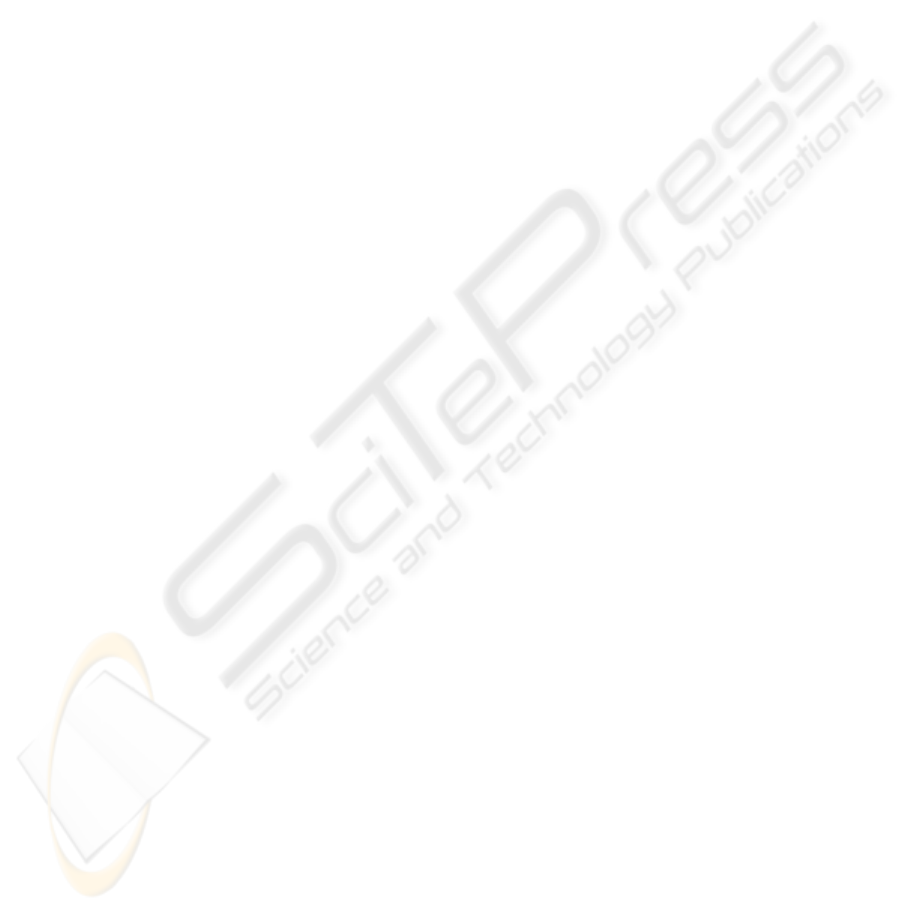
rules to facilitate his comprehension of the extracted
knowledge.
Experiments were carried out in two data sets aim-
ing to evaluate the knowledge quality expressed by
the generalized rules. The analysis showed that de-
pending on the side occurrence of a generalization
item a different group of measures has to be used
to evaluate the GAR quality. In other words, if a
rule presents a generalized item in the lhs, the lhs
measures (Table 2) have to be used, since these mea-
sures have a better behavior when applied to evaluate
a GAR with a generalized item in the lhs; the same
idea applies to the rhs. Thus, this paper gives a huge
contribution to the post-processing knowledge step.
An analytical evaluation of some presented objec-
tive measures is presented in (Carvalho et al., 2007a)
to base the empirical results.
ACKNOWLEDGEMENTS
We wish to thank the Instituto Fbrica do Milnio (IFM)
and Fundao de Amparo Pesquisa do Estado de So
Paulo (FAPESP) for the financial support.
REFERENCES
Adamo, J.-M. (2001). Data Mining for Association Rules
and Sequential Patterns. Springer-Verlag.
Agrawal, R. and Srikant, R. (1994). Fast algorithms for
mining association rules. In Bocca, J. B., Jarke, M.,
and Zaniolo, C., editors, Proceedings of the 20th In-
ternational Conference on Very Large Data Bases,
VLDB’94, pages 487–499.
