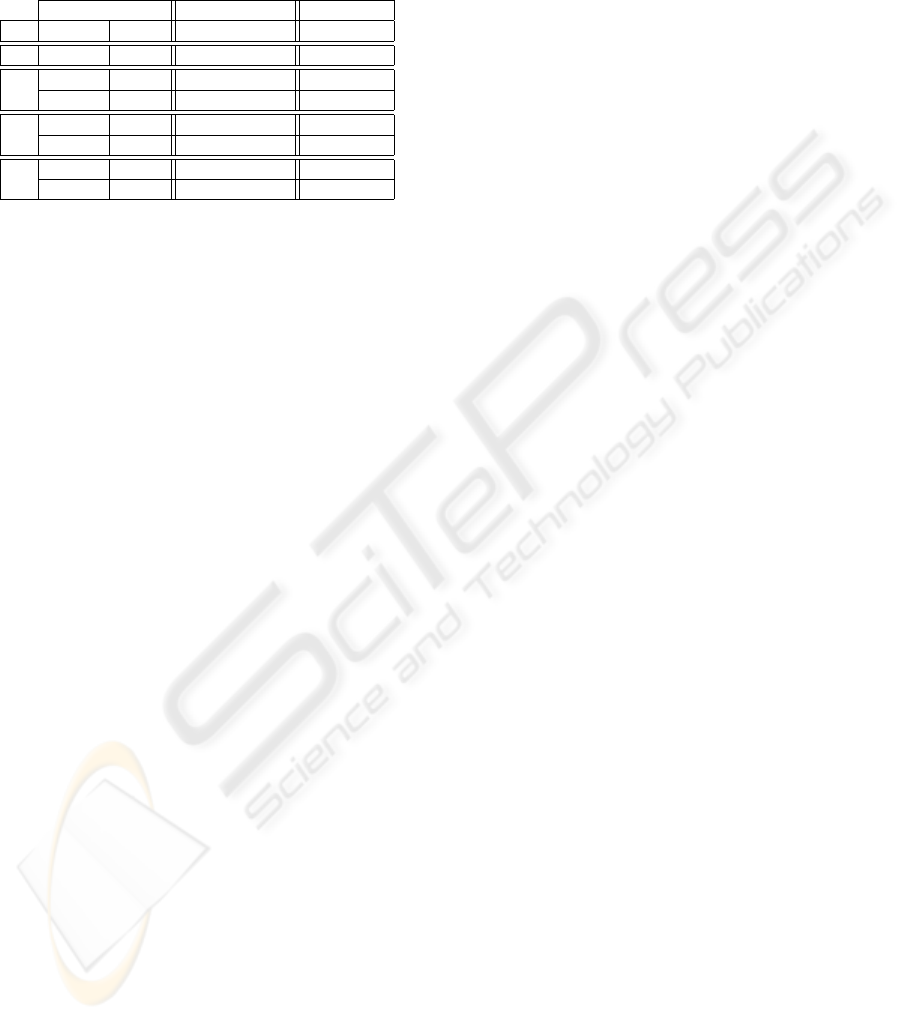
Table 2: Usual criteria values for non-regularized and reg-
ularized
b
f obtained from
d
h.∗f from degraded [LACORNOU]
image (defocusing blur d = 9, and additive gaussian noise
with various standard deviation levels σ
n
=0, 8, 12 and 16)
filtered by filter of Chan et al.
d
h∗ f
non-regularized
b
f regularized
b
f
σ
n
iteration PSNR PSNR PSNR
0 0 Inf 30.861 23.907
8
0 30.046 10.952 23.101
3 39.526 23.663 23.534
12
0 26.566 8.790 22.219
4 37.127 22.709 23.302
16
0 24.134 7.837 21.255
5 35.495 22.005 23.062
3 CONCLUSION AND FUTURE
WORK
Both the numerical performance and visual evalua-
tion of the results obtained by the proposed restora-
tion method are significantly more favorable than that
of the classical method without preliminary noise re-
duction. The numerical results indicate that the pro-
posed scheme is quite robust and the image f can be
recovered under presence of the noise even if it is high
level. The quality of the restored image depends vig-
orously on the estimation of h∗ f, which means, the
choice of the filter and the number of iteration are
two important factors to get the satisfying results. We
have evaluated a finite set of representative filtering
methods in order to select the optimal one. The filter
of Chan et al. achieves the best compromise between
the quality of the filtering result and the number of
iterations involved.
