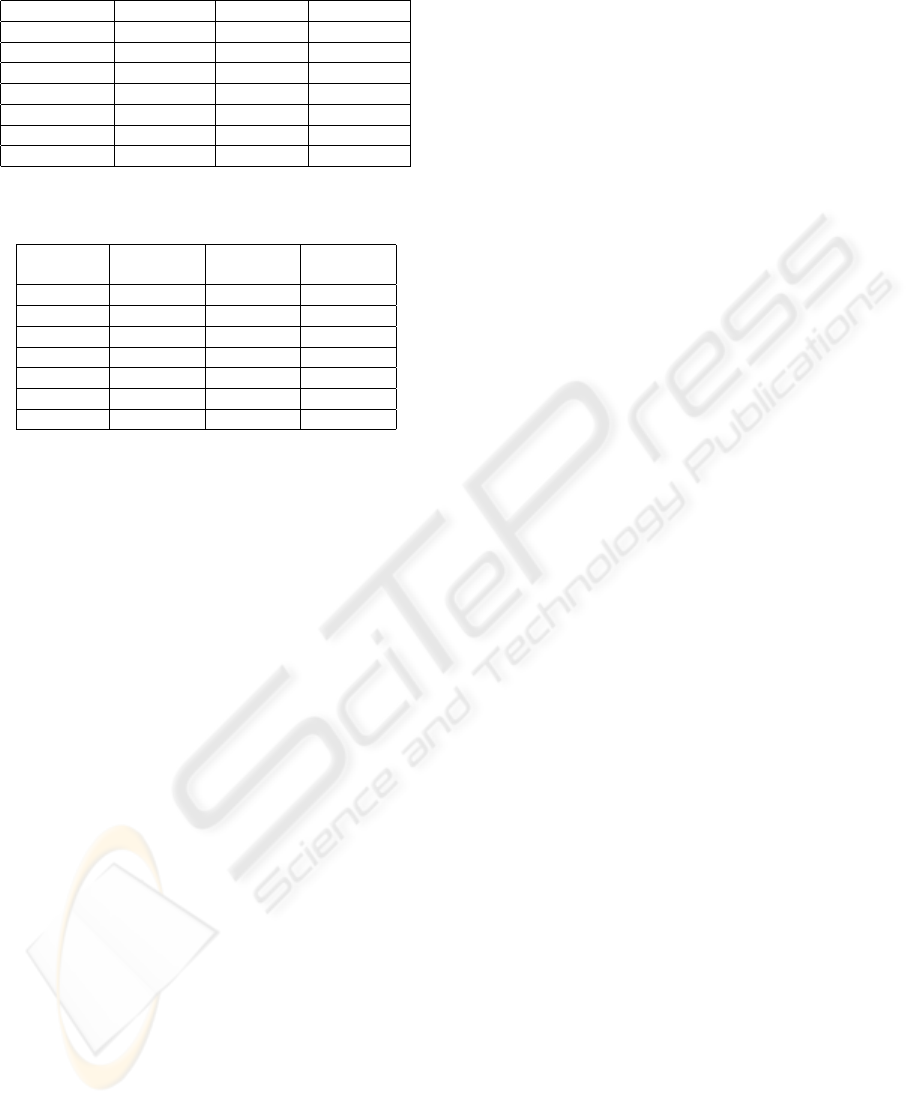
Table 4: Greedy execution time (hours).
Last attrib. All attrib. No candid.
beck-s 2,14054 9,98000 18,80485
farmer-d 5,988998 16,00856 21,52744
kaminski-v 7,60864 27,47413 36,43047
kitchen-l 24,11993 60,77219 66,94359
lokay-m 4,60200 4,46918 10,06760
sanders-r 1,24783 1,70260 2,07056
williams-w3 6,20118 10,23979 11,26965
Table 5: Improvement (and value of N) for each type of
candidate set when using 1000 attributes as input.
