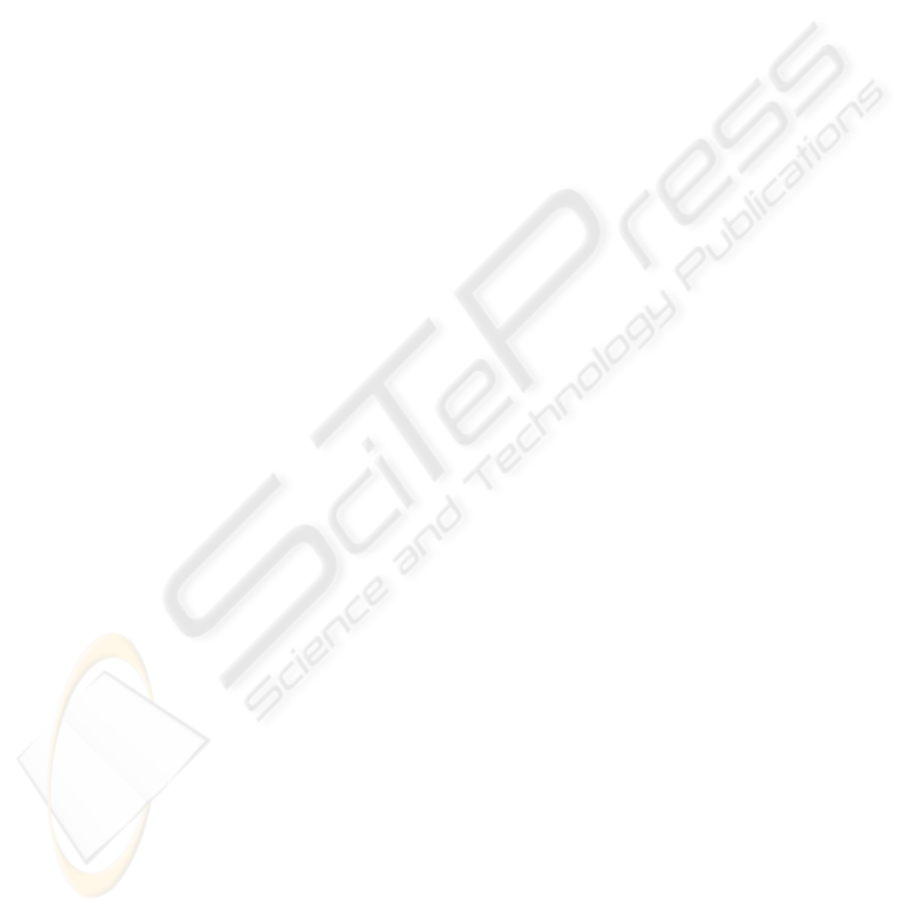
lationship between terms and people, but also for any
relationship between terms and possible conceptual
packets of terms, such as, sentences, messages, etc.
Similar idea to key terms/persons extraction can be
applied to these relationship; key terms are included
in many key sentences (or messages), and key sen-
tences (or messages) contain many key terms. We
would like to apply our approaches to various data
source, and lead to innovation and creativity support.
6 RELATED WORKS
The DISCUS project targets on innovation sup-
port through network-based communication (Gold-
berg et al., 2003). In addition to KEE methods, two
chance discovery approaches: KeyGraph (Ohsawa
and Yachida, 1998) and influence diffusion models
(IDM) (Matsumura et al., 2002) are used in the DIS-
CUS. Various methods have been proposed for find-
ing significant terms from text (key phrases (Witten
et al., 1999), topic words (Lawrie et al., 2001)). Many
approaches have been proposed for analyzing text
stream by topic detection, tracking, and segmentation
(Allan et al., 1998; Beeferman et al., 1999). Some
works have focused on finding persons in text-based
communication (Kamimaeda et al., 2005; Reich et al.,
2002). However, there had been no method for find-
ing significant terms and persons simultaneously.
