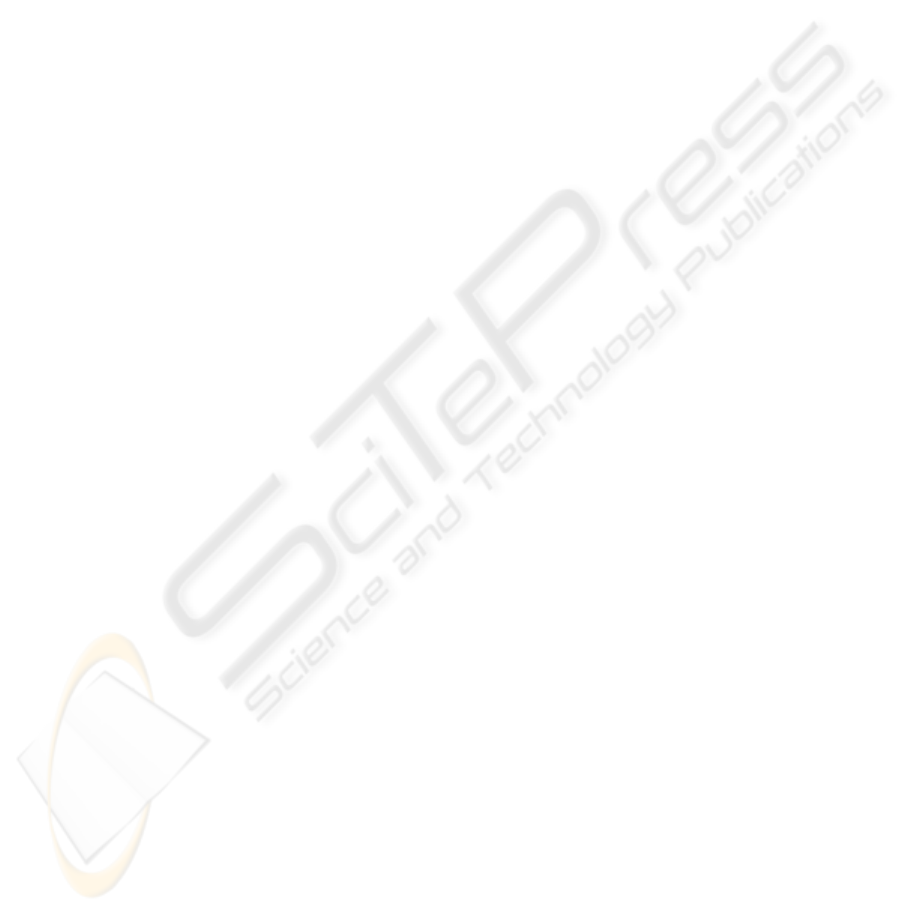
3.10 CIE-XYZ Model
The results obtained with this colour space are quite
similar to the obtained using RGB. However we can
notice that XYZ provides better results for channel
R (X in this case –87.44%–) than RGB format
(82.79%). So, we can say that XYZ colour space is a
bit more robust to illumination conditions than RGB.
4 CONCLUSIONS
We have done a complete study at pixel level of 10
different colour spaces using typical images in face
recognition systems. The purpose of this study was
to perform an objective comparison among the most
used colour spaces in skin detection to discover
which colour model provides the best results. We
can group the different colour spaces into 4 different
families: RGB family (RGB and CMY), YUV
family (YUV, YIQ, YCbCr, YPbPr, YCgCr,
YDbDr), HSV family and CIE family. According to
the obtained results, the most appropriate family for
skin detection is HSV (because HSV colour format
is the winner in our study). However, there is a
component in all colour models which, in general,
provides constant and positive results. This
component is Red component (the more significant
channel for skin detection).
We can also state that luminance channel (in
colour spaces where it is separated from
chrominance. –Y in almost all channels and V in
HSV–) is not a very important channel in skin colour
detection. In fact, we can also say that colour spaces
where luminance and chrominance are separated get
better results (RGB, CMY and XYZ colour spaces
have the lowest right detection rates of all models).
All in all, the 10 colour spaces that we have
studied provide quite good results in skin colour
detection. In general, all colour models have reduced
false positives and false negatives rates (peaks
values are explained by some unlucky highlights in
the face of some people), and the right detection
rates are at least over 86% in all colour spaces, so
we can conclude that all the models can be used for
skin colour detection with more or less success and
precision (this explains why in the bibliography
there are studies using such amount of different
colour spaces).
To sum up, after doing the quantitative study
described in this paper, we can conclude that HSV
colour space is the model which gets the best results
for skin colour detection. On the other hand, there
are colour spaces that obtain quite poor results, such
as CMY, CIE-XYZ, YIQ or even RGB. In any case,
it is possible to use almost any colour space to find
skin colour because with the appropriate classifier
and some pre-processing in the images (such as
giving higher values to contrast) most colour spaces
have quite high right detections rates.
ACKNOWLEDGEMENTS
This work has been developed in part thanks to the
OPLINK project (TIN2005-08818-C04-03). José M.
Chaves-González is supported by research grant
PRE06003 from Junta de Extremadura (Spain).
REFERENCES
Albiol, A., Torres, L., et al. Optimum Color Spaces for
Skin Detection. IEEE International Conference on
Image Processing. vol 1, pp: 122-124, Oct. 2001.
Campadelli, P., Lanzarotti, R., et al. Face and facial
feature localization. Int. Conf. on Image Analysis and
Processing. vol 1, pp: 1002-1009, Oct. 2005.
de Dios, J.J., Garcia, N. Fast face segmentation in
component color space. International Conference on
Image Processing. vol. 1, pp: 191-194. Oct. 2004.
Jinfeng Y., Zhouyu F., et al. Adaptive skin detection using
multiple cues. International Conference on Image
Processing. vol. 2, pp: 901-904. Oct. 2004.
Martinez, A.M., Benavente, R. The AR Face Database.
CVC Technical Report #24, June 1998.
Naseem, I., Deriche, M. Robust human face detection in
complex color images. IEEE International Conference
on Image Processing. vol. 2, pp: 338-341, Sept. 2005.
Phung, S.L., Bouzerdoum, A., et al. Skin segmentation
using color pixel classification: analysis and
comparison. IEEE Transactions on PAMI. vol. 27, No.
1, pp.: 148-154, Jan. 2005.
Pratt, W.K. Digital Image Processing: Pinks Inside. 3rd.
edition. John Wiley & Sons, 2001.
Runsheng J., Bin K., et al. Color Edge Detection Based on
YUV Space and Minimal Spanning Tree. IEEE
International Conference on Information Acquisition.
vol. 1, pp: 941 – 945, Aug. 2006.
Shapiro, L.G., Stockman, G.C. Computer Vision. Prentice-
Hall, 2001.
Sigal, L., Sclaroff, S., et al. Skin color-based video
segmentation under time-varying illumination. IEEE
Transactions on Pattern Analysis and Machine
Intelligence. vol. 26, No. 7, pp: 862 – 877, July 2004.
Yang, M-H., Kriegman, D.J., et al. Detecting Faces in
Images: A Survey. IEEE Transactions on PAMI, Vol.
24, No. 1, pp: 34-58, Jan. 2002.
Zhao, W., Chellappa, R., et al. Face Recognition: A
literature survey. ACM Computing Surveys, Vol. 35,
No. 4, pp: 399-458, Dec. 2003.
SIGMAP 2007 - International Conference on Signal Processing and Multimedia Applications
178