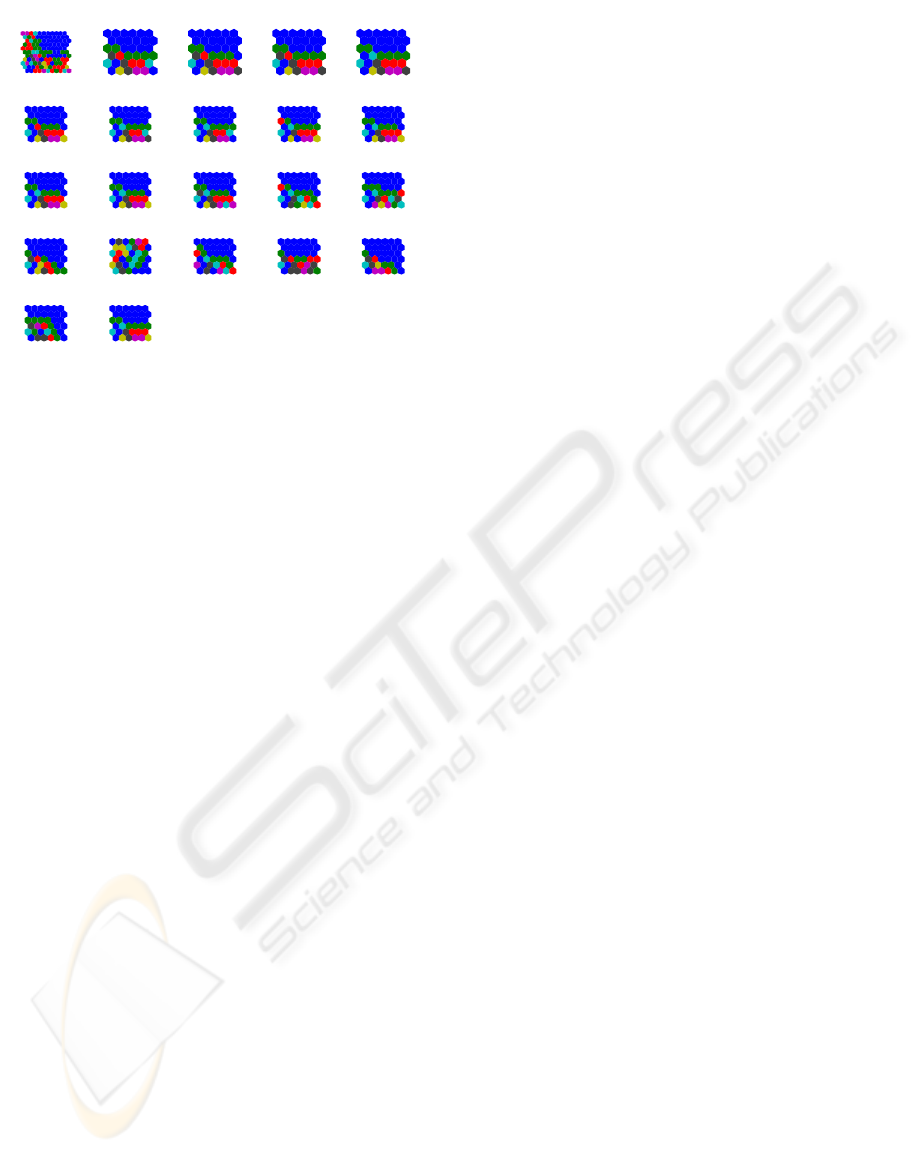
them show similarities. The results are presented in
2D maps.
The trouble in clustering the cities of Rio de
Janeiro (67) and Volta Redonda (91) was due to the
fact that both showed energy consumption above
average, making them different from the others. As a
matter of fact, Rio de Janeiro shows large energy
consumption in all variables except rural
consumption. Volta Redonda also shows this
characteristic in less volume but significantly in the
industrial energy consumption due to its steel
industry.
Although the results given by the Kohonen neural
nets showed a great deal of homogeneity in the
clustering formation, it is expected a classification
improvement if more variables are inserted such as
the city area, number of inhabitants and some
economical variable e. g. per capita income.
REFERENCES
Tanure, J.E,P.S., Carvalho, E.B., 2000. Regulation by
performance comparison for establishing goals for
continuity in energy dispensing. In National Workshop
on Electrical Energy Distribution, Foz de Iguaçu,
Brazil, 2000, in Portuguese.
Tou, J. T., 1974. Pattern recognition principles. Addison-
Wesley Publishing Company. Massachusetts, 2
nd
edition.
Anderberg, M.R. 1973. Cluster analysis for applications.
Academic Press.
Queiroz, H. L., Borba, C. M., 2001. Methodology for set
evaluation by means of quality technical indices. In
Electrical Energy Distribution Systems, Puerto
Iguaçu, Argentina, in Portuguese.
Sperandio, M., Coelho, J., Queiroz, H. L. , 2003.
Identification of clusters of energy consumption users
using self-organizing maps. In Proceedings of the V
SBQEE, Aracaju - Brazil, in Portuguese.
Ramos, A. J. P., 2000. Control and assessment of
electrical energy quality. In Technical Report 2 –
Monitoring and Instrumentation Protocol, in
Portuguese.
Kohonen, T., 2001. Self-organizing maps. 3rd ed.
Springer-Verlag, Berlin.
Haykin, S. , 2001. Neural networks: a comprehensive
foundation. Prentice Hall, 2nd ed., Upper Saddle
River: New Jersey.
Kohonen, T., Hynninen, J., Kangas J., Laaksonen J., 1996.
SOM_PAK: The self-organizing map program
package, Technical Report A31. In Technical Report
A31, Helsinki University of Technology, 1996,
available in http://www.cis.hut.fi/nnrc/nnrc-
programs.html.
Willshaw, W. D. J., Buneman, O. P., Longuet-Higgins, H.
C., 1969. Non-holographic associative memory. In
Nantre, London, voI.222, pp.960-962.
Willshaw, D. J., von der Malsburg ,C., 1976. How
patterned neural connections can be set up by self-
organization. In Proceedings of the Royal Society of
London Series B. voI.194, pp.431-445.
Dryden, I.. L. , Mardia, K.V., 1998. Statistical shape
analysis. John Wiley: Chichester.
Michie, D., Spiegelhalter D. J., Taylor, C. C., 1994.
Machine learning, neural and statistical classification.
Ellis Horwood, pp. 35-36.
Akkus, A., Guvernir H. A., 1996. K-Nearest neighbour
classification on feature projections. In Proceedings of
ICML’96 Lorenza Saitta (ed.). Proceedings of ICML
1996, Bari, Italy.
Mitra, P., Murthy, C.A., Pal , S.K., 2002. Unsupervised
feature election using feature similarity. In IEEE
Transactions on Pattern Analysis and Machine
Intelligence, vol. 24, no. 3.
Bishop, C. M., 1995. Neural networks for pattern
recognition. New York, Oxford University Press.
U-mat rix JAN FEB MAR APR
MAY JUN JUL AUG SEP
OCT NOV DEC RESIDENTIAL INDUSTRIAL
COMMERCIAL RURAL P. ILLUMINATION P. SERVICES P. POWER
S. CONSUMPTION TOTAL
Figure 5: Overview of the Clustering Problem.
ICEIS 2007 - International Conference on Enterprise Information Systems
450