ACKNOWLEDGEMENTS
We would like to thank to Hakuhodo Inc. for their
project collaboration. This work was sponsored by
the Air Force Office of Scientific Research, Air Force
Materiel Command, USAF (AF9550-06-1-0096 and
AF9550-06-1-0370). The US Government is autho-
rized to reproduce and distribute reprints for Govern-
ment purposes notwithstanding any copyright nota-
tion thereon.
REFERENCES
Allan, J., Carbonell, J., Doddington, G., Yamron, J., and
Yang, Y. (1998). Topic detection and tracking pilot
study: Final report.
Beeferman, D., Berger, A., and Lafferty, J. D. (1999). Sta-
tistical models for text segmentation. Machine Learn-
ing, 34(1-3):177–210.
Goldberg, D. E., Welge, M., and Llor
`
a, X. (2003). DISCUS:
Distributed Innovation and Scalable Collaboration In
Uncertain Settings. IlliGAL Report No. 2003017,
University of Illinois at Urbana-Champaign, Illinois
Genetic Algorithms Laboratory, Urbana, IL.
Kamimaeda, N., Izumi, N., and Hasida, K. (2005). Dis-
covery of key persons in knowledge creation based on
semantic authoring. In KMAP 2005.
Kleinberg, J. M. (1999). Authoritative sources in a hyper-
linked environment. Journal of the ACM, 46(5):604–
632.
Lawrie, D., Croft, W. B., and Rosenberg, A. (2001). Finding
topic words for hierarchical summarization. In SIGIR
’01: the 24th ACM SIGIR conference on Research and
development in information retrieval, pages 349–357.
Lin, D. (1998). An information-theoretic definition of sim-
ilarity. In Proc. 15th International Conf. on Machine
Learning, pages 296–304. Morgan Kaufmann, San
Francisco, CA.
Llor
`
a, X., Goldberg, D., Ohsawa, Y., Matsumura, N.,
Washida, Y., Tamura, H., Masataka, Y., Welge, M.,
Auvil, L., Searsmith, D., Ohnishi, K., and Chao, C.-J.
(2006). Innovation and creativity support via chance
discovery, genetic algorithms, and data mining. New
Mathematics and Natural Computation, 2(1):85–100.
Matsumura, N., Ohsawa, Y., and Ishizuka, M. (2002). Influ-
ence diffusion model in text-based communication. In
WWW ’02: Special interest tracks and posters of the
11th international conference on World Wide Web.
Ohsawa, Y.and Benson, N. E. and Yachida, M. (1998). Key-
Graph: Automatic indexing by co-occurencd graph
based on building construction metaphor. In Proceed-
ings of Advances in Digital Libraries, pages 12–18.
Porter, M. F. (1997). An algorithm for suffix stripping.
pages 313–316.
Reich, J. R., Brockhausen, P., Lau, T., and Reimer, U.
(2002). Ontology-based skills management: Goals,
opportunities and challenges. Universal Computer
Science, 8(5):506–515.
Salton, G. and Buckley, C. (1987). Term weighting ap-
proaches in automatic text retrieval. Technical report.
Salton, G. and McGill, M. J. (1986). Introduction to Mod-
ern Information Retrieval. McGraw-Hill, Inc. New
York, NY, USA.
Strehl, A. and Ghosh, J. (2000). Value-based customer
grouping from large retail data-sets. In Proceedings
of the SPIE Conference on Data Mining and Knowl-
edge Discovery: Theory, Tools, and Technology II, 24-
25 April 2000, Orlando, Florida, USA, volume 4057,
pages 33–42. SPIE.
Witten, I. H., Paynter, G. W., Frank, E., Gutwin, C., and
Nevill-Manning, C. G. (1999). Kea: practical auto-
matic keyphrase extraction. In DL ’99: the fourth
ACM conference on Digital libraries, pages 254–255.
DELINEATING TOPIC AND DISCUSSANT TRANSITIONS IN ONLINE COLLABORATIVE ENVIRONMENTS
21