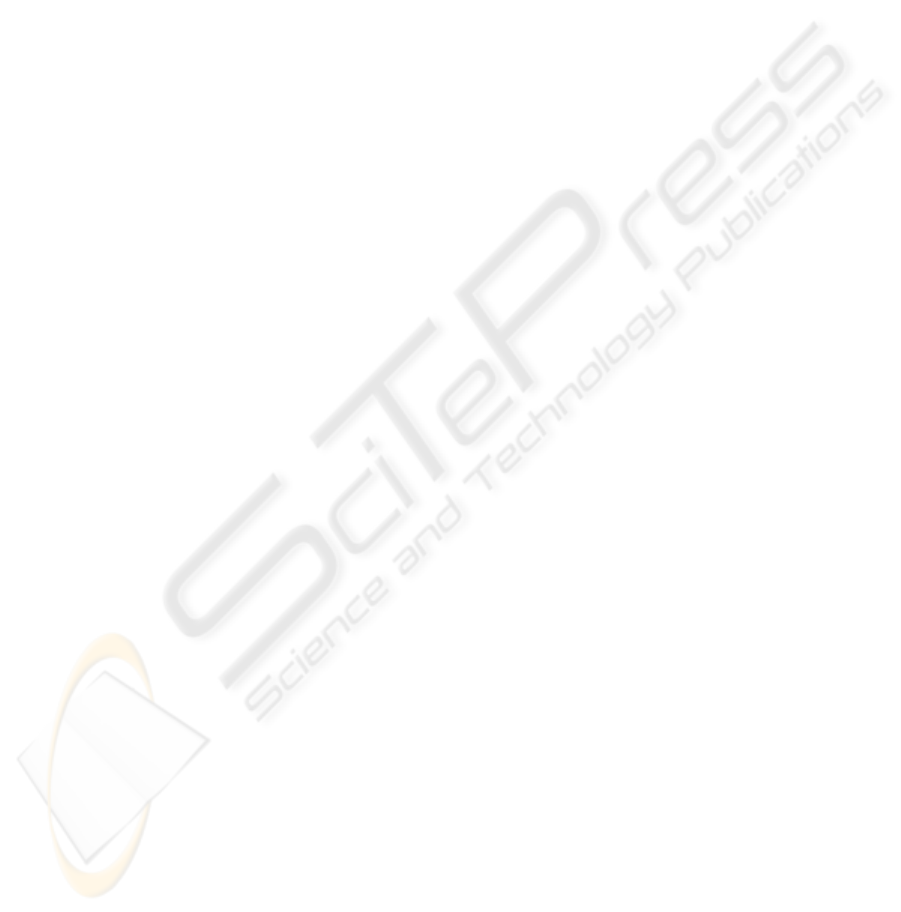
its range of values only to (’TextureDescriptor’, ’Col-
orDescriptor’ or ’PhotometricDescriptor’). During
the user’s formulation, the interface is built dynami-
cally according to the user’s choices and the ontology
content. Therefore, the interface is updated as soon
as new concepts are introduced in the ontology by the
cognitive expert. The formulation system also uses
inference rules to propose default values to the user.
For example, at the physical level, it proposes types of
noise and defects that often degrade images according
to the type of acquisition system.
We conduct experiments with inexperienced users
in the image processing field. They are asked to for-
mulate a problem defining an application using the
human-machine interface. This work allows us to
check if the concepts of the domain ontology are han-
dleable for this kind of users and to enhance the in-
terface ergonomics. It also reveals the difficulties en-
countered during the act of formulation.
Some recent works use application ontologies to
represent visual properties in order to solve a prob-
lem of vision (e.g. in (Koenderink et al., 2006) to
assess the quality of young tomato plant, in (Bom-
bardier et al., 2004) to classify wood defects). These
ontologies are built through meetings between a do-
main expert and an application designer, and they are
specific to the task to be performed. Such ontologies
can be constructed using our system and used, at least,
by the image processing part of the considered appli-
cation.
