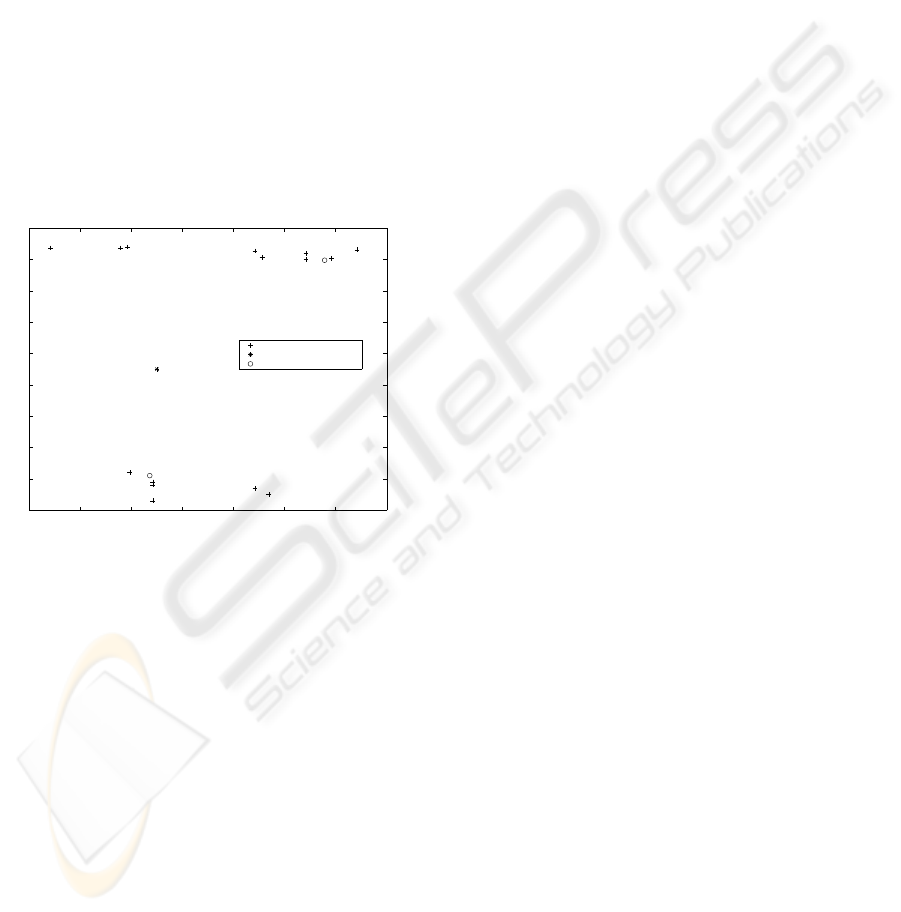
ima appear as time increases. In this paper we have
focussed the experience on a bi-dimensional represen-
tation (2-dimensional feature vectors) because we ob-
tain very intelligible 2-D graphs.
Fourth-order sliding cumulants exhibit clear dif-
ferences, not only for the shape of the computation
graph (the bottom graphs in Fig. 9, but also for the
different location of minima, which suggest a cluster-
ing zone for the points.
Fig. 10 presents the results of the training stage,
using the Kohonen rule. The horizontal (vertical) axis
corresponds to the maxima (minima) value. Each
cross in the diagram corresponds to an input vector
and the circles indicate the final location of the weight
vector (after learning) for the two neurons of the com-
petitive layer. Both weight vectors point to the aster-
isk, which is the initializing point (the midpoint of the
input intervals).
0.03 0.04 0.05 0.06 0.07 0.08 0.09 0.1
−9
−8
−7
−6
−5
−4
−3
−2
−1
0
x 10
−3
Cluster classification. 4th−order cumulant
cumulant maxima
cumulant minima
:measured vector
:initial neuron weight vector
:final neuron weight vector
Figure 10: Competitive layer training results over 20
epochs. Upper cluster: Short-duration PQ-events. Down
cluster: Long-duration event.
The separation between classes (inter-class dis-
tance) is well defined. Both types of PQ events are
horizontally clustered. The correct configuration of
the clusters is corroborated during the simulation of
the neural network, in which we have obtained an ap-
proximate classification accuracy of 97 percent. Dur-
ing the simulation new signals (randomly selected
from our data base) were processed using the method
described.
The accuracy of the classification method in-
creases with the number of data. To evaluate the con-
fidence of the statistics a significance test have been
conducted. This informs if the number of experiments
is statistically significant according to the fitness test
(
¨
Omer Nezih Gerek and Ece, 2006). As a result of
the test, the number of measurements is significatively
correct.
5 CONCLUSION
In this work we have reviewed computation of higher-
order statistics. Concretely we have focussed on a
4-order estimates. We have also proposed a method
to detect and classify two electrical power transients,
named short and long-duration. The method com-
prises two stages. The first includes pre-processing
(normalizing and filtering) and outputs the 2-D fea-
ture vectors, each of which coordinate corresponds to
the maximum and minimum of the central cumulants.
The second stage uses a neural network to classify the
signals into two clusters. This stage is different-in-
nature from the one used in (
¨
Omer Nezih Gerek and
Ece, 2006) consisting of quadratic classifiers. The
configuration of the clusters is assessed during the
simulation of the neural network, in which we have
obtained an acceptable classification accuracy.
ACKNOWLEDGEMENTS
The authors would like to thank the Spanish Min-
istry of Education and Science for funding the project
DPI2003-00878 which involves noise processes mod-
eling, and the PETRI project PTR95-0824-OP in-
volving higher-order statistics. Also thanks to the
Andalusian Government for the trust put in the re-
search group PAI-TIC-168, and for supporting the ex-
cellence project PAI2005-TIC00155, which involves
higher-order statistics.
REFERENCES
Hinich, M. J. (1990). Detecting a transient signal
by biespectral analysis. IEEE Trans. Acoustics,
38(9):1277–1283.
Jakubowski, J., Kwiatos, K., Chwaleba, A., and Osowski,
S. (2002). Higher order statistics and neural network
for tremor recognition. IEEE Trans. on Biomedical
Engineering, 49(2):152–159.
Mendel, J. M. (1991). Tutorial on higher-order statistics
(spectra) in signal processing and system theory: The-
oretical results and some applications. Proceedings of
the IEEE, 79(3):278–305.
Nandi, A. K. (1999). Blind Estimation using Higher-Order
Statistics, volume 1. Kluwer Academic Publichers,
Boston, 1 edition.
Nikias, C. L. and Petropulu, A. P. (1993). Higher-Order
Spectra Analysis. A Non-Linear Signal Processing
Framework. Englewood Cliffs, NJ, Prentice-Hall.
¨
Omer Nezih Gerek and Ece, D. G. (2006). Power-
quality event analysis using higher order cumulants
HIGHER-ORDER STATISTICS INTERPRETATION. APPLICATION TO POWER-QUALITY CHARACTERIZATION
77