Baixeries, J., Casas, G., and Balc
´
azar, J. L. (2000). Fre-
quent sets, sequences, and taxonomies: New, efficient
algorithmic proposals. Technical Report LSI-00-78-
R, Departament de LSI – Universitat Polit
`
ecnica de
Catalunya.
Carvalho, V. O., Rezende, S. O., and Castro, M. (2007a).
An analytical evaluation of objective measures beha-
vior for generalized association rules. In IEEE Sympo-
sium on Computational Intelligence and Data Mining
– CIDM/2007. In Press.
Carvalho, V. O., Rezende, S. O., and Castro, M. (2007b).
Evaluating generalized association rules through ob-
jective measures. In Deved
˘
zic, V., editor, IASTED In-
ternational Conference on Artificial Intelligence and
Applications – AIA 2007. ACTA Press.
Chung, F. and Lui, C. (2000). A post-analysis frame-
work for mining generalized association rules
with multiple minimum supports. In Post-
Processing in Machine Learning and Data
Mining: Interpretation, Visualization, Inte-
gration, and Related Topics (Workshop within
KDD’2000). Retrivied November 17, 2006, from
http://www.cs.fit.edu/ pkc/kdd2000ws/post.html.
Domingues, M. A. and Rezende, S. O. (2005). Using ta-
xonomies to facilitate the analysis of the association
rules. In Proceedings of ECML/PKDD’05 – The Se-
cond International Workshop on Knowledge Disco-
very and Ontologies (KDO-2005), pages 59–66.
Han, J. and Fu, Y. (1995). Discovery of multiple-level asso-
ciation rules from large databases. In Dayal, U., Gray,
P. M. D., and Nishio, S., editors, Proceedings of 21th
International Conference on Very Large Data Bases
VLDB’95, pages 420–431.
Han, J. and Fu, Y. (1999). Mining multiple-level association
rules in large databases. IEEE Transactions on Know-
ledge and Data Engineering, 11(5):798–805.
Hipp, J., Myka, A., Wirth, R., and G
¨
untzer, U. (1998). A
new algorithm for faster mining of generalized asso-
ciation rules. In Zytkow, J. M. and Quafafou, M., edi-
tors, Proceedings of the 2nd European Symposium on
Principles of Data Mining and Knowledge Discovery
PKDD’98, pages 74–82.
Huang, Y.-F. and Wu, C.-M. (2002). Mining genera-
lized association rules using pruning techniques. In
Proceedings of the 2002 IEEE International Confe-
rence on Data Mining (ICDM’02), pages 227–234,
Washington, DC, USA. IEEE Computer Society.
Srikant, R. and Agrawal, R. (1995). Mining generalized
association rules. In Proceedings of the 21th In-
ternational Conference on Very Large Data Bases
VLDB’95, pages 407–419.
Srikant, R. and Agrawal, R. (1997). Mining generalized
association rules. Future Generation Computer Sys-
tems, 13(2/3):161–180.
Sriphaew, K. and Theeramunkong, T. (2004). Fast algo-
rithms for mining generalized frequent patterns of ge-
neralized association rules. IEICE Transactions on In-
formation and Systems, 87(3):761–770.
Tan, P.-N., Kumar, V., and Srivastava, J. (2004). Selecting
the right objective measure for association analysis.
Information Systems, 29(4):293–313.
Weber, I. (1998). On pruning strategies for discovery
of generalized and quantitative association rules. In
Bing, I. L., Hsu, W., and Ke, W., editors, Proceedings
Knowledge Discovery and Data Mining Workshop
Pricai’98. 8 pp.
Yen, S.-J. and Chen, A. L. P. (2001). A graph-based ap-
proach for discovering various types of association
rules. IEEE Transactions on Knowledge and Data En-
gineering, 13(5):839–845.
OBTAINING AND EVALUATING GENERALIZED ASSOCIATION RULES
315