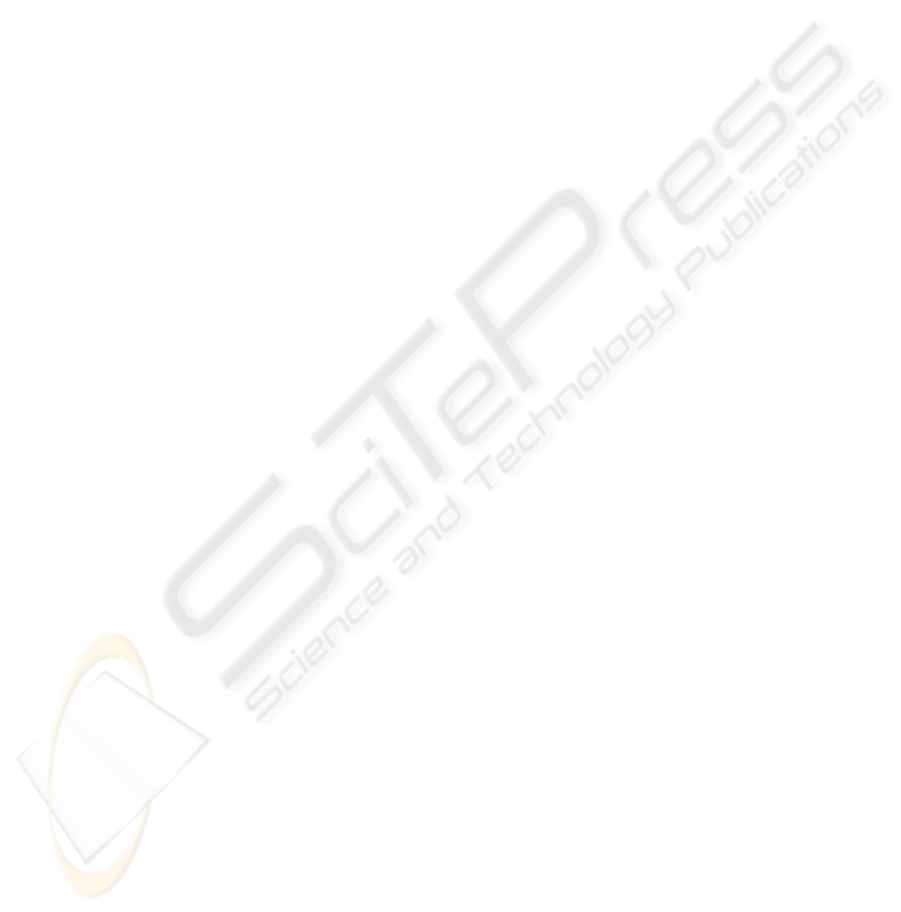
as a learning tool since it gathers information,
defined by experts, that is needed for the
tuberculosis diagnosis.
The NeuralTB system can be easily installed in
hospitals or health care units and can also be
executed in portable computers that are carried to
different regions. The approach to incorporate the
knowledge into the system, allowing an easy
maintenance of the information, guarantees the
lifetime of the proposal.
Currently the NeuralTB system is being installed
in health care units in the Rio de Janeiro, the number
one city for TB cases in Brazil. This effort will
facilitate the implantation of a network to integrate
diverse professionals and specialists in tuberculosis.
During the system operation we will be able to
validate the impact of this initiative.
As next steps, we intend to integrate the
NeuralTB input data form with other questionnaire
items used during an anamnesis interview. Actually,
the proposal is to integrate the input form with the
system that is used in the hospital reception. As a
result, the attendance will use a single environment
to register all data related to patients. Another
enhancement is to develop queries in the central
database to extract the information that comes from
the various health care units. The knowledge of
which information should be extracted can also be
modelled and incorporated into the repository. Data
quality metrics (Chapman, 2005) will also be
applied to ensure network information quality. This
is quite important as network performance relies on
the accuracy of questionnaire answers. The
continuous update of the neural model with
incoming new data is also being developed. This
involves stability studies and the monitoring of TB
main features, trying to track disease evolvement in
time and geographically.
