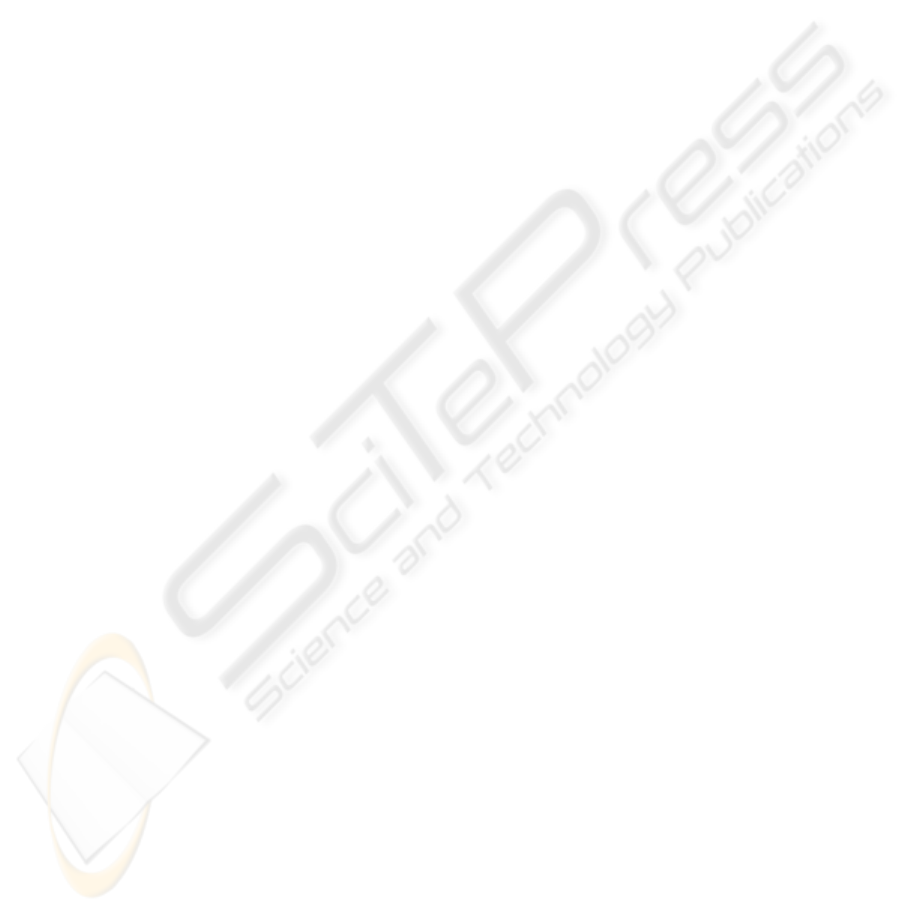
for any applications where natural language may be
used. Such systems utilise a natural ability of the
human user, and therefore have the potential for
making computer control effortless and natural.
Furthermore, due to the very dense information that
can be coded in speech, speech based human
computer interaction (HCI) can provide richness
comparable to human-to-human interaction.
In recent years, significant progress has been
made in speech recognition technology, making
speech an effective modality in both telephony and
multimodal human-machine interaction. Speech
recognition systems have been built and deployed
for numerous applications. The technology is not
only improving at a steady pace, but is also
becoming increasingly usable and useful. However,
speech recognition technology using voice signals
has three major shortcomings - it is not suitable in
noisy environments such as vehicles or factories, not
applicable for people with speech impairment
disability such as people after a stroke attack, and it
is not applicable for giving discrete commands when
there may be other people talking in vicinity.
This research reports how to overcome these
shortcomings with a voice recognition approach,
which identifies silent vowel-based verbal
commands without the need to sense the voice sound
output of the speaker. Possible users of such a
system would be people with disability, workers in
noisy environments, and members of the defence
forces. When we speak in noisy environments, or
with people with hearing deficiencies, the lip and
facial movements often compensate the lack of
audio quality.
The identification of speech by evaluating lip
movements can be achieved using visual sensing, or
tracking the movement and shape using mechanical
sensors (Manabe et al., 2003), or by relating the
movement and shape to facial muscle activity (Chan
et al., 2002; Kumar et al., 2004). Each of these
techniques has strengths and limitations. The video
based technique is computationally expensive,
requires a camera monitoring the lips that is fixed to
a view of the speaker’s head, and it is sensitive to
lighting conditions. The sensor based technique has
the obvious disadvantage that it requires the user to
have sensors fixed to the face, making the system
not user friendly. The muscle monitoring systems
have limitations in terms of low reliability. In the
following sections, the approach is reported of
recording activity of the facial muscles (fEMG) for
determining silently commands from a human
speaker.
Earlier work reported by the authors have
demonstrated the use of multi-channel surface
electromyogram (SEMG) to identify the unspoken
vowel based on the normalized integral values of
facial EMG during the utterance, and this
construction had been tested with native Australian
English speakers. The main concern with such
systems is the difficulty to work across people of
different backgrounds, and the main challenge is the
ability of such a system to work for people of
different languages – native ones as well as foreign
ones. Consequently, in this particular work the error
in classification of the unvoiced English and German
vowels by a group of German native speakers are
compared. Hence, this investigation covers the
application case of two different languages used by
native speakers, and the case of speakers talking and
commanding in a foreign language.
2 THEORY
This research aims to recognize the multi-channel
surface electromyogram of the facial muscle with
speech and identify the variation in the accuracy of
classification for two different languages, German
and English. Articulatory phonetics considers the
anatomical detail of the utterance of sounds. This
requires the description of speech sounds in terms of
the position of the vocal organs, and it is convenient
to divide the speech sounds into vowels and
consonants. The consonants are relatively easy to
define in terms of shape and position of the vocal
organs, but the vowels are less well defined and this
may be explained because the tongue typically never
touches another organ when making a vowel
(Parsons, 1986). When considering speech
articulation, the shapes of the mouth during speaking
vowels remain constant while during consonants the
shape of the mouth changes.
2.1 Face Movement and Muscles
Related to Speech
The human face can communicate a variety of
information including subjective emotion,
communitive intent, and cognitive appraisal. The
facial musculature is a three dimensional assembly
of small, pseudo-independently controlled muscular
lips performing a variety of complex orfacial
functions such as speech, mastication, swallowing
and mediation of motion (Lapatki et al., 2003).
When using facial SEMG to determine the shape of
lips and mouth, there is the issue of the proper
SILENT BILINGUAL VOWEL RECOGNITION - Using fSEMG for HCI based Speech Commands
69