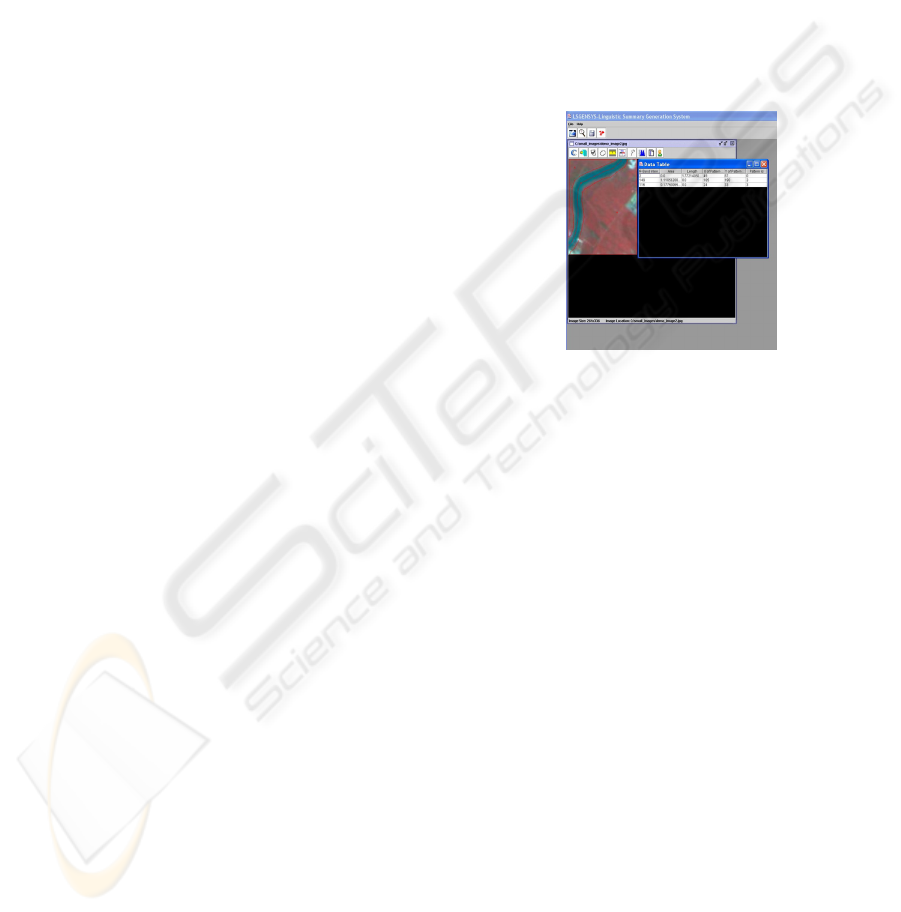
the linguistic summaries or descriptions of all
objects in the table is
f=max(T), (5)
where T is evaluated as shown in the previous
section.
4.2 Results and Discussion
Image objects/patterns are classified at the highest
level into land, water or fire. Land is further
classified into island and other land. Water is further
classified into river and other water body. The fuzzy
sets that quantify area or length are defined with
reference to geographic facts such as:
• Largest continent is Asia with area of
44579000 km
2
• Largest freshwater lake is Lake Superior
with area of 82103 km
2
• Smallest continent is Australia/Oceania
with area of 7687000 km
2
In (6), the triangular fuzzy set for considerably
large expanse of water is shown.
waterof expanse largely considerab
(x)=
1-(55068.66-x)/27034.33, for
28034.33≤x≤55068.66
=1-(x-55068.66)/27034.33, for 55068.66≤x≤
82103
=0, x< 28034.33
=0, x> 82103 (6)
An example SPOT MS satellite image to be
analysed is shown in Figure 2. Figure 2 shows the
image analysis tool of the system as it extracts area
and length attributes of three patterns in the image.
These attributes are calculated and displayed in the
data table in the figure. Pattern id attribute denotes
numbers as follows: 0= River, 1=Water Body,
2=Island, 3=Land, 4=Fire. Location is indicated by
X, Y pixel co-ordinates of centroid of pattern/object.
For river, its length is the most significant attribute
for calculation, whereas for all other patterns, area is
the most significant attribute for calculation.
The user may choose, at this stage, to interact with
the system and suggest some possible summaries.
The system can evaluate the fitness of the user-
summaries and inform the user if they are most
suitable to describe the image patterns in the table.
After the user chooses triangular fuzzy sets in the
knowledge base, the system displays triangular
fuzzy set definitions (lower-left window in Figure
3) in order to guide the user to select the appropriate
fuzzy set for area or for length of different patterns
such as land, island, water body, river etc. With the
aid of these fuzzy set definitions, the user then
constructs some possible pattern summaries as
shown in Window 1. The fitness value (1.0) of these
possible summaries is calculated and displayed in
Window 1. Thereafter, the user may verify the
correctness of these summaries and their suitability
with respect to the image patterns by running the GA
Inference Engine.
Figure 4 shows a snapshot of the GA Inference
Engine as it runs (in the top left window) and
evolves the most suitable summaries with higher
fitness, over several generations.
Figure 2: The image analysis tool of the system as it
extracts area and length of patterns in a SPOT MS image.
Approximate scale of image 1: 0.000019.
The summaries generated by the engine are
captured in a text file as displayed in the top right
window of Figure 4. The user may also chose to
bypass the summary construction process, and
directly invoke the inference engine (by pressing the
Run button in Figure 3) to construct and generate the
most suitable summaries. This would resemble
unsupervised classification and description.
The GA is run with following input parameter
set. These parameter values are set after several trial
runs.
No: of bits in a chromosome string of the
population = 10
Generations per cycle = 27
Population size = 200 strings
Probability of cross-over = 0.535
Probability of mutation = 0.001
After 216 generations, the linguistic summaries
generated for the data in Table 1 are:
• A short river at the top left
• A small area of land at the top left
• A small area of land at the right
Thus, the possible summaries formulated by the
user compare well with the summaries generated by
the inference engine.
ICEIS 2007 - International Conference on Enterprise Information Systems
480