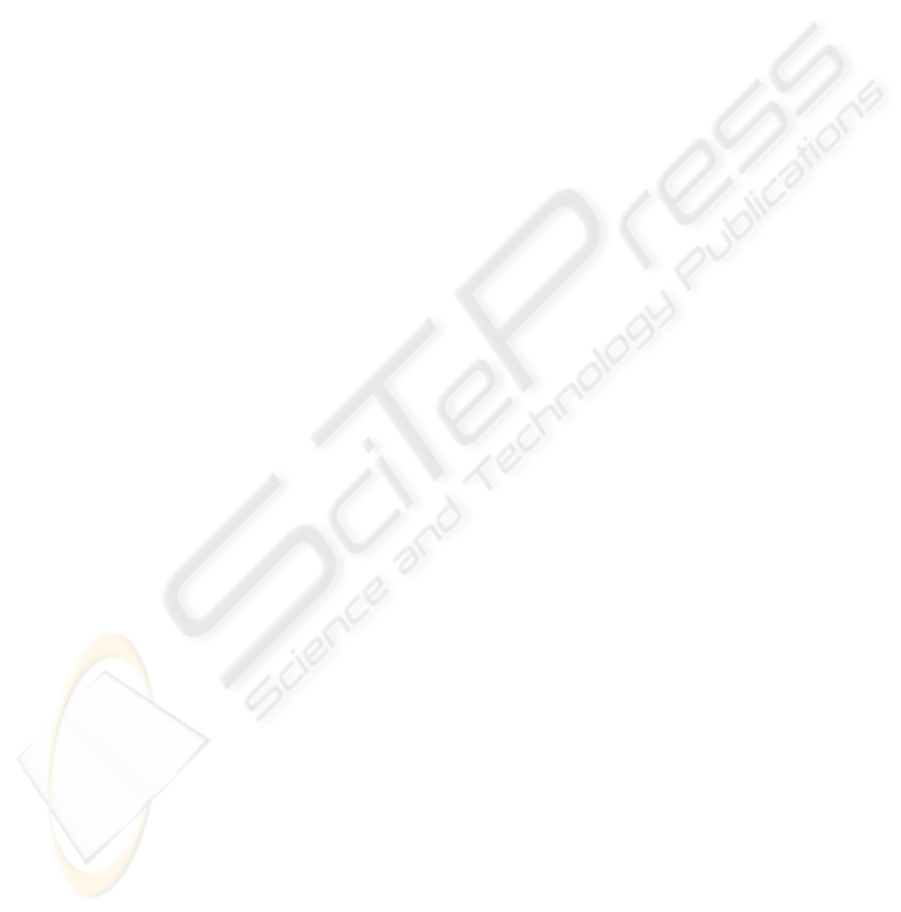
In Fig. 4 we compare this results in two query scenar-
ios: single image (Image ROC) and multi-image (Per-
son ROC) and two lighting scenarios: unconstrained
(upper part ROCs) and neutral (lower part ROCs).
In case of Image ROC, we observe the improve-
ment of Equal Error Rate EER for the neutral lighting
for about two times. The insignificant improvement
in case of Person ROC is justified by small number of
neutral photos for most of persons engaged in training
and testing.
4 DISCUSSION AND
CONCLUSIONS
Impact of light conditions on face verification are con-
sidered for three linear discriminant feature extraction
schemes. Two verification scenarios, the single image
query and multi image query, were compared. The ex-
traction algorithms are based on compositions of fea-
ture projections on global, intra and inter-class error
subspaces: Linear Discriminant Analysis LDA, Dual
Linear Discriminant Analysis DLDA, and their com-
bination LDA+DLDA.
The metrics for evaluation of the verification er-
ror is the Mahalanobis distance between normalized
feature vectors. The normalization of feature vectors
is justified with the upper bound by Fisher separation
index for feature vectors.
Experiments conducted on facial databases with
complex background show the high performance of
DLDA and DLDA+LDA verifiers with Equal Error
Rate EER less than one percent. The degradation of
results when controlled light conditions are replaced
by uncontrolled ones is of factor two.
In cash machine application the input of verifica-
tion system is given as temporal sequence of images.
On the basis of the previous works we recommend
the design of face verifier for this application by the
following six steps:
1. Detect frontal pose of face by Discrete Gabor Jet
DGJ algorithm w.r.t. inner eye and nose corners
(Skarbek W. and Naruniec J., 2007).
2. Compensate lighting by Quotient Illumination
Relighting algorithm (QIR) (Cao B., Shan S., Gao
W. and D. Zhao, 2003).
3. Compensate pose by inner eye and mouth corners
to find the homographic mapping.
4. Align the compensated image by alignment of the
line segment joining outer eye corners.
5. Design the feature extraction scheme by optimiz-
ing DAD diagram.
6. If the verifier of 5 is not satisfactory then optimize
DLDA cascade.
ACKNOWLEDGEMENTS
The work presented was developed within VIS-
NET 2, a European Network of Excellence
(http://www.visnet-noe.org), funded under the
European Commission IST FP6 Programme.
REFERENCES
Fukunaga, K. (1992). Introduction to Statistical Pattern
Recognition. Academic Press.
Golub, G. and Loan, C. (1989). Matrix Computations. The
Johns Hopkins University Press.
Jain, A. K., Bolle, R., and Pankanti, S., editors (1999). Bio-
metrics: Personal Identification in Networked Society.
Kluwer Academic Publishers.
Cao B., Shan S., Gao W. and D. Zhao, (2003). Illumina-
tion normalization for robust face recognition against
varying lighting conditions in . IEEE ICCV-2003,
157:164, 2003
MPEG-7, editor (2004). Multimedia content description in-
terface. Visual Descriptor Extensions., volume 15938-
3:2002/Amd.1:2004. ISO/IEC.
Skarbek W., Kucharski K. and M. Bober, (2004). Dual LDA
for Face Recognition in . Fundamenta Informaticae
303:334:2004
Skarbek W., Kucharski K. and Bober M. , (2006). Cas-
cade of dual LDA operators for face recognition in .
Geometric properties from incomplete data, Kluwer
Academic Publishers 199:219, 2006
Leszczy
´
nski M. and Skarbek W., (2007). Biometric Verifi-
cation by Projections in Error Subspaces in . Rough
Set and Knowledge Technology, Springer LNAI 4481,
166:174, 2007
Naruniec J. and Skarbek W., (2007). Face Detection by
Discrete Gabor Jets and Reference Graph of Fiducial
Points in . Rough Set and Knowledge Technology,
Springer LNAI 4481, 187:195, 2007
FACE VERIFICATION IN UNCONTROLLED LIGHT CONDITIONS OF STREET
431