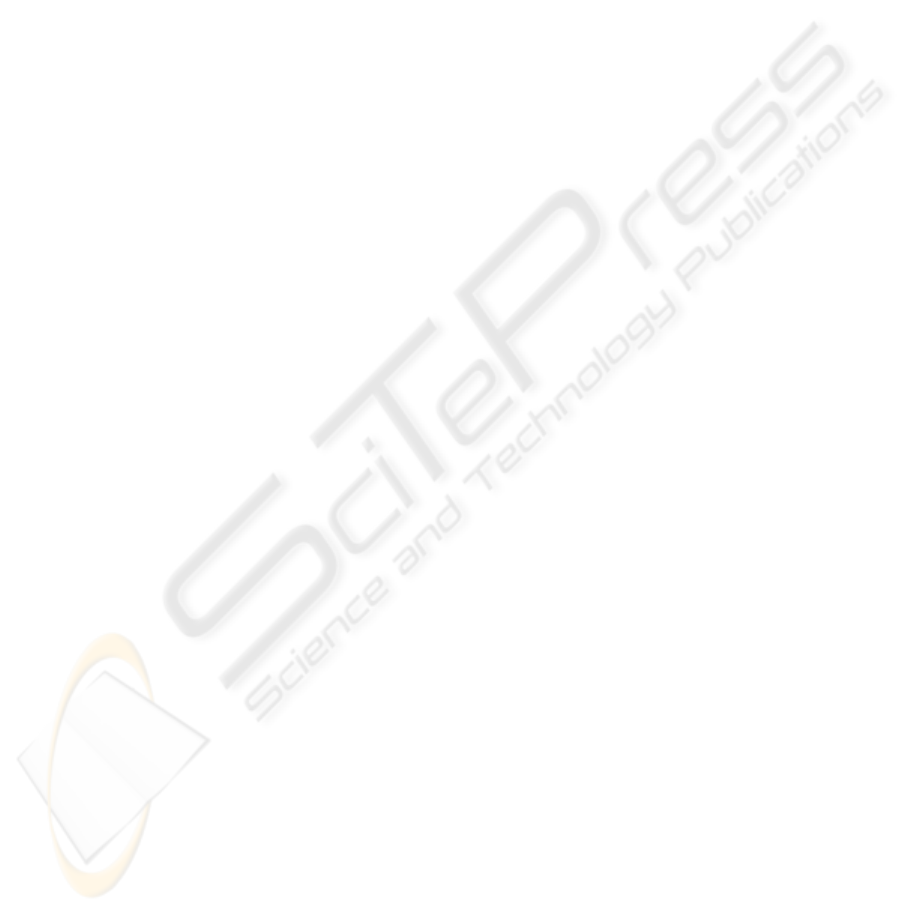
the selected fishes on the interface. Aqu@theque
brings to the visitor an additional dimension by
allowing him to become an actor instead of staying a
passive spectator.
In this paper, we addressed particularly the
problem of background subtraction which is the one
of the key steps in the system. First, we identified
the critical situations met in video and improved the
classification made by (Toyama, 1999).
This classification can be used in any application
which uses background subtraction like video
surveillance, motion capture or video games. In a
second step, we made a comparison between three
statistical background subtraction methods in the
context of video sequence acquired from aquatic
scenes.
A first qualitative evaluation showed that the
MOG is more efficient, without adding time to the
user’s request. Quantitative tests confirm that the
MOG enhance the percentage of detection. So, the
recognition was improved and the performance of
our interactive learning space too. In the future, we
can test more background subtraction methods and
make sophisticated evaluation using ROC Curves
and the PDR method developed by Kim (Kim, 2006)
on sequences test of the VSSN 2006 (VSSN, 2006).
Furthermore, the principle of Aqu@theque can
be used in any multimedia environments that need
this type of interaction. The background subtraction
method must be chosen according to the critical
situations met in the sequence used in the
application.
REFERENCES
Semani D., Saint-Jean C., Frélicot C., Bouwmans T.,
Courtellemont P., January 2002, Alive Fish species
characterization for on line video-based recognition,
Proceeding of the SPR 2002, Windsor, Canada, pages
689-698.
Semani D., Bouwmans T., Frélicot C., Courtellemont P.,
July 2002, Automatic Fish Recognition in Interactive
Live Videos, Proceeding of the IVRCIA 2002, volume
XIV, Orlando, Florida, pages 94-99.
Desfieux J., Mascarilla L., Courtellemont P., 2002,
Interactivity and Educational Information in Virtual
Real-Time 3D Videos, Proceeding of the IVRCIA
2002.
Wren C., Azarbayejani A., Darrell T., Pentland A., , July
1997, Pfinder : Real-Time Tracking of the Human
Body, IEEE Transactions on Pattern Analysis and
Machine Intelligence, Volume 19, No. 7, pages 780 –
785.
Stauffer C., 1999, Adaptive background mixture models
for real-time tracking, Proceedings IEEE Conference
on Computer Vision and Pattern Recognition, pages
246-252.
Elgammal A., Harwood D., Davis L., June 2000, Non-
parametric Model for Background Subtraction, 6th
European Conference on Computer Vision 2000,
Dublin, Ireland.
Toyama K., Krumm J., Brumitt B., 1999, Wallflower:
Principles and Practice of Background Maintenance,
Proceedings of the International Conference on
Computer Vision 1999, pages 255-261.
Cucchiara R., Grana C., Piccardi M., Prati A. , 2003,
Detecting Moving Objects, Ghosts and Shadows in
Video Streams", IEEE Transactions on Pattern
Analysis and Machine Intelligence 2003, volume 25,
no 10, pages 1337-1342, 2003.
Culibrk D., Socek D. , Marques O., Furht B., March 2007,
Automatic kernel width selection for neural network
based video object segmentation, VISAPP 2007,
Barcelona, Spain.
KaewTraKulPong P., Bowden R., September 2001, An
Improved Adaptive Background Mixture Model for
Real-time Tracking with Shadow Detection,
Proceedings 2nd European Workshop on Advanced
Video Based Surveillance Systems, AVBS 2001,
Kingston, UK.
Kim K., Chalidabhongse T., Harwood D., Davis L. , 2006,
PDR: Performance Evaluation Method for
Foreground-Background Segmentation Algorithms,
EURASIP Journal on Applied Signal Processing.
Huerta I., Rowe D., Gonzàlez J., Villanueva J., October
2006, Improving Foreground Detection for Adaptive
Background Segmentation, First CVC Internal
Workshop on the Progress of Research and
Development (CVCRD 2006).
VSSN 2006 - Algorithm Competition in
Foreground/Background Segmentation:
http://www.imagelab.ing.unimo.it/vssn06/.
SIGMAP 2007 - International Conference on Signal Processing and Multimedia Applications
158