We expect that the accomplishments of this
project bring social benefits, allow a better
integration of the information technology in the
diagnosis domain, and provide an infrastructure to
enable an efficient communication and information
exchange among tuberculosis experts.
ACKNOWLEDGEMENTS
The authors thank the Tuberculosis Research Unit,
Faculty of Medicine, Federal University of Rio de
Janeiro, for making available the data used in this
work and CAPES, CNPq, and FAPERJ for
financially supporting this project.
REFERENCES
Castelo A., Kritski A.L., Werneck A., Lemos A.C.,
Ruffino Netto A., et al., 2004. Brazilian Directives for
Tuberculosis. J Brás Pneumo, 30 (supl 1). 1- 86. In
Portuguese.
Chapman, A., 2005. Principles of Data Quality, Report,
Global Biodiversity Information Facility.
Cook, J., 2000. XML Sets Stage for Efficient Knowledge
Management, IT professional, v.2, n.3, 55-57.
El-Solh, A.A., Hsiao, C.-B., Goodnough, S., Serghani, J.,
Grant, B.J.B., 1999. Predicting Active Pulmonary
Tuberculosis using an Artificial Neural Network.
Chest, 116, 968–973.
Hendriks, P., Vriens, D. 1999. Knowledge-Based Systems
and Knowledge Management: Friends or Foes?.
Information & Management, v.35, n.2 (Feb), 113-125.
Kohavi, R., 1995. A study of cross-validation and
bootstrap for accuracy estimation and model selection.
In International Joint Conference on Artificial
Intelligence.
Mello, F.C.Q., 2001. Smear Negative Pulmonary
Tuberculosis Predicting Models, Ph.D. Thesis,
Medicine Faculty, Federal University of Rio de
Janeiro, Brazil. In Portuguese.
Perkins, M.D.., Kritski, A.L., 2002. Perspectives.
Diagnostic Testing in the Control of Tuberculosis. In:
Bull WHO, 80 (6), 512-513.
Probst, G., Raub, S., Romhardt, K. 1999. Managing
Knowledge: Building Blocks for Success, 368 pp,
ISBN: 0-471-99768-4.
Rabarijaona, A., Dieng, R., Olivier, C., Quaddari, R. 2000.
Building and Searching an XML-Based Corporate
Memory, IEEE Intelligent Systems, v.15, n.3 (May),
56-63.
Sarmiento, O., Weigle, K., Alexander, J., Weber, D.J.,
Miller, W., 2003. Assessment by Meta-Analysis of
PCR for Diagnosis of Smearnegative Pulmonary
Tuberculosis, Journal of Clinical Microbiology, 41,
3233-3240.
Santos, A.M. 2003. Neural Networks and Classification
Trees Applied to Smear Negative Pulmonary
Tuberculosis Diagnosis, Ph.D. Thesis, COPPE/ UFRJ,
Rio de Janeiro, Brazil. In Portuguese.
Santos, A.M., Pereira, B.B., Seixas, J.M., Mello, F.C.Q.,
Kristski, A.L., 2006. Neural Networks: an Application
for Predicting Smear Negative Pulmonary
Tuberculosis. In: Balakrishnan, N.; Auget, J.L.;
Mesbah, M.; Molenberghs, G. (org.). In: Advances in
Statistical Methods for The Health Sciences. 279-292.
Seixas, J.M., Calôba, L.P., Delpino, I., 1996. Relevance
Criteria for Variable Selection in Classifier Design. In:
International Conference on Engineering Applications
of Neural Networks, 451-454.
Vassali, M.R., Seixas, J.M., Calôba, L.P., 2002. A Neural
Particle Discriminator Based on a Modified Art
Architecture. In: IEEE International Symposium on
Circuits and Systems, v. II., 121-124.
World Health Organization (WHO), 2002. Stop TB annual
report 2001.
NEURALTB WEB SYSTEM: Support to the Smear Negative Pulmonary Tuberculosis Diagnosis
203