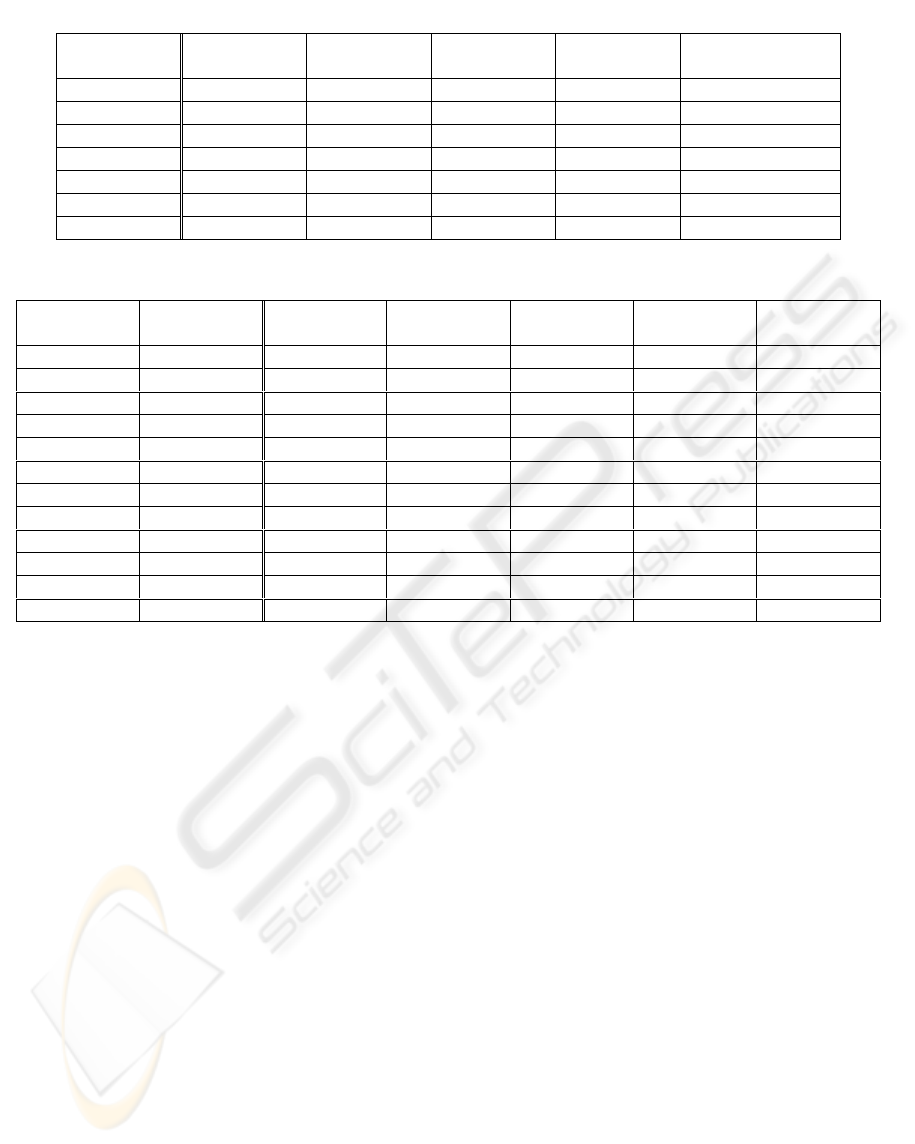
Although at this point there are more indications to
use 35 MFCCs to characterize the music signal, we
chose 40 for two reasons:
1. To make sure that the parameterisation of the
system is within the saturation zone of
information provided by the MFCCs.
2. Although this number of coefficients doesn’t
provide the best accuracy rate, it is the one that
gets minor substitution errors. This kind of error
goes down significantly by increasing the
MFCCs number.
7.3 Third Stage
It’s possible that the high insertion rates of the
system at this point, could be given by the different
temporary evolution of the notes played with
different instruments. This fact motivates the
following system tests, which consists of using
several window sizes with some different overlays
between them.
The window sizes used in the experiment
oscillate between 30 and 90 ms with overlays
between 50 and 80% of the window size.
Table 5 shows the experimental results. The
optimum point is produced using 60 ms windows
displaced by 12 ms. On the other hand, the
successful outcome of the results confirms the
validity of the HMMs topology for notes
recognition.
Figure 5 represents the percentage accuracy
evolution of the recognition system through the
successive parameterisation improvements made in
experiments.
Finally, we have to point out that accuracy rate
obtained by Durey’s system is 71.7% in multi-
instrument recognition conditions. This value is
lower than any one obtained by the proposed system
in any experiment in all the three stages (Table 5).
8 CONCLUSIONS
The present work shows a study on a suitable set of
features extracted from the signal to be used in
musical notes recognition. Likewise, Hidden
Markov Models have been shown to be powerful
enough when applied to musical notes recognition.
Table 4: Recognition and error rates varying the number of MFCCs.
Number of
MFCCs
% correct
notes
% deleted
notes
% substituted
notes
% inserted
notes
Percent Accuracy
14 85,16 1,96 12,88 3,79 81,37
20 92,49 1,75 5,77 4,29 88,19
25 95,14 1,50 3,36 4,66 90,48
30 96,72 1,70 1,58 4,73 91,99
35 97,39 1,97 0,64 4,93 92,46
40 97,43 2,10 0,46 5,84 91,60
45 97,27 2,18 0,55 6,05 91,22
Table 5: Recognition and error rates varying the windows width and its overlapping.
Window
Width (ms)
Overlapping
(ms)
% correct
notes
% deleted
notes
% substituted
notes
% inserted
notes
Percent
Accuracy
30 6 98,23 1,55 0,22 4,95 93,28
30 7,5 98,29 1,54 0,17 6,86 91,43
30 10 98,16 1,62 0,22 8,31 89,85
30 15 96,49 3,12 0,39 3,59 92,97
60 12 98,43 1,25 0,32 0,17 98,26
60 15 98,11 1,65 0,24 0,05 98,06
60 20 97,16 2,61 0,23 0,01 97,15
60 30 95,94 3,63 0,42 0 95,95
90 18 97,90 1,91 0,19 0,04 97,86
90 22 97,10 2,65 0,25 0 97,10
90 30 96,10 3,61 0,30 0,01 96,08
90 45 94,14 5,49 0,37 0 94,14
SIGMAP 2007 - International Conference on Signal Processing and Multimedia Applications
190