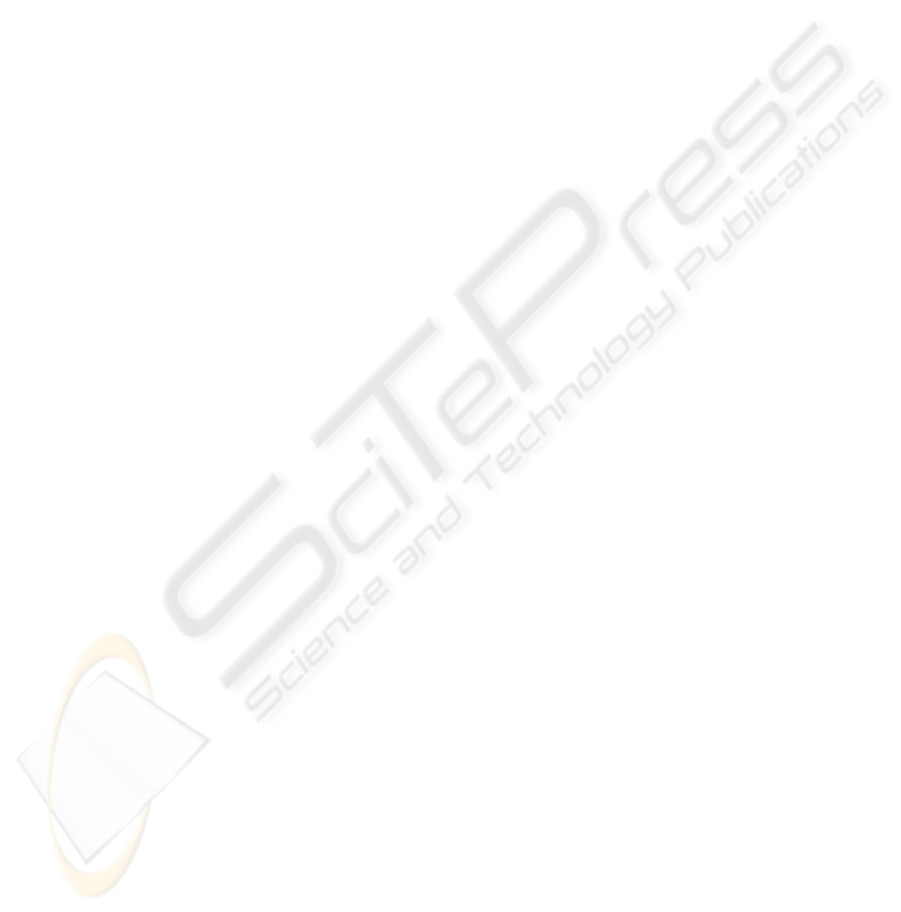
each variable, we propose a measurement
methodology. The main contribution of this model is
to address the moderating functionality of IQ
awareness in the relationship of IQ and decision
quality.
Based on the model, we provide an empirical
scenario to facilitate the model validation. In the
application scenario, we point out several
experimental indicators such as human learning,
decision issue selection and measurement
calculation.
The model in this paper has several possible
extensions. First, more IQ dimensions could be
included in the IQ measurement. For example, we
could include completeness, relevancy, consistency
etc.. Second, more decision quality measurements
could be taken into consideration, such as decision
consistency and decision consensus. Third, more
experiment indicators could be included to a more
comprehensive and concrete application scenario.
For instance, we could develop a concrete finance
situation for each decision maker or increase the task
complexity of the decision environments. Overall
future work of this research is improving the
theoretical model and building a comprehensive
empirical scenario.
5 REFERENCE
Ahituv, N., Igbaria, M., and Stella, A., “The Effects of
Time Pressure and Completeness of Information on
Decision Making,” Journal of Management
Information Systems, (15:2), 1998, pp. 153-172.
Ballou, D. P. and Pazer, H. L. Modelling data and process
quality in multi-input, multi-output information
systems. Manage. Science. 31, 2 (1985), pp. 150-162.