6 CONCLUSION
Our platform allows to study the image processing
knowledge used in the development of applications. It
is complete since it allows to formulate the problems,
to model the solutions and to rationalize the design
process during their development. Its different com-
ponents help the actors of the platform in their work
and the ontologies permit an effective collaborative
work through their central role.
This work is a contribution to the image process-
ing field because the modeling of the formulation al-
lows to give prominence to the knowledge used in the
development of such applications. It defines a guide-
line on the way we have to tackle such applications
and identifies their formulation elements. The ex-
plicitness of these elements is very useful to acquire
the image processing knowledge used by the planning
system: they are used to justify the choice of the algo-
rithms regarding the application context and therefore
to define the conditions of applicability of the image
processing techniques. Hence, it also enhances the
evaluation and favors the reusability of solution parts.
REFERENCES
Bloehdorn, S., Petridis, K., Saathoff, C., Simou, N., Tzou-
varas, V., Avrithis, Y., Handschuh, S., Kompatsiaris,
Y., Staab, S., and Strintzis, M. G. (2005). Semantic
annotation of images and videos for multimedia anal-
ysis. In ESWC, volume 3532 of LNCS, pages 592–
607. Springer.
Bombardier, V., Lhoste, P., and Mazaud, C. (2004).
Mod
´
elisation et int
´
egration de connaissances m
´
etier
pour l’identification de d
´
efauts par r
`
egles linguistiques
floues. Traitement du Signal, 21(3):227–247.
Chien, S. and Mortensen, H. (1996). Automating image
processing for scientific data analysis of a large image
database. IEEE PAMI, 18(8):854–859.
Cl
´
ement, V. and Thonnat, M. (1993). A knowledge-based
approach to integration of image procedures process-
ing. CVGIP: Image Understanding, 57(2):166–184.
Clouard, R., Elmoataz, A., Porquet, C., and Revenu, M.
(1999). Borg : A knowledge-based system for auto-
matic generation of image processing programs. IEEE
PAMI, 21(2):128–144.
Draper, B., Hanson, A., and Riseman, E. (1996).
Knowledge-directed vision : Control, learning, and
integration. In Proc. of IEEE, volume 84, pages 1625–
1681.
Hudelot, C. and Thonnat, M. (2003). A cognitive vision
platform for automatic recognition of natural complex
objects. In Proc. of the 15th IEEE ICTAI, page 398,
Washington, DC, USA. IEEE Computer Society.
Koenderink, N. J. J. P., Top, J. L., and van Vliet, L. J.
(2006). Supporting knowledge-intensive inspection
tasks with application ontologies. Int. J. Hum.-
Comput. Stud., 64(10):974–983.
Maillot, N., Thonnat, M., and Boucher, A. (2004). To-
wards Ontology Based Cognitive Vision (Long Ver-
sion). Machine Vision and Applications, 16(1):33–40.
Nouvel, A. and Dalle, P. (2002). An interactive approach for
image ontology definition. In 13
`
eme Congr
`
es de Re-
connaissance des Formes et Intelligence Artificielle,
pages 1023–1031, Angers, France.
Renouf, A., Clouard, R., and Revenu, M. (2007). How to
formulate image processing applications ? In Pro-
ceedings of the International Conference on Computer
Vision Systems, Bielefeld, Germany.
Schreiber, G., Wielinga, B., Akkermans, H., Van de Velde,
W., and Anjewierden, A. (1994). CML: The Com-
monKADS Conceptual Modelling Language. In
Steels, L., Schreiber, G., and de Velde, W. V., editors,
EKAW 94, volume 867 of Lecture Notes in Computer
Science, pages 1–25, Hoegaarden, Belgium. Springer
Verlag.
Town, C. (2006). Ontological inference for image and video
analysis. Mach. Vision Appl., 17(2):94–115.
ICEIS 2007 - International Conference on Enterprise Information Systems
276