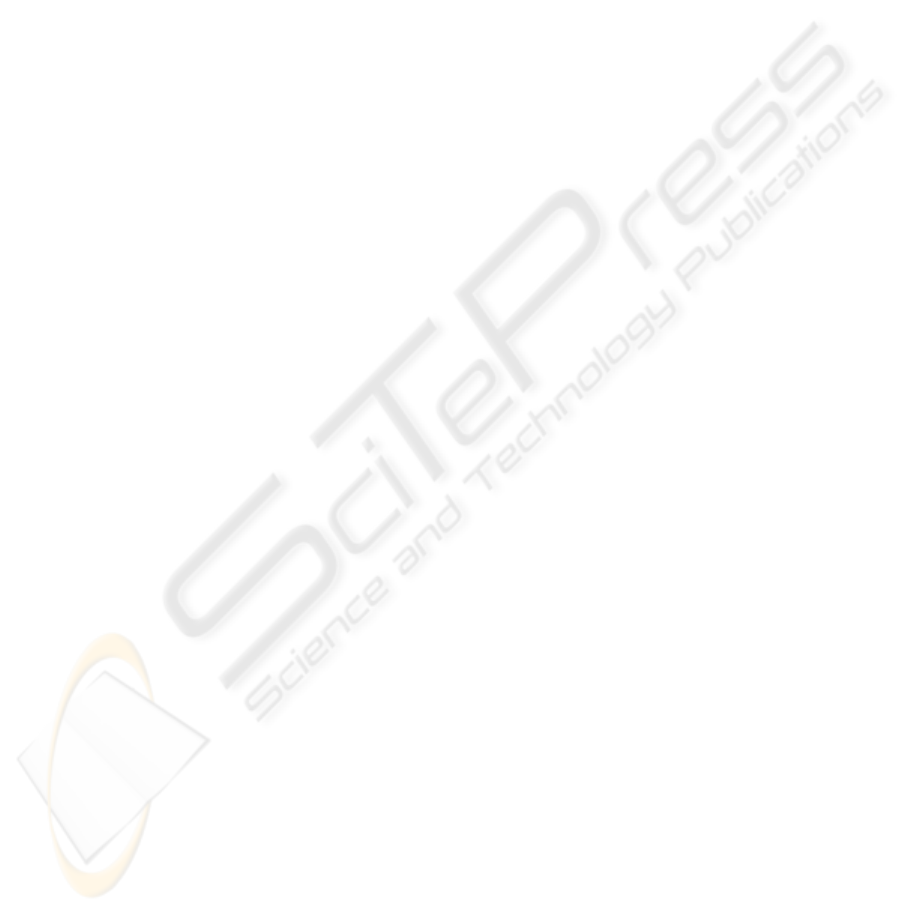
ontologies in user modeling and similarity analysis
in recommender systems has already been developed
(Middleton, Shadbolt, and De Roure, 2004).
A survey of current machine learning techniques
for automatic text classification that can be used to
classify information items into categories of the
taxonomy is provided in (Sebastiani, 2002).
The agent paradigm can be exploited in the
development of information filtering systems such
as recommender systems. Experiences in this area
are described in (Sheth and P. Maes, 1993).
There has been much work done in the domain
of Artificial Intelligence and Law. The development
and usage of legal ontologies to represent and access
legal information has been addressed in (Tiscornia,
2001) and (Valente, 1995). (Benjamins, Casanovas,
Breuker and Gangemi, 2005) provide an overview of
the application of Semantic Web technologies to the
legal domain.
5 CONCLUSIONS
This work described the requirements analysis of
Infonorma multi-agent system. A solution to the
requirements specified here was designed and
implemented also under the guidelines of MAAEM
methodology, although it is not described in this
paper. The next step is to carry out tests with real
legal users and define criteria for measuring the
quality of recommendations.
In the current version of Infonorma users have to
explicitly specify their interests by filling a form.
This is used to create and update the user model and
no feedback is obtained from the user. One research
issue to be addressed in the future is to combine web
usage mining techniques (Girardi and Marinho,
2007) with Semantic Web technologies to support
the implicit acquisition of user profiles and their
dynamic update through user feedback.
The case study described in this article also
contributed for the evaluation of the MAAEM
methodology application analysis phase.
Both MAAEM and ONTORMAS have proved
their usefulness for capturing and specifying
requirements of a specific application through
appropriate guidelines and representation and
decomposition mechanisms.
ACKNOWLEDGEMENTS
This work is supported by CNPq.
REFERENCES
Adomavicius, G., Tuzhilin, A., 2005. Toward the next
generation of recommender systems: A survey of the
state-of-the-art and possible extensions. IEEE Trans.
Knowledge and Data Engineering, v. 17, n. 6, 734-
749.
Antoniou G., Van Harmelen, F., 2004. A Semantic Web
Primer, MIT Press.
Balabanovic, M., Shoham, Y., 1997. Fab: Content-Based,
Collaborative Recommendation Comm. ACM, v. 40, n.
3, 66-72.
Benjamins, V. R., Casanovas, P., Breuker, J., Gangemi,
A., 2005. Law and the Semantic Web, an Introduction.
In Lecture Notes in Computer Science, v. 3369, 1–17.
Drumond L., Girardi. R., Lindoso, A., Marinho, L.,
2006. A Semantic Web Based Recommender System
for the Legal Domain. In Proc. of the European
Conference on Artificial Intelligence (ECAI 2006)
Workshop on Recommender Systems, Riva del Garda,
Italy, pp. 81-83.
Girardi. R., Marinho, L., 2007. A Domain Model of Web
Recommender Systems based on Usage Mining and
Collaborative Filtering, Requirements Engineering
Journal, London, Springer-Verlag Press, v. 12, n. 1,
pp. 23-40.
Gruber, T., 1995. Toward Principles for the Design of
Ontologies used for Knowledge Sharing. International
Journal of Human-Computer Studies, n. 43, 907-928.
Jennings, N., 2000. On Agent-based Software
Engineering. Artificial Intelligence, v. 117, n. 2, 277-
296.
Lindoso, A., Girardi, R., 2006. The SRAMO Technique
for Analysis and Reuse of Requirements in Multi-
agent Application Engineering. IX Workshop on
Requirements Engineering, Cadernos do IME, UERJ
Press, v. 20, 41-50. Rio de Janeiro.
McBride, B., 2002. Jena: a Semantic Web Toolkit.
Internet Computing IEEE, 6, pp. 55-59.
Middleton, S., Shadbolt, N., De Roure, D., 2004.
Ontological User Profiling in Recommender Systems.
ACM Transactions on Information Systems, 22, 54-88.
Sebastiani, F., 2002. Machine learning in automated text
categorization. ACM Computing Surveys.
Sheth, B., Maes, P., 1993. Evolving Agents for
Personalized Information Filtering. In Proc. Ninth
IEEE Conf. Artificial Intelligence for Applications,
345-352.
Tiscornia, D., 2001. Ontology-Driven Access to Legal
Information. DEXA 12th International Workshop on
Database and Expert Systems Applications, p. 792.
Valente, A., 1995. Legal Knowledge Engineering: a
Modeling Approach. IOS Press, Amsterdam, The
Netherlands.
Ziegler, C., 2004, Semantic Web Recommender Systems.
Proc. Joint ICDE/EDBT Ph.D. Workshop,
78-89.
ICEIS 2007 - International Conference on Enterprise Information Systems
160