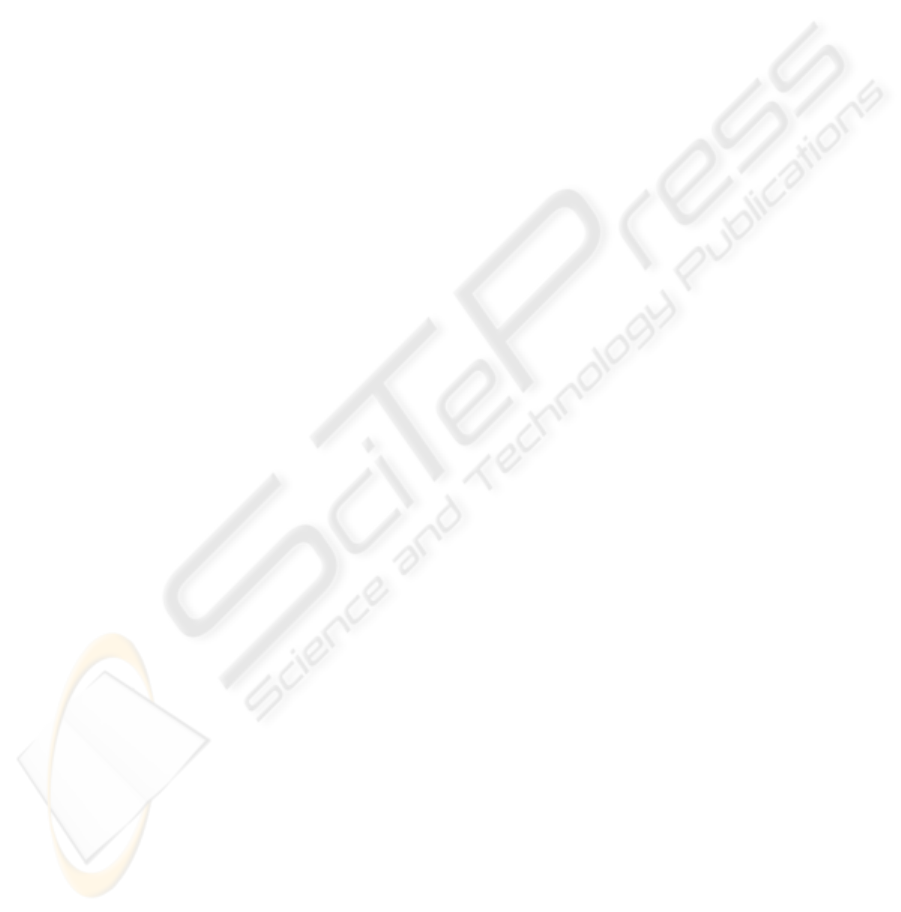
objective. Note that although we are able to compare
two axioms structurally, we have not yet considered
the semantic mapping between axioms. Another way
to categorize the tools is to consider the type of in-
put on which the tool relies in its analysis and which
it requires: (1) class names or natural-language defi-
nitions, (2) class hierarchy and properties, or (3) in-
stances. Our approach is based on (2) and (4); we
also introduces a new type of input: Axioms (includ-
ing Schemata Axioms and Domain Axioms).
Then, in (Ehrig and Sure, 2004), a similarity stack
is provided in order to classify the different measures
that can be used to perform ontology matching. This
stack is composed of five levels: the Entities level,
the Semantic Nets level, the Description Logics level,
the Restrictions level and the Rules level. For the first
three levels, the authors provide similarity measures
which of course differ according to semantic com-
plexity of the level which is considered. However, for
the Restrictions level and Rules level, no measure is
proposed. Explanations given by the authors are the
following: ”the features like algebraic properties or
equivalence/disjointness are not sufficiently used by
the community to be considered as a material for sim-
ilarity measure; for the Rules level, there has not been
sufficient research and practical support for the Rule
Layer of the Semantic Web Layer Cake”. Our work
must be considered as an extension of this classifica-
tion in the sense that it provides measures based on
the axioms of the domain which include both the Re-
strictions level and the Rules level. However, as we
claim that it is not possible to consider rules and con-
straints at the ontological level (rules and constraints
only exist at the operational level, we propose to mod-
ify the stack by merging the two levels Restrictions
and Rules into only one: the Axioms level.
