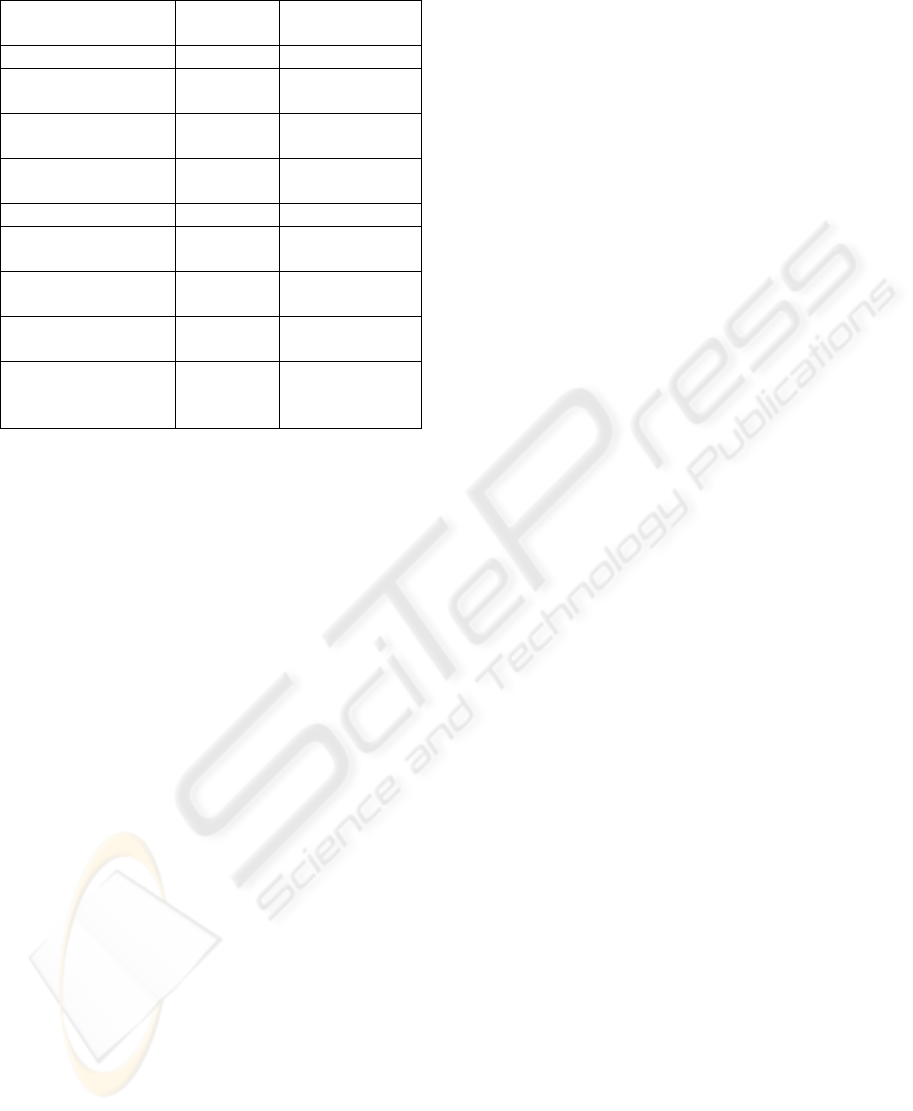
Table 2: Weights before and after improving.
Name Weights
before
Weights after
21 iterations
no-ratio weight -4,336 -4,336
Net income/Total
assets
-4,513 -4,323
Total liabilities/Total
assets
5,679 5,412
S.-t. assets/ S.-t.
liabilities
0,004 -0,276
no-ratio weight -4,336 -4,336
Net income/Total
assets
-4,513 -4,797
Total liabilities/Total
assets
5,679 5,715
S.-t. assets/ S.-t.
liabilities
0,004 -1,290
Performance of
bankruptcy
prediction (%)
77.78 92.41
Changing of weights allows seek the highest
accuracy of bankruptcy prediction
The highest impact on results has Short-term
assets/Short-term liabilities ratio – accuracy of
prediction increases rapidly due to changing of
weight of this ratio.
5 CONCLUSIONS
The presented model for forecasting of changes
of companies financial standings on the basis
of Self-organizing maps also includes
multivariate discriminate analysis of
bankruptcy and feed-forward supervised
neural network; combination of these methods
makes original model suitable for forecasting.
The presented model works well with real
world data, the tests of the model with
presented dataset showed accuracy of
prediction with more than 92% performance.
Changing of weights with supervised neural
network allows seek the highest accuracy of
bankruptcy prediction.
Changing of the weights with supervised ANN
makes assumptions which ratios have highest
impact on prediction results.
Further works in this area would bee related with
testing of other discriminate models of bankruptcy,
experiments with other datasets, comparison with
other methods of bankruptcy prediction.
REFERENCES
Atiya, A.F., 2001. Bankruptcy prediction for credit risk
using neural networks: a survey and new results. In
IEEE Transactions on Neural Networks, Vol. 12, No.
4, pp. 929-935.
Altman, E., 1968. Financial Ratios, Discrimination
Analysis and the Prediction of Corporate Bankruptcy.
Journal of Finance. pp. 589-609.
Altman, E., 2000. Predicting Financial Distress of
Companies: Revisiting the Z-Score and ZETA®
Models. Working paper at
http://pages.stern.nyu.edu/~ealtman/Zscores.pdf .
Deboeck, G., 1998. Self-Organizing Maps Facilitate
Knowledge Discovery In Finance. Financial
Engineering News.
EDGAR Online, Inc. 1995-2006. http://pro.edgar-
online.com.
Kiviluoto, K., 1998. Predicting bankruptcies with the self-
organizing map. Neurocomputing, Vol. 21.pp.191–
201.
Kohonen, T., 1997. The Self-Organizing Map. In
Proceedings of the IEEE, 78.pp.1464-1480.
Martin-del-Prio, K., Serrano-Cinca, 1993. Self-Organizing
Neural Network: The Financial State of Spanish
Companies. In Neural Networks in Finance and
Investing. Using Artificial Intelligence to Improve
Real-World Performance. R.Trippi, E.Turban, Eds.
Probus Publishing,, pp. 341-357.
Merkevičius, E., Garšva, G., Girdzijauskas, S., 2006. A
Hybrid SOM-Altman Model for Bankruptcy
Prediction. In International Conference on
Computational Science (4), Lecture Notes in
Computer Science, 3994, pp. 364-371, ISSN 0302-
9743.
Shumway, T., 2001. Forecasting Bankruptcy More
Accurately: A Simple Hazard Model, Journal of
Business, Vol. 74, No. 1, pp. 101-124.
Vesanto, J., Himberg, J., Alhoniemi, E., Parhankangas, J.,
2000. SOM toolbox for Matlab 5, Technical report
A57, Helsinki University of Technology, Finland.
Zmijewski, M.E., 1984. Methodological Issues Related to
the Estimation of Financial Distress Prediction
Models. In Journal of Accounting Research 24
(Supplement). pp. 59-82.
FORECASTING OF CHANGES OF COMPANIES FINANCIAL STANDINGS ON THE BASIS OF
SELF-ORGANIZING MAPS
419