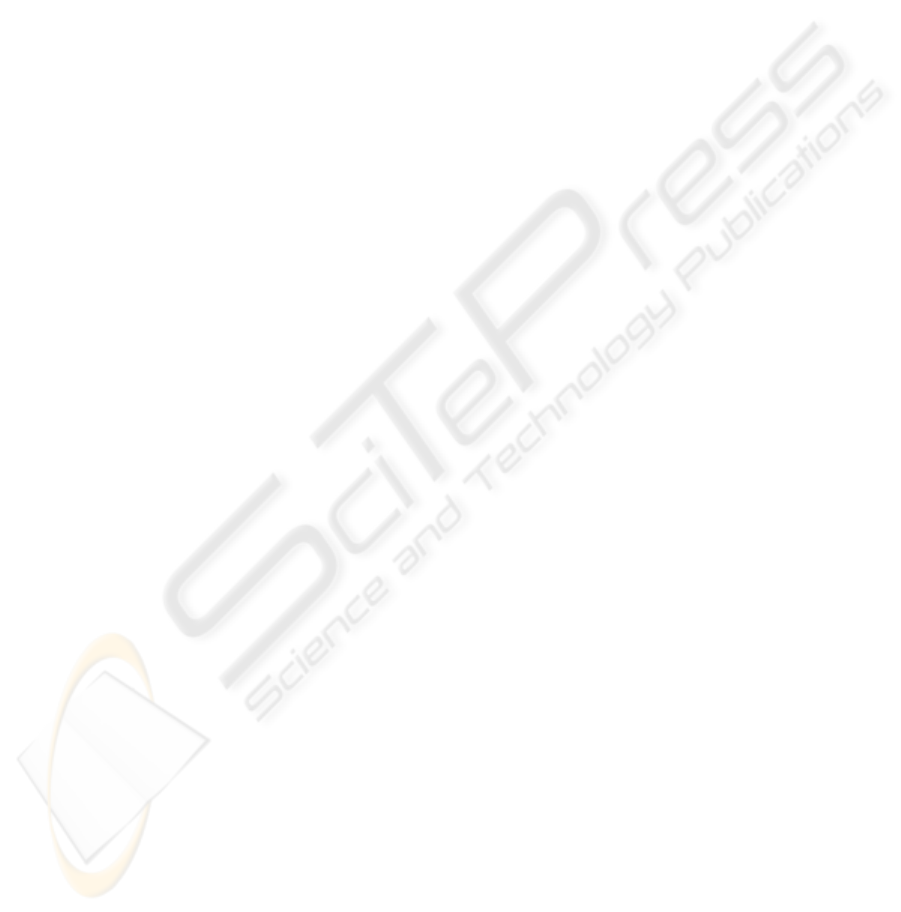
based on P@5 we obtained on average 9.4% of
improvement when using two systems and 9.9%
when using five systems. These results lead to two
main conclusions: the method we present is efficient
however, there is room for more improvements,
specifically using more systems.
In a further analysis, we have explored the
hypothesis according to which variability in results
is lower when MAP is higher. This hypothesis
would support the fact that there is more potentiality
to fuse the best systems using our method when the
task is difficult. However this analysis has led to the
conclusion that there is no direct correlation between
the variability in each query considered individually
and the possibility for our method to improve the
results.
Future works will investigate different directions.
First, our approach is based on precision at 5 (P@5);
we would like to analyse the effect of the number of
documents chosen in order to see if fewer
documents would be enough. A second direction
concerns evaluation. We would like to consider
residual collection evaluation, that means that we
would delete judged documents when evaluate the
results. This will be crucial if we want to consider
other performance measures such as high precision.
Longer term studies concern first a way to predict
the effectiveness of the method. We show that
variability is not a good predictor of that but other
direction have to be explored and probably
combined such as the number of retrieved
documents, the type of query, the models used in the
search engines considered, etc.. Finally another
future work is related to different fusion techniques.
We would like to consider different query features in
order to predict which system would be the best to
select to treat the query. This could be combined
with relevance information as studied in this paper.
(He & Ounis, 2003) and (Mothe & Tanguy, 2005)
open tracks in this direction considering query
difficulty prediction as a clue to perform better
information retrieval.
ACKNOWLEDGEMENTS
This work was carried out in the context of the
European Union Sixth Framework project “Ws-
Talk”.
We also want to thanks Yannick Loiseau for his
participation in programming.
REFERENCES
Harman, D., 1994. Overview of the Third Text REtrieval
Conference (TREC-3), 3
rd
Text Retrieval Conference,
NIST Special Publication 500-226, pp 1-19.
Beitzel, S. M., Frieder, O., Jensen, E. C., Grossman, D.
Chowdhury A., Goharian, N., 2003. Disproving the
fusion hypothesis: an analysis of data fusion via
effective information retrieval strategies. SAC'03,
ACM symposium on Applied computing, pp. 823-827.
Buckley, C., Harman, D., 2004. The NRRC reliable
information access (RIA) workshop. 27
th
International
ACM SIGIR Conference on Research and
Development in Information Retrieval, pp. 528-529.
Fox E. A., Shaw, J. A., 1994. Combination of Multiple
Searches. 2
nd
Text Retrieval Conference (TREC-2),
NIST Special Publication 500-215, pp. 243-252.
He, B., Ounis, I., 2003. University of Glasgow at the
Robust Track – A query-based Model Selection
Approach for Poorly-performing Queries. 12
th
Text
Retrieval Conference (TREC-12), NIST Special
Publication 500-255, pp. 636-645.
Lee, J., 1997. Analysis of multiple evidence combination.
22
th
International ACM SIGIR Conference on
Research and Development in Information Retrieval,
pp. 267-276.
Mothe, J., Tanguy, L., 2005. Linguistic features to predict
query difficulty - A case study on previous TREC
campaign. SIGIR workshop on Predicting Query
Difficulty - Methods and Applications, pp. 7-10.
Voohrees E., Harman, D., 2001. Overview of TREC 2001.
10
th
Text Retrieval Conference, NIST Special
Publication 500-255, pp. 1-15.
RELEVANCE FEEDBACK AS AN INDICATOR TO SELECT THE BEST SEARCH ENGINE - Evaluation on TREC
Data
189