5 CONCLUSION
In this paper, we have introduced a new ontology
matching approach. This approach, which mainly re-
lies on graph-based representations and graph-based
reasoning mechanisms, is particularly relevant to
manage heavyweight ontologies since the main com-
ponents of these ontologies are axioms which can
be easily represented and compared with graph-based
solutions. Our method has the advantage of incor-
porating most of the descriptive features of a heavy-
weight ontology into the matching process whereas
most of the current methods cover only subsets of a
lightweight ontology (mainly the hierarchy of con-
cepts and their natural language expression). Of
course, this method, although applicable, is not very
efficient in a context of lightweight ontologies (and
this is why we are not yet involved in the OAEI cam-
paigns) . However, as demonstrated by the current
challenge ”Reasoning the Semantic Web”, the need
for developing heavyweight ontologies inevitably will
increase in an immediate future. So, it seems interest-
ing to focus on developing matching techniques dedi-
cated to this type of ontology.
REFERENCES
Ashpole, B., Ehrig, M., Euzenat, J., and Stuckenschmidt,
H. (2005). Ontology alignment evaluation initiative -
2005. In Proceedings of the K-CAP 2005 Workshop on
Integrating Ontologies. CEUR Proceedings - Volume
156.
Benjamins, R., Euzenat, J., Noy, N., Shvaiko, P., Stuck-
enschmidt, H., and Uschold, M. (2006). Ontology
alignment evaluation initiative - 2006. In Proceedings
of the International Workshop on Ontology Matching,
http://oaei.ontologymatching.org/2006/.
Berners-Lee, T., Handler, J., and Lassila, O. (2001). The
semantic web. In Scientific American, volume 248,
pages 35–43.
Doan, A. and Halevy, A. (2005). Semantic integration re-
search in the database community: A brief survey. In
AI Magazine, Special Issue on Semantic Integration.
Ehrig, M. and Sure, Y. (2004). Ontology Mapping - an inte-
grated approach. In Proceedings of the First European
Semantic Web Symposium, pages 76–91. Springer-
Verlag (LNCS 3053).
F
¨
urst, F., Lecl
`
ere, M., and Trichet, F. (2004). Operational-
izing domain ontologies: a method and a tool. In
de Mantaras, R. L. and Saitta, L., editors, European
Conference on Artificial Intelligence (ECAI’2004),
pages 318–322. IOS Press.
F
¨
urst, F. and Trichet, F. (2005a). Integrating domain on-
tologies into KBS. In 18th International Florida
Artificial Intelligence Research Society Conference
(FLAIRS’2005), pages 826–827. AAAI Press.
F
¨
urst, F. and Trichet, F. (2005b). Toocom: bridge the
gap between ontologies and knowledge-based sys-
tems. In 17th International Conference on Knowledge
Engineering and Software Engineering (SEKE’2005),
pages 235–243. KSI editors.
Gomez-Perez, A., Fernandez-Lopez, M., and Corcho, O.
(2003). Ontological Engineering. Springer, Advanced
Information and Knowledge Processing.
Noy, N. F. (2004). Semantic integration: A survey
of ontology-based approaches. SIGMOD Record,
33(4):65–70.
Shvaiko, P. and Euzenat, J. (2005). A survey of schema-
based matching approaches. In Journal on Data Se-
mantics (3730), pages 76–171. Springer-Verlag.
Sowa, J. (1984). Conceptual Structures : information pro-
cessing in mind and machine. Addison-Wesley.
ICEIS 2007 - International Conference on Enterprise Information Systems
270