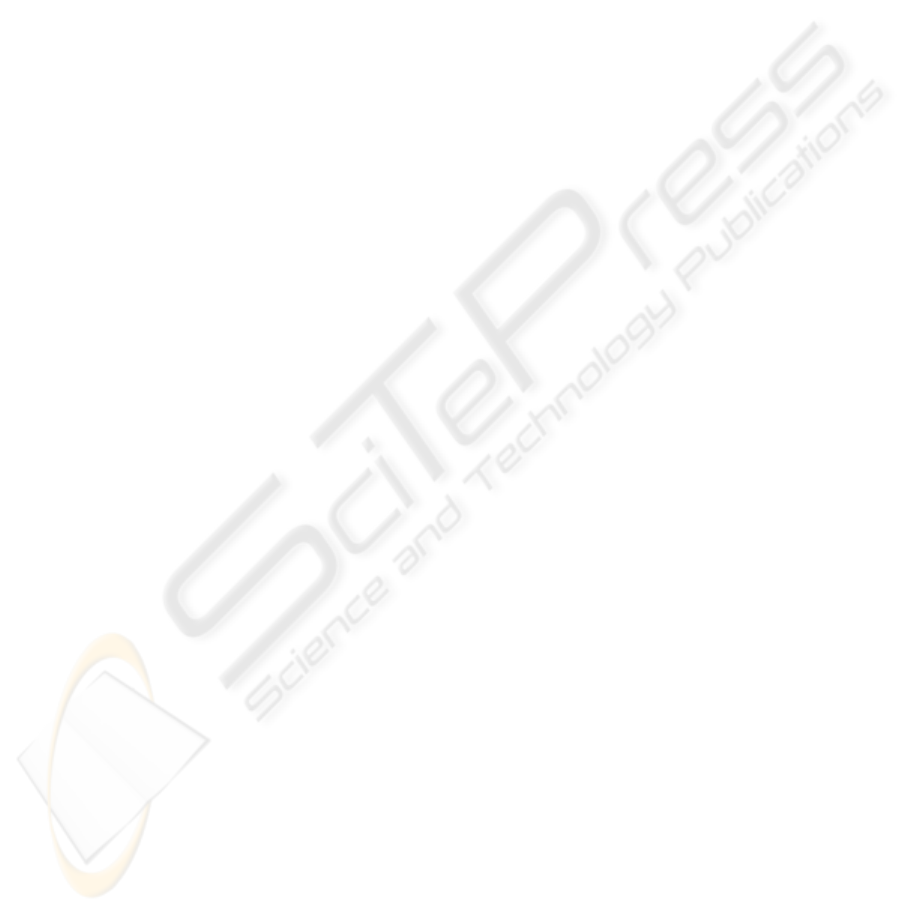
the one, which could be substantiated and used for
the modeling of real processes. Mathematical or
quantitative models are developed, when it is
difficult and time-consuming to develop the physical
model. Within the decision support systems the
analysis is often performed numerically by means of
mathematical models. The main reasons to use the
mathematical models (Turban, 1988):
• They enable to analyze a great, and
sometimes unlimited, number of possible
solutions.
• They help to save time. The operations,
which in reality take several years, can be
performed in a shorter period of time using
computer.
• It is easier to manipulate the model (change
its parameters) than with a real system. It is
easier to carry out experiments, the results of
which do not affect the real system.
• The costs of errors, made during the
experiments, are relatively lower than of
those, made regarding real systems.
• The models enable to calculate the risk of
specific activities.
• The costs of modelling are relatively lower
than similar experimental costs of real
systems.
• It is easier to show the obtained results of
mathematical models graphically – easy to
understand for everybody.
One of the most commonly used actualization
mathematical models of forest stand estimation
indicators in Latvia does not provide the result
necessary for FMP process. For example, when
actualizing average height and diameter of forest
stand, the linear regression model of tree growth
process is used H(A+10) = a0 + a1 * H(A). It is
stated that this model can be used only for the period
of time, which is not longer than 10 years. Thus it
does not solve the planning problem, where the
estimation data should be actualized for the present
moment and several decades in advance. At present
widely applied model is linear and consists only of 2
parameters (a0 and a1), where the values are
determined by the expert. Parameter a0, as a matter
of fact, already shows the imperfection of the model,
because the dimensions of any growing thing (height
and diameter) at the age of 0 are equal to 0.
Parameter a1 indicates the rapidity of the increase of
starting value. This model functions very well for
the data actualization for a short period of time, i.e.
the non-linear phenomenon could be described
within the short interval by means of linear
coherence. However, there emerges a problem
concerning the use of these coefficients for the
particular forecasting of tree growth process for a
longer period of time, because the tree growth
process within the full growth cycle is characterized
by the non-linear coherence. Therefore, it would be
necessary to determine the coefficients of linear
equation repeatedly with a set step, if this model is
used for a different age of forest stand.
The laws of forest growth that we know at
present, do not fully reflect the essence of
phenomena, and they have stochastic nature. Taking
into consideration the great variety of nature, it is
rather difficult to find the functional coherence in it.
Therefore a law, defined mathematically, also
characterizes a separate object only approximately.
In order to describe any regularity in a mathematical
form (as a mathematical formula), it is necessary,
first of all, to find a general form of this
mathematical formula and then, using the
experimental data, calculate the parameters of this
formula. The level of modern computer engineering
(software provision) enables to calculate the
parameters of formulae without any difficulty, while
the informed choice of the general form of equation
is a yet unsolved problem. It is especially related to
the multiple stochastic coherences, which one often
comes across in the research in the area of modelling
growth process. In case with mathematical models, it
is necessary to describe the conditions, under which
these models are characterized by persistent
functioning and are able to provide correct results.
A research performed recently, (Dagis, 2006b)
concerns the mathematical models of tree growth
process, where the following non-linear regression
models of these process were studied:
y(t) = a·t
3
+ b·t
2
+ c·t (1)
y(t) = y
0
·e
-b·t
(2)
y(t) =a·t
k
/ (b
2
+t
k
) (3)
The third polynomial level (1) is viewed, as it
provides good correlation coefficient within the
process of approximation. The polynomial
coefficient has no biological meaning and it does not
represent any growth model, therefore its application
is irrelevant. The exponential model (2) has
biological meaning, it models the limit of tree
growth in time: when increasing the age of tree
growth t, the speed of growth has a tendency
towards zero. But, unfortunately, when
approximating, the correlation coefficient is not as
good as it is in case with Power model (3). Power
model excellently approximates experimental data
with a good correlation coefficient. This
mathematical model was determined as the best one
for the further use to model the tree growth process.
INFORMATION SYSTEM REQUIREMENT ANALYSIS AND SPECIFICATION IN FOREST MANAGEMENT
PLANNING PROCESS
577