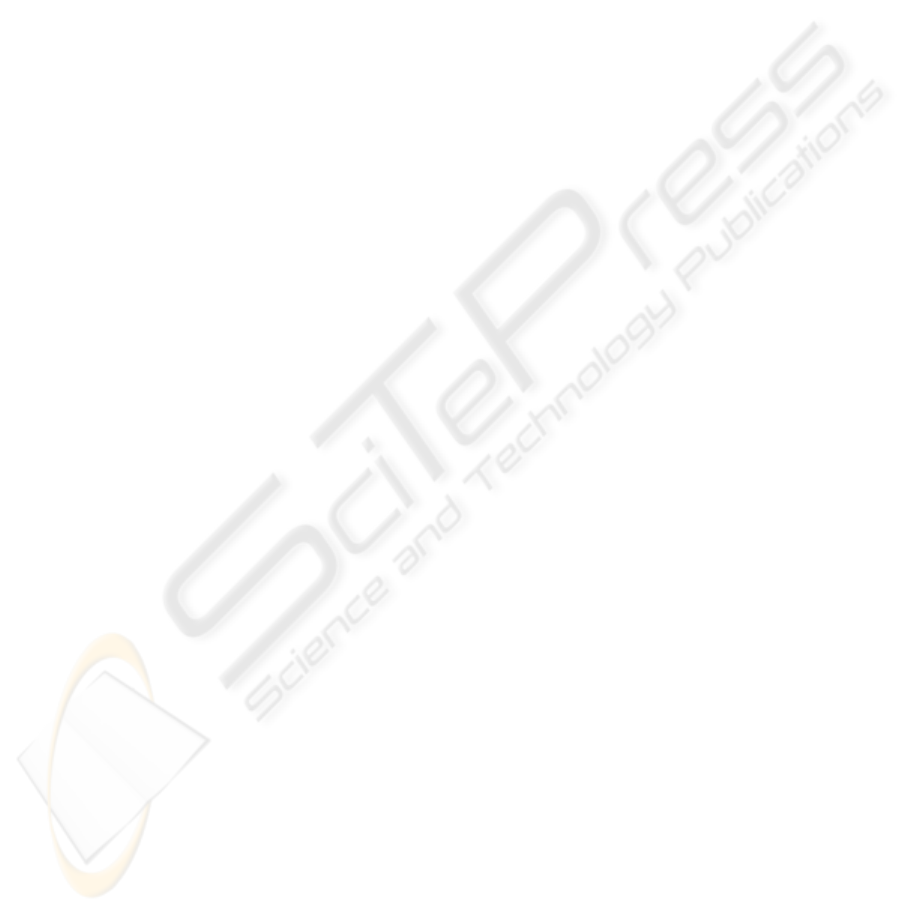
lief system regarding the possible types of their po-
tential partners and update their beliefs in a reinforce-
ment learning based approach in repeated games sce-
nario. As mentioned above, our work differs from
theirs in that we do not pre-assume any probabilis-
tic models in particular, and that our model assumes
the more general problem of non-transferable utilities
games, instead of transferable one.
A solution concept for coalition game with
stochastic payoff is presented in (Suijs et al., 1999). In
this approach, the payoffs (i.e., the consequences) are
assumed to be stochastic variables, and agents pref-
erences over those stochastic variables are used to de-
termine the stability of a coalition. Thus, their work is
on stochastic games, whereas our focus is on a more
general class of non-transferable utility game that are
not necessarily probabilistic in nature.
A mechanism for forming coalition under uncer-
tainty is proposed in (Kraus et al., 2003; Kraus et al.,
2004). In these two works, a multi-rounds mecha-
nism is proposed where, in each round, the agents are
arranged in a certain order and they make coalition
formation proposals or accept proposals in that order.
The mechanism repeats until each agent belongs to a
coalition. Again, the difference is that their approach
is based on the assumption of transferable utility and
the kernel is used as the stability concept, which is not
our assumption.
9 CONCLUSION
Classical coalition formation concepts in game the-
ory are deterministic in nature. That is, they as-
sume the value of each coalition to be publicly known
for certain. However this assumption is not practi-
cal in many software agent applications where intel-
ligent agents have to rely on whatever evidences they
can perceive or their past experience to estimate such
coalition values. The probabilistic approaches pro-
vide a good alternative in many cases, but are not suit-
able in some multi-agent applications where the sam-
ples are sparse and where the agents utility are non-
transferable. In this paper, we propose a new type of
game which we label non-transferable utility games
with uncertainty, and provide a new concept for de-
scribing the stability of coalitions under uncertainties,
namely, the CU-core. By doing so, we are able to
provide useful stability concepts for this new type of
game which otherwise cannot be handled using the
classic deterministic approaches or the probabilistic
approaches. We believe our model provide a useful
tool in evaluating coalition formation algorithms for
cooperative games under uncertainty.
REFERENCES
Blankenburg, B. and Klusch, M. (2004). Pon safe ker-
nel stable coalition forming among agents. In Pro-
ceedings of the Third International Joint Conference
on Autonomous Agents and Multiagent Systems, vol-
ume 2, pages 580–587.
Chalkiadakis, G. and Boutilier, C. (2004). Bayesian rein-
forcement learning for coalition formation under un-
certainty. In Proceedings of the Third International
Joint Conference on Autonomous Agents and Multia-
gent Systems, pages 1090–1097.
Gillies, D. B. (1959). Solutions to general non-zero-sum
games. InTucker, A. W. and Luce, R. D., editors, Con-
tributions to the Theory of Games Volume IV. Prince-
ton University Press.
He, L. and Ioerger, T. (2004). Combining bundle search
with buyer coalition formation in electronic markets:
A distributed approach through negotiation. In Pro-
ceedings of the Third International Joint Conference
on Autonomous Agents and Multiagent Systems, pages
1440–1441.
Ketchpel, S. (1994). Forming coalitions in the face of uncer-
tain rewards. In Proceedings of National Conference
on Artificial Intelligence (AAAI-94), pages 414–419.
Kraus, S., Shehory, O., and Taase, G. (2003). Coalition for-
mation with uncertain heterogeneous information. In
Proceedings of the Third International Joint Confer-
ence on Autonomous Agents and Multiagent Systems,
pages 1–8.
Kraus, S., Shehory, O., and Taase, G. (2004). The advan-
tages of compromising in coalition formation with in-
complete information. In Proceedings of the Third In-
ternational Joint Conference on Autonomous Agents
and Multiagent Systems, pages 588–595.
Osborne, M. J. and Rubinstein, A. (1994). A Course in
Game Theory. The MIT Press.
Plaza, E. and Mcginty, L. (2005). Distributed case-
based reasoning. The knowledge Engineering Review,
00:01-4.
Sandholm, T. (1999). Distributed rational decision making.
In Weiss, G., editor, Multiagent Systems A Modern
Approach to Distributed Artificial Intelligence. The
MIT Press.
Scarf, H. (1967). The core of n person game. Econometrica,
35(1):50–59.
Suijs, J., Borm, P., Waegenaere, A. D., and Tijs, S. (1999).
Cooperative games with stochastic payoffs. European
Journal of Operational Reseach, 133.
Yamamoto, J. and Sycara, K. (2001). A stable and efficient
buyer coalition formation scheme for e-marketplaces.
In Proceedings of the Fifth International Conference
on Autonomous Agents, pages 576–583.
RULE BASED STABILITY CRITERIA FOR COALITION FORMATION UNDER UNCERTAINTY
177