Last X-of-N All
attrib attribs. attribs.
beck-s 1,56 (9) 2,01 (18) 2,03 (20)
farmer-d 0,40 (5) 0,51 (10) 0,47 (12)
kaminski-v 1,15 (11) 1,36 (25) 1,37 (26)
kitchen-l 0,59 (7) 0,76 (18) 0,80 (21)
lokay-m 0,47 (5) 0,51 (5) 0,52 (10)
sanders-r 1,08 (4) 1,08 (4) 1,08 (4)
williams-w3 0,58 (3) 0,73 (6) 0,73 (6)
methods to look for it have also been designed and
tested. The experiments carried out show that the
use of the new attribute is beneficial with respect to
the classifier accuracy, and also that in many cases
it is interpretable. Besides, its construction process
is not classifier-specific. With respect to the search
methods we can say that the one considering as can-
didates to be included in
X-of-N only those attributes
sharing docs with the current
X-of-N, exhibits the best
tradeoff between CPU requirements and accuracy im-
provement. For the future we plan to go deeper in
this study (different designs for
X-of-N and different
search methods) and also to consider the inclusion of
more than one X-of-N attributes or the combination
of attribute selection and construction instead of per-
forming them in a two-stage process as in the current
work.
ACKNOWLEDGEMENTS
This work has been supported by the JCCM under project
PBI-05-022, MEC under project TIN1504-06204-C03-03
and the FEDER funds.
REFERENCES
Bekkerman, R., McCallum, A., and Huang, G. (2005). Au-
tomatic categorization of email into folders: Bech-
mark experiments on enron and sri corpora. Technical
report, Department of Computer Science. University
of Massachusetts, Amherst.
Brutlag, J. D. and Meek, C. (2000). Challenges of the email
domain for text classification. In ICML ’00: Proceed-
ings of the Seventeenth International Conference on
Machine Learning.
Freitas, A. A. (2001). Understanding the crucial role of
attributeinteraction in data mining. Artif. Intell. Rev.,
16:177–199.
Hu, Y.-J. (1998a). Constructive induction: covering at-
tribute spectrum In Feature Extraction, Construction
and Selection: a data mining perspective. Kluwer.
Hu, Y.-J. (1998b). A genetic programming approach to con-
structive induction. In 3rd Anual Genetic Program-
ming Conference.
Klimt, B. and Yang, Y. (2004). The enron corpus: a new
dataset for email classification research. In 15th Eu-
ropean Conference on Machine Learning, pages 217–
226.
Larsen, O., Freitas, A., and Nievola, J. (2002). Constructing
x-of-n attributes with a genetic algorithm. In Proc Ge-
netic and Evolutionary Computation Conf (GECCO-
2002).
Lewis, D. (1992). Representation and learning in informa-
tion retrieval. PhD thesis, Department of Computer
Science, University of Massachusetts.
Lewis, D. D. (1998). Naive (Bayes) at forty: The inde-
pendence assumption in information retrieval. In Pro-
ceedings of ECML-98, 10th European Conference on
Machine Learning, number 1398, pages 4–15, Chem-
nitz, DE. Springer Verlag, Heidelberg, DE.
Liu, H., Motoda, H., and Yu, L. (2002). Feature selection
with selective sampling. In Nineteenth International
Conference on Machine Learning, pages 395 – 402.
Mateo, J. L. and de la Ossa, L. (2006). Lio: an easy
and flexible library of metaheuristics. Technical
report, Departamento de Sistemas Informticos, Es-
cuela Polit
´
ecnica Superior de Albacete, Universidad
de Castilla-La Mancha.
McCallum, A. and Nigam, K. (1998). A comparison of
event models for naive bayes text classification. In
AAAI/ICML-98 Workshop on Learning for Text Cate-
gorization, pages 41–48.
Otero, F., Silva, M., Freitas, A., and NIevola, J. (2003). Ge-
netic programming for attribute construction in data
mining. In Genetic Programming: Proc. 6th Euro-
pean Conference (EuroGP-2003).
Salton, G. and Buckley, C. (1987). Term weighting ap-
proaches in automatic text retrieval. Technical report,
Cornell University.
Witten, I. H. and Frank, E. (2005). Data Mining: Practi-
cal Machine Learning Tools and Techniques (Second
Edition). Morgan Kaufmann.
Zheng, Z. (1995). Constructing nominal x-of-n attributes.
In International Joint Conference on Artificial Intelli-
gence (IJCAI-05). Morgan Kaufmann.
ICEIS 2007 - International Conference on Enterprise Information Systems
252