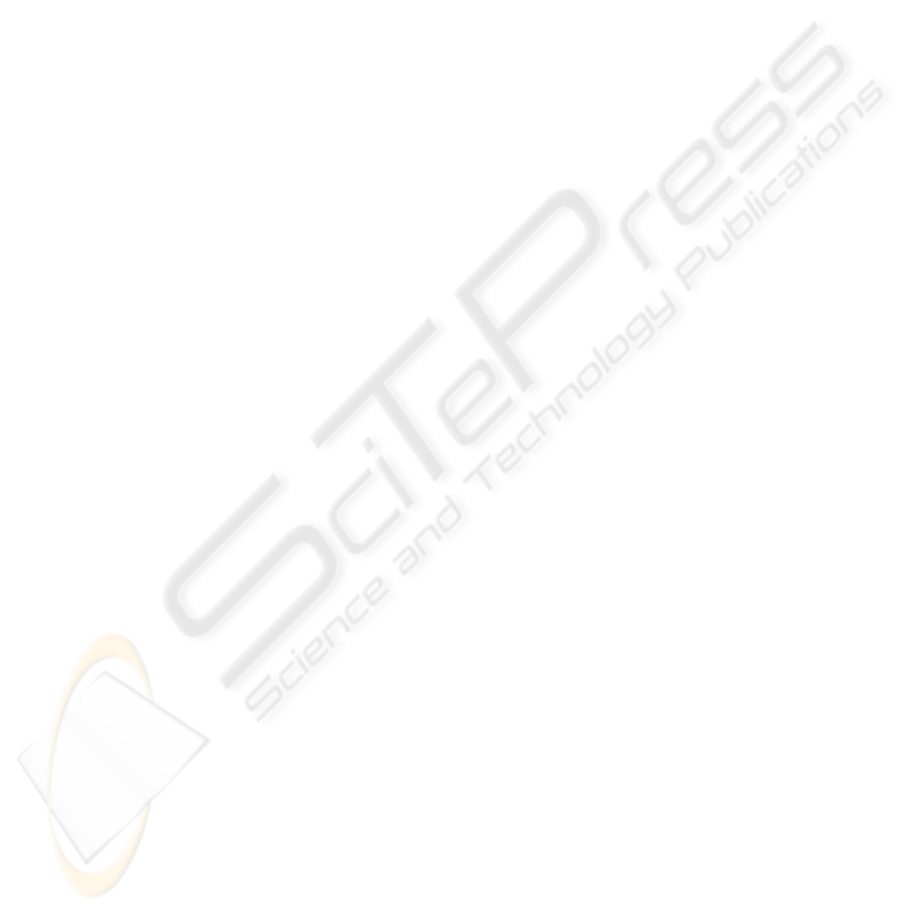
their product evaluation ascribe most importance to
those attribute beliefs which are closer in line with
their internal affective state.
From a technical point of view, we opted for an
alternative methodology in using the OWA-operator.
In our opinion, this is a useful approach while the in-
terpretation of the OWA-weights is more straightfor-
ward compared to the more complex LISREL-models
as they have been traditionally used for instance by
Han (1988). An additional advantage lies in the fact
that the ‘orness’ gives us the needed quantification of
the optimistic degree of an evaluation. This aspect is
already a huge advantage of the fuzzy set approach
compared to the more traditional LISREL approaches
where this degree of optimism cannot be extracted
from the data. Finally, we introduced a bootstrap pro-
cedure to estimate the orness and the level of uncer-
tainty around it. This enables us to construct confi-
dence intervals and conduct hypothesis tests. As far
as we know, estimating this degree of uncertainty of
the orness has never been introduced in the literature
before.
4 CONCLUSION
Within the fuzzy set field, past application-oriented
research has mainly been focused on AGOP’s domain
representation power or decision-making strength. As
far as we know, the AGOP’s characteristics them-
selves have not been of any significant importance in
this particular type of research.
However, this paper has demonstrated that the
value of certain characteristics for applied research
should not be disregarded. It is illustrated that ag-
gregation operator characteristics carry the potential
of functioning as valid proxies for domain specific
knowledge, which is hard to measure directly or to
derive statistically from the data.
The presence of such potential has been proven to
exist by means of two marketing case-studies. The
first case-study examined the use of the uninorm in
customer satisfaction theory. It could be established
that the uninorm’s neutral value is a proxy for cus-
tomers’ expectations. This approach in turn pro-
vides the manager with new and important informa-
tion about the company’s performance. The second
case-study has explored the value of the OWA oper-
ator for country-of-origin research. The orness was
found to be suitable for the quantification of the cus-
tomers’ degree of optimism (pessimism) during the
process of product evaluation. Such method for quan-
tification in itself already counts as a technical con-
tribution toward the coo-field. In addition, managers
have gained more insight into the precise role of coo-
related feelings. As such, they are capable now of
dealing more effectively with this particular market-
ing phenomenon.
The validity of both operator characteristics as do-
main specific proxies has been verified theoretically
as well as empirically, which adds indirectly to the
value of our findings.
REFERENCES
Anderson, E. W., Fornell, C., and Lehman, D. (1994).
Customer satisfaction, market share and profitability:
Findings from sweden. Journal of Marketing, 58:63–
66.
Anderson, E. W., Fornell, C., and Mazvancheryl, S. K.
(2004). Customer satisfaction and shareholder value.
Journal of Marketing, 68:172–185.
Bilkey, W. and Nes, E. (1982). Country-of-origin effects on
product evaluations. Journal of International Business
Studies, (Spring/Summer):89–99.
Brijs, K., Vanhoof, K., Brijs, T., and Karlis, D. (2006). Us-
ing fuzzy set theory to assess country-of-origin effects
on te formation of product attitude. In Torra, V., edi-
tor, Proc. MDAI’06, volume 3885 of Lecture Notes in
Artificial Intelligence, pages 138–149.
Cacioppo, J. and Petty, R. (1989). The elaboration likeli-
hood model: The role of affect and affect-laden in-
formation processing in persuasion. In (Cafferata and
Tybout, 1989), pages 69–89.
Cadotte, E. R., Woodruff, R. B., and Jenkins, R. L. (1987).
Expectations and norms in models of consumer satis-
faction. Journal of Marketing Research, 24:305–314.
Cafferata, P. and Tybout, A., editors (1989). Cognitive and
Affective Responses to Advertising. Lexington Books,
Toronto.
Dombi, J. (1982). Basic concepts for the theory of evalua-
tion: The aggregative operator. European Journal of
Operational Research, 10:282–293.
Dubois, D. and Prade, H. (2004). On the use of aggregation
operations in information fusion processes. Fuzzy Sets
and Systems, 142:143–161.
Eagly, A. and Chaiken, S. (1993). The Psychology of Atti-
tudes. Harcourt Brace Jovanovich, Forth Worth, TX.
Efron, B. and Tibshirani, R. (1993). An Introduction to the
Bootstrap. Chapman & Hall.
Filev, D. and Yager, R. (1998). On the issue of obtain-
ing OWA operator weights. Fuzzy Sets and Systems,
94:157–169.
Fishbein, M. and Ajzen, I. (1975). Belief, Attitude, Inten-
tion and Behavior: An Introduction to Theory and Re-
search. Addison-Wesley.
Fodor, J. C., Yager, R. R., and Rybalov, A. (1997). Structure
of uninorms. International Journal of Uncertainty,
Fuzziness and Knowledge-Based Systems, 5:411–427.
THE IMPORTANCE OF AGGREGATION OPERATOR CHARACTERISTICS IN MARKETING RESEARCH
245