Primary results based on the estimation of the
standard deviation of the filtering residuals are en-
couraging to solve the problem of the determination
of the optimal iteration number of filtering.
ACKNOWLEDGEMENTS
Supported by the European Union. Co-financed
by the ERDF and the Regional Council of Brittany
through the Interreg3B project number 190 PIMHAI.
REFERENCES
Biemond, J., Lagendijk, R. L., and Mersereau, R. M.
(1990). Iterative methods for image deblurring. Pro-
ceedings of the IEEE, 78(5):856–883.
Bronstein, M. M., Bronstein, A. M., Zibulevsky, M., and
Zeevi, Y. Y. (2005). Blind deconvolution of images
using optimal sparse representations. IEEE Transac-
tions on Image Processing, 14(6):726–736.
Chan, T., Osher, S., and Shen, J. (2001). The digital TV
filter and nonlinear denoising. IEEE Transactions on
Image Processing, 10(2):231–241.
Chantas, G. K., Galatsanos, N. P., and Likas, A. (2006).
Bayesian restoration using a new nonstationary edge-
preserving image prior. IEEE Transactions on Image
Processing, 15(10):2987–2997.
Jalobeanu, A., Blanc-Feraud, L., and Zerubia, J. (2002).
Hyperparameter estimation for satellite image restora-
tion using a MCMC maximum-likelihood method.
Pattern Recognition, 35(2):341–352.
Klaine, L. (2004). Filtrage et restauration myopes des im-
ages num
´
eriques. PhD thesis, Universit
´
e de Rennes 1,
France.
Koenderink, J. (1984). The structure of images. Biological
Cybernetics, 50(5):363–370.
Lee, J. (1980). Digital image enhancement and noise fil-
tering by use of local statistics. IEEE Transactions
on Pattern Analysis and Machine Intelligence (PAMI),
2(2):165–168.
Likas, A. C. and Galatsanos, N. P. (2004). A Variational
Approach for Bayesian Blind Image Deconvolution.
IEEE Transactions on Image Processing, 52(8):2222–
2233.
Mohammad-Djafari, A. (1996). Joint estimation of param-
eters and hyperparameters in a Bayesian approach of
solving inverse problems. International Conference
on Image Processing, Lausanne, Switzerland, 1:473–
476.
Molina, R., Mateos, J., and Katsaggelos, A. K. (2006).
Blind Deconvolution Using a Variational Approach to
Parameter, Image, and Blur Estimation. IEEE Trans-
actions on Image Processing, 15(12):3715–3727.
Molina, R., Mateos, J., Katsaggelos, A. K., and Vega, M.
(2003). Bayesian multichannel image restoration us-
ing compound Gauss-Markov random fields. IEEE
Transactions on Image Processing, 12(12):1642–
1654.
Nikolova, M., Idier, J., and Mohammad-Djafari, A. (1998).
Inversion of large-support ill-posed linear operators
using apiecewise Gaussian MRF. IEEE Transactions
on Image Processing, 7(4):571–585.
Park, S. C. and Kang, M. G. (2006). Noise-adaptive
edge-preserving image restoration algorithm. Optical
Engineering (Bellingham, Washington), 39(12):3124–
3137.
Perona, P. and Malik, J. (1990). Scale-space and edge de-
tection using anisotropic diffusion. IEEE Transac-
tions on Pattern Analysis and Machine Intelligence,
12(7):629–639.
Rudin, L., Osher, S., and Fatemi, E. (1992). Nonlinear total
variation based noise removal algorithms. Physica D,
60(1-4):259–268.
IMAGE RESTORATION - A New Explicit Approach in Filtering and Restoration of Digital Images
203