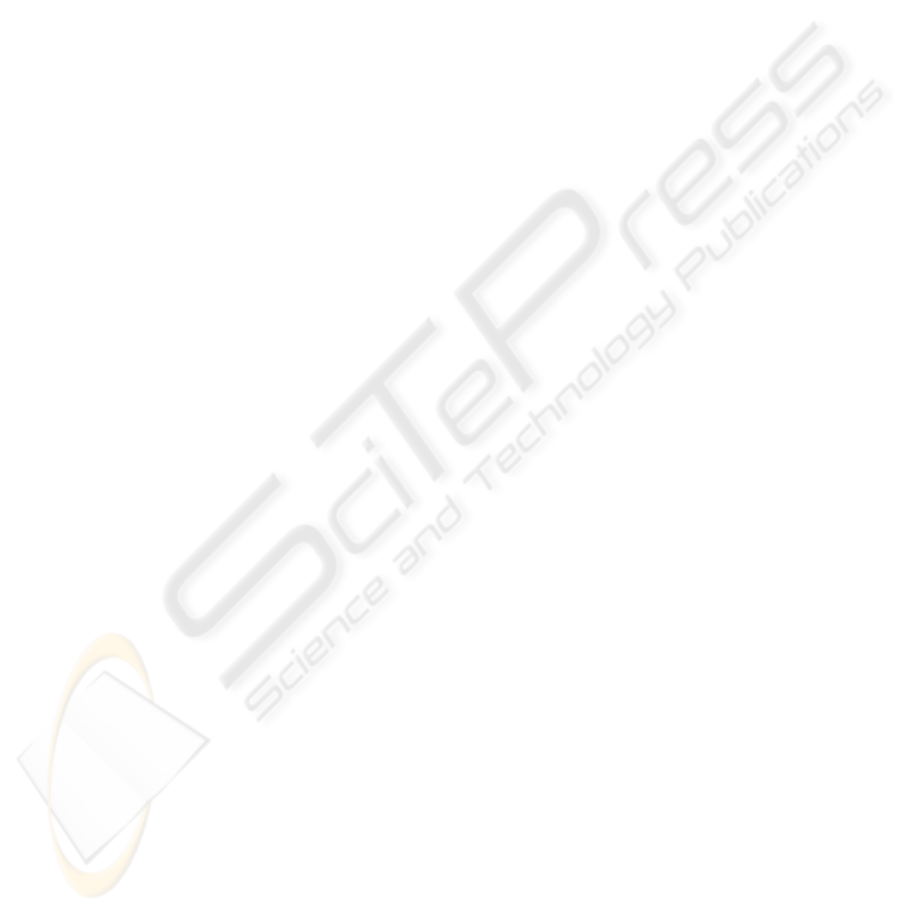
4 CONCLUSIONS AND FUTURE
WORK
One interesting conclusion was obtained from the
optimization process, and this is that the most
elaborate and complex strategies, and therefore those
with the greatest number of parameters, obtained
excellent values in optimisation but disastrous ones
in the test. On the other hand, while more basic and
simpler strategies with a lower number of
parameters did not perform particularly well in
optimisation they did in tests. This is due to the fact
that very complex strategies are capable of perfectly
adjusting to the particular characteristics of the
optimisation period, but overlearn and do not know
how to act when the conditions change. However,
simpler strategies abstract and generalise better and
their behaviour is similar in both optimisation and
test periods.
The extracted data are extremely valuable since
they can be used to carry out a large number of
scientific studies. A first study would consist in
representing the previous data in a data warehouse
and applying different data mining techniques in
order to extract patterns between the different
variables. Taking into account that the values of the
variables can easily be transformed into fuzzy
variables by means of linguistic labels, it would be
possible to carry out a similar study to the previous
one by using a fuzzy data warehouse capable of
extracting fuzzy association rules (Delgado, 2007).
Similarly, it would be advisable to find the results
with the greatest significant interest (Shekar, 2004).
The portfolio selection problem (Schlottmann
2004) can also be studied by using the methodology
in this work. This problem consists in looking for
the combination of strategies which, by acting
jointly, increase profits and reduce risk. This is a
typical multiobjective optimisation problem. The
data can also be studied with clustering techniques
which search for the groupings between strategies or
variables.
It would also be interesting to devise an expert
system for stock market investment, and one
possibility might be to achieve an expert assessment
of each strategy. By applying neural networks or
some other artificial intelligence technique, expert
knowledge could then be abstracted and represented
for implementation on the expert system’s
knowledge base.
The information obtained is subject to changes
according to time. An analysis could be carried out
to search for values which have undergone changes,
thereby obtaining new knowledge and eliminating
part of the previous knowledge (Chen, 2005).
REFERENCES
Baba, N., Inoue, N. et al, 2002. Utilization of Soft
Computing Techniques for Constructing Reliable
Decision Support Systems for Dealing Stocks. In
IJCNN’02: Proceedings of the 2002 International
Joint Conference on Neural Networks, Honolulu,
Hawaii.
Chapman, A.J., 1994. Stock Market Trading Systems
Through Neural Networks: Developing a Model.
International Journal of Applied Expert Systems, Vol.
2, no. 2, 1994, pages 88-100.
Chen, M., Chiu, A., Chang, H., 2005. Mining changes in
customer behaviour in retail marketing. Expert
Systems with Applications, 28, 773-781.
Delgado Calvo-Flores, M., Gibaja Galindo, E., Molina
Fernández, C., Nuñez Negrillo, J., 2007. Using Fuzzy
DataCubes in the Study of Trading Strategies. ICEIS
2007: International Conference on Enterprise
Information Systems, Funchal, Madeira – Portugal.
Fung, W., Hsieh, D., 1997. Empirical characteristics of
dynamic trading strategies: The case of hedge funds.
Review of Financial Studies, 10, 275-302.
Goldberg, D.E., 1989. Genetic Algorithms in Search,
Optimization, and Machine Learning. Addison-
Wesley. New York, USA.
Holland, J.H., 1975. Adaptation in Natural and Artificial
Systems. Ann Arbor, MI/USA: Mich. Univ. Press.
Liu, N. K., Lee, K. K., 1997. An Intelligent Business
Advisor System for Stock Investment. Expert Systems
14(3): 129-139.
Schittenkopf, C., Tino, P., Dorffner, G., 2000. The
profitability of trading volatility using real-valued and
symbolic models. In IEEE/IAFE/INFORMS 2000
Conference on Computational Intelligence for
Financial Engineering, New York City, NY, pages 8–
11.
Schlottmann, F., Seese, D., 2004. Financial applications of
multiobjective evolutionary algorithms: recent
developments and future research directions. In
Coello-Coello, C.; Lamont, G. (eds.): Applications of
Multi-Objective Evolutionary Algorithms, World
Scientific, Singapore, pages 627-652.
Shekar, B., Natarajan, R., 2004. A Framework for
Evaluating Knowledge-Based Interestingness of
Association Rules. Fuzzy Optimization and Decision
Making 3, 157-185.
Skabar, A., Cloete, I., 2001. Discovery of Financial
Trading Rules. Proceedings of the IASTED
International Conference on Artificial Intelligence and
Applications.
AN EMPIRICAL STUDY OF SIGNIFICANT VARIABLES FOR TRADING STRATEGIES
335