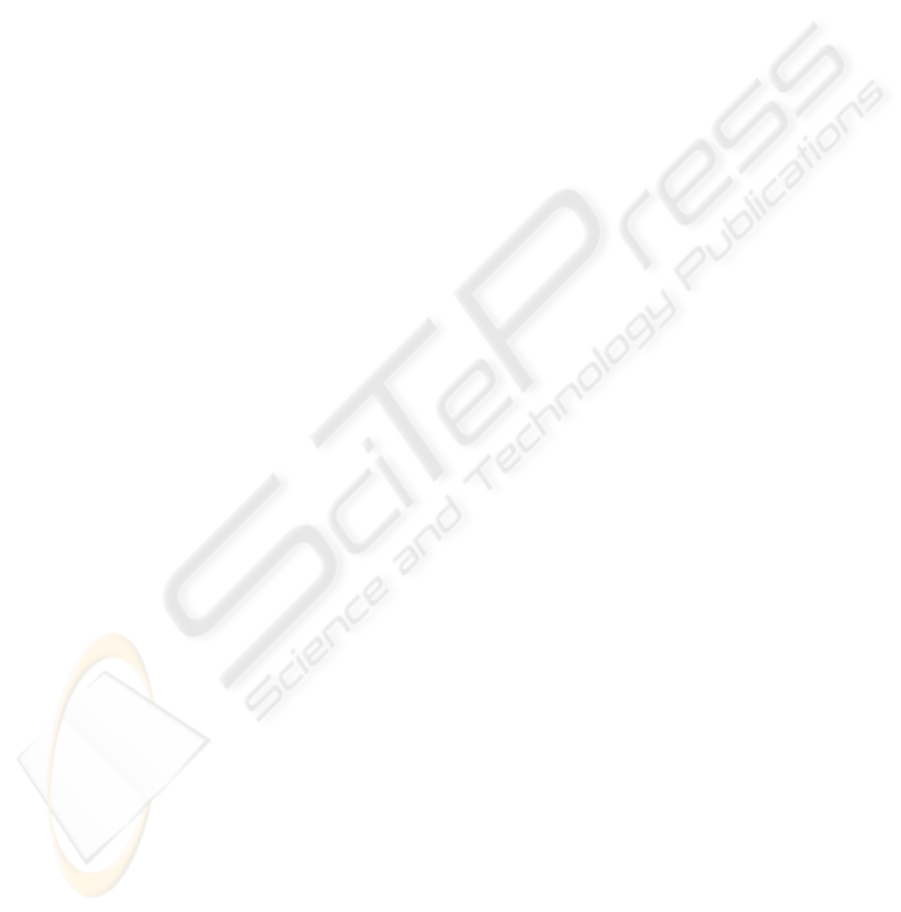
3. Comparison of results by couple of metrics (i,j)
where i is a conceptual data model quality
metric and j is a data quality metric.
Validation of interdependencies between QoD
dimensions. In order to validate the
interdependencies between QoD dimensions, we
focus on one status of CRM_DB. Then, for each
couple (d
1
,d
2
) of quality dimensions (Section 2.1,
namely, freshness and accuracy, completeness and
uniqueness, completeness and consistency), we use
the following approach:
1. Measure of d
1
and d
2
for CRM_DB
2. Artificial deterioration (or improvement) of the
data quality for the dimension d
1
3. Characterization of d
2
behavior.
6 CONCLUSIONS
This paper describes an ongoing research project
dedicated to the evaluation and improvement of data
quality in enterprise information systems. A
framework, called QUADRIS, has been proposed
and is currently under experimentations on very
large databases in three application domains: CRM
(EDF), medical domain (Institut Curie) and
geographical domain (Cemagref). The aim is to
identify the interdependencies between quality
dimensions considering two IS design levels: i)
interdependencies between dimensions of quality of
data (QoD), and ii) interdependencies between QoD
dimensions and quality of conceptual data model
(QoM) dimensions. This study already offers very
interesting and quantifiable perspectives for
designing quality-aware information systems and for
setting up cost optimal strategies for data quality
prevention and improvement in EIS.
ACKNOWLEDGEMENTS
The QUADRIS project is funded by the ANR ARA
Masses de Données Program (2005-2008), “Grant ARA-
05MMSA-0015”.
REFERENCES
Batini C., Ceri S., Navathe S.B., Conceptual database
design: An Entity Relationsip approach, Benjamen
Cummings, 1992.
Bobrowski, M.; Marré, M.; Yankelevich, D.: A Software
Engineering View of Data Quality. Intl. Software
Quality Week Europe (QWE'98), 1998.
Bouzeghoub M. Peralta, A Framework for Analysis of
Data Freshness, Intl. Workshop on Information
Quality in Information Systems (IQIS’2004), 2004.
Dasu T. Johnson T., Exploratory Data Mining and Data
Cleaning, John Wiley, 2003.
Gertz, M.; Tamer Ozsu, M.; Saake, G.; Sattler, K., Report
on the Dagstuhl Seminar: Data Quality on the Web.
SIGMOD Record, Vol. 33(1), March 2004.
Grigori D., Peralta V., Bouzeghoub M. Service Retrieval
Based on Behavioral Specifications and Data Quality
Requirements. 3rd Intl. Conf. on Business Process
Management (BPM'05), 2005.
Mecella, M.; Scannapieco, M.; Virgillito, A.; Baldoni, R.;
Catarci, T.; Batini, C.: Managing Data Quality in
Cooperative Information Systems. Intl. Conf. DOA,
CoopIS and ODBASE (DOA/CoopIS/ODBASE'02),
2002.
Naumann, F.; Leser, U.; Freytag, J.C., Quality-driven
Integration of Heterogeneous Information Systems.
Intl. Conf. on Very Large Databases (VLDB'99),
1999.
Naumann, F.; Freytag, J.C.; Leser, U., Completeness of
Information Sources. Intl. Workshop on Data Quality
in Cooperative Information Systems (DQCIS'03),
2003.
Peralta V.: Data Quality Evaluation in Data Integration
Systems, PhD Thesis, Université de Versailles, France
& Universidad de la República, Uruguay, November
2006.
Redman, T., Data Quality for the Information Age. Artech
House, 1996.
Segev, A.; Weiping, F., Currency-Based Updates to
Distributed Materialized Views. Intl. Conf. on Data
Engineering (ICDE'90), 1990.
Sisaïd S., Akoka J., Comyn-Wattiau I., Conceptual
Modeling Quality - From EER to UML Schemas
Evaluation, Intl. ER2002 Conf., 2002.
Sisaïd S., Akoka J., Comyn-Wattiau I., Use Case
Modeling and Refinement: A Quality-Based
Approach. Intl. ER2006 Conf., 2006.
Vassiliadis, P., Bouzeghoub, M., Quix, C.: Towards
Quality Oriented Data Warehouse Usage and
Evolution. Information Systems J., Vol 25, N°2, pp.
89-115, 2000.
Wang, R.; Strong, D., Beyond accuracy: What data quality
means to data consumers. J. on Management of
Information Systems, Vol. 12 (4):5-34, 1996.
A FRAMEWORK FOR QUALITY EVALUATION IN DATA INTEGRATION SYSTEMS
175