Ballou D. P., Wang R. Y., Pazer H. L., Tayi G. K.,
Modeling Information Manufacturing Systems to
Determine Information Product Quality, Management
Science, v.44 n.4, p.462-484, April 1998.
Baron J. and Hershey J. C.. Outcome bias in decision
evaluation, Journal of Personality and Social
Psychology. Apr Vol 54(4) , 1988, pp: 569-579.
Chengalur-Smith I. N., Ballou D. P., Pazer H. L.: The
Impact of Data Quality Information on Decision
Making: An Exploratory Analysis. IEEE Trans.
Knowl. Data Eng. 11(6): 853-864 (1999)
Cinzia C., Chiara F., and Barbara P., Time-Related Factors
of Data Quality in Multichannel Information Systems,
Journal of Management Information Systems Vol. 20
No. 3, pp. 71 – 91, 2004
Fisher C. W., Chengalur-Smith I., Ballou D. P.. The
Impact of Experience and Time on the Use of Data
Quality Information in Decision Making, Information
Systems Research/Vol. 14, No. 2, 2003.
Ge M., Helfert M.. A Framework to Assess Decision
Quality Using Information Quality Dimensions, 11th
International Conference on Information Quality,
MIT. November 10-12, 2006
Huang K., Lee Y. W., Wang R. Y.. Quality Information
and Knowledge Management, Prentice Hall, 1st
edition, ISBN: 0130101419, 1999.
Jung W., Olfman L., An Experimental Study of the Effects
of Contextual Data Quality and Task Complexity on
Decision Performance, Information Reuse and
Integration, Conf, 2005. IRI -2005
Keller K.L. and Staelin R. Effects of Quality and Quantity
of Information on Decision Effectiveness, Journal of
Consumer Research, volume 14, page 200
Lee Y. W., Strong D., Kahn B., and Wang R.Y., AIMQ: A
Methodology for Information Quality Assessment,
Information & Management, December 2002, Volume
40, Issue 2, pp. 133-146.
Matthew B., Raiendra S. P.; Brenda M.; A conceptual
framework and belief-function approach to assessing
overall information quality, International journal of
intelligent systems, 2003, vol. 18, no 1 (42 ref.), pp.
51-74
Meager N., Tyers C., Perryman S., Rick J. Willison R.,
Awareness, knowledge and exercise of individual
employment rights, Employment Relations Research
Series No.15
Olson J. E., Data Quality: The Accuracy Dimension,
Publisher: Morgan Kaufmann (December 26, 2002),
ISBN: 1558608915
Redman, T. Data Quality: The Field Guide, Publisher:
Digital Press (January 15, 2001), ISBN: 1555582516
Redman, T. Data Quality For The Information Age,
Publisher: Artech House Publishers (December 1996),
ISBN: 0890068836
Parssian A., Sarkar S., Jacob V. S., Assessing Data
Quality for Information Products: Impact of Selection,
Projection, and Cartesian Product, Management
Science Volume 50, Issue 7, July 2004.
Shankaranarayan G., Ziad M., Wang R.Y.. Managing Data
Quality in Dynamic Decision Environments: An
Information Product Approach, Journal of Data
Management, 2003.
Wand Y., Wang R. Y., Anchoring data quality dimensions
in ontological foundations, Communications of the
ACM, v.39 n.11, p.86-95, Nov. 1996
Wang, R. Y. and Strong, D. M. Beyond accuracy: What
data quality means to data consumers. J. Manage. Info.
Syst. 12, 4 (1996), pp. 5-34.
Wang R.Y., Lee Y. W., Pipino L., Strong D.M., Manage
Your Information as a Product," Sloan Management
Review, Summer 1998, Volume 39, No. 4. pp. 95-105.
Wang R. Y., A Product Perspective on Total Data Quality
Management, Communications of the ACM, February
1998, pp. 58-63.
A THEORETICAL MODEL TO EXPLAIN EFFECTS OF INFORMATION QUALITY AWARENESS ON DECISION
MAKING
169