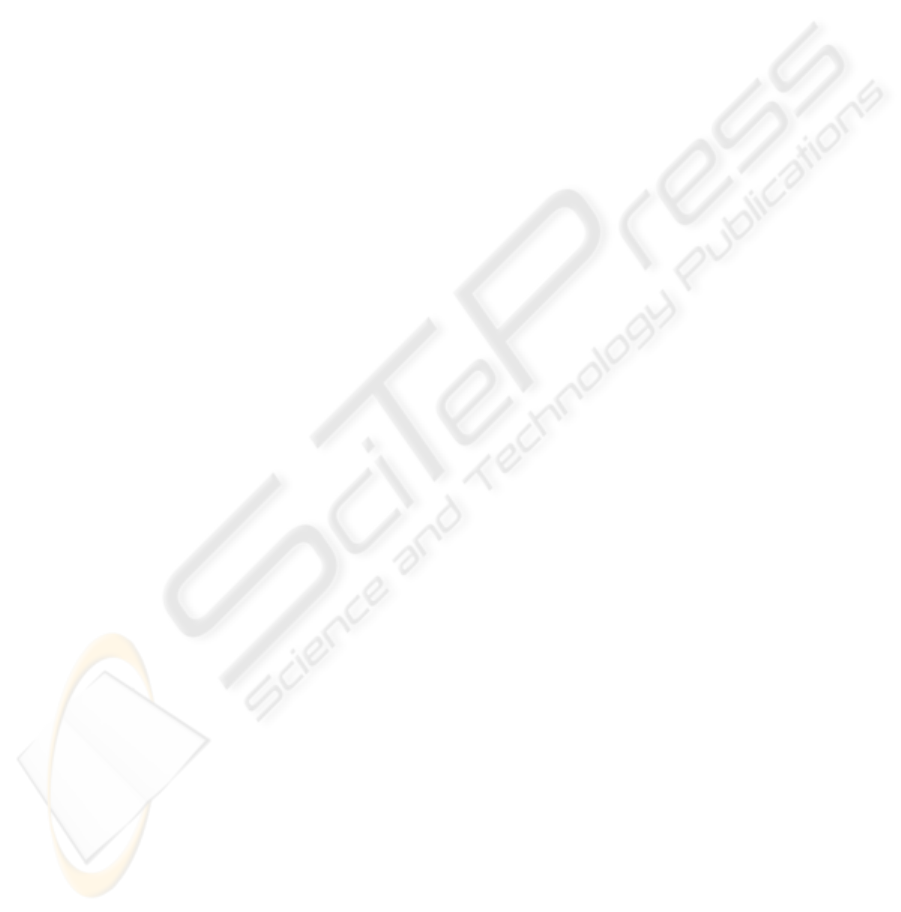
If the preferences of occupant A have not been
changed for “1” week, the TCI preference of
occupant A can be updated according to the
predicted TCI value that occurs most often
(personalised TCI value) in all related rules.
An occupant’s personal agent will negotiate with
local agents in respect of which learning mechanism
to be used for preferences learning. Such design of
occupant’s preferences, the rule, and the learning
process enables MASBO to search for the best
preferences learning mechanisms.
5 EVALUATION
Investigations have been made on previous similar
projects (Davidsson and Boman, Rutishauser et al.,
2005, Callaghan et al., Hagras et al., 2004) on how
to evaluate the model and eventually the
implemented system.
Qualitative simulation.
Energy consumption quantitative analysis that
compares the energy consumption of using or
not using MASBO.
Satisfaction quantitative analysis that checks
how well temperature or lighting history
records meet specified policies.
Satisfaction quantitative analysis that compares
the learned rules with the occupant’s journal
entries.
The number of rules learned over time that
indicates how well the Multi-agent system
performs.
6 CONCLUSIONS AND FUTURE
WORK
Many efforts have been made on using multi-agent
system for intelligent building control. However,
while previous work has addressed most of the
important features for MAS based intelligent
building control, we claim that no research has been
done to consider all the following requirements:
Energy efficiency and occupants' comfort
Preferences learning in shared environment
Personalized control and feedback
Human readable and accurate knowledge
representation
Sophisticated agent platform and techniques
We believe above requirements are essential to a
successful intelligent building environment and a
complete solution should be able to tackle all of
them. The first four requirements have been
discussed in this paper. The fifth requirement was
presented in (Qiao et al., 2006). The last requirement
will be addressed in the next step of this project.
Future work will be carried out in the following
steps 1) testing different strategies for preferences
learning and decision making in a simulated
environment; 2) developing MASBO on advanced
agent platform; 3) system integration with wireless
sensor network and building automation system, and
4) evaluation by experiments in real world buildings.
ACKNOWLEDGEMENTS
This paper is based on the research outcomes of
CMIPS project, sponsored by the Department of
Trade and Industry, UK. The project is a
collaborative work between University of Reading,
Thales PLC and Arup Group Ltd.
REFERENCES
Callaghan, V., Clarke, G., Colley, M. & Hagras, H. (2001)
A Soft-Computing DAI Architecture for Intelligent
Buildings. Soft Computing Agents: New Trends for
Designing Autonomous Systems. Springer-Verlag.
Davidsson, P. & Boman, M. (1998) Energy Saving and
Value Added Sevices: Controlling Intelligent
Buildings Using a Multi-Agent Systems Approach.
DA/DSM Europe DistribuTECH. PennWell.
Davidsson, P. & Boman, M. (2005) Distributed
Monitoring and Control of Office Buildings by
Embedded Agents. Information Sciences, 171, 293-
307.
Hagras, H., Callaghan, V., Colley, M., Clarke, G., Pounds-
Cornish, A. & Duman, H. (2004) Creating an
Ambient-Intelligence Environment Using Embedded
Agents. IEEE Intelligent Systems, 19, 12-20.
Qiao, B., Liu, K. & Guy, C. (2006) A Multi-Agent System
for Building Control. IEEE/WIC/ACM International
Conference on Intelligent Agent Technology. Hong
Kong.
Rutishauser, U., Joller, J. & Douglas, R. (2005) Control
and Learning of Ambience by an Intelligent Building.
IEEE Transactions on Systems, Man, and Cybernetics,
Part A: Systems and Humans, 35, 121-132.
Wooldridge, M. (2002) Introduction to MultiAgent
Systems, Wiley.
ICEIS 2007 - International Conference on Enterprise Information Systems
164