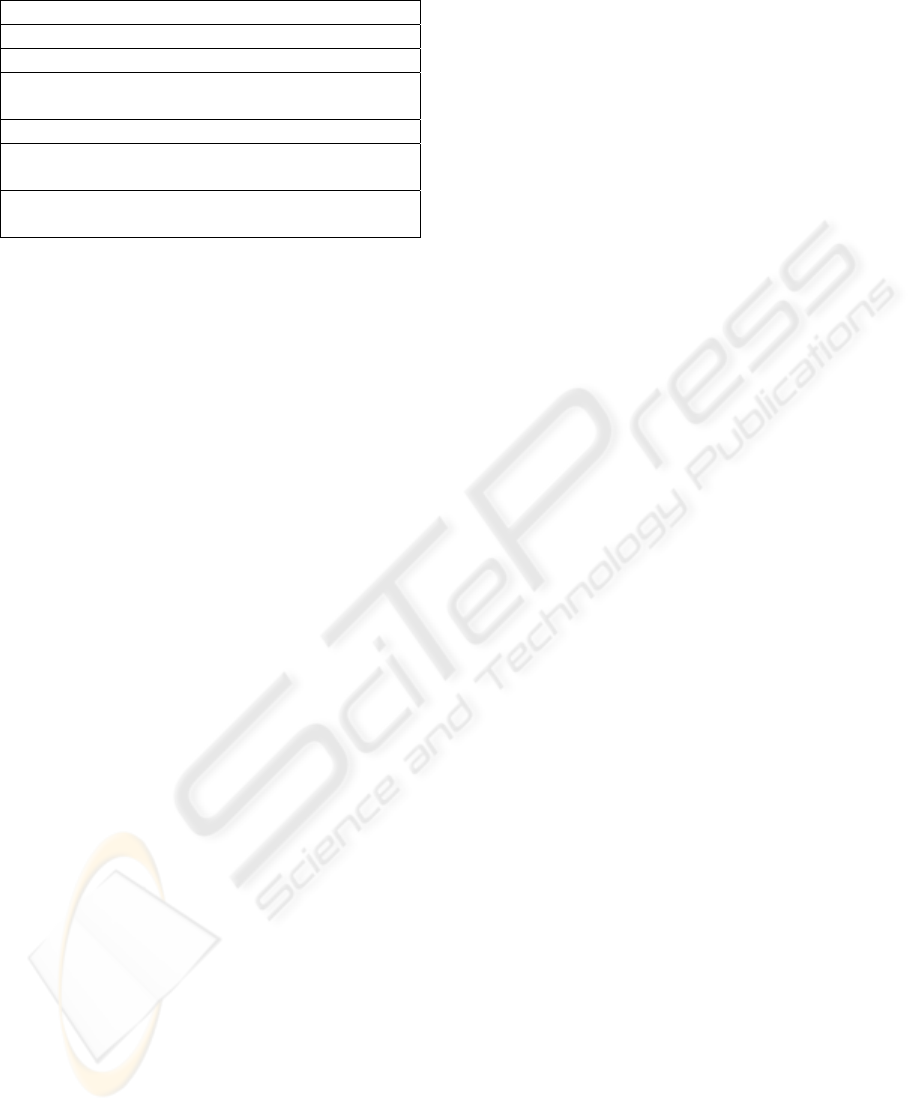
Table 6: Benefits of RDS Defect Tracker.
RDS Defect Tracker - Benefits
1. Centralized Information System
2. Feedback mechanism Æ RPN values
3. Streamlines process for recording, tracking
and updating identified defects
4. Reduces uncertainty: Reliability Prediction
5. GUI interface, customized reports, alert
updates, and statistical analysis capabilities
6. Proposed neural network module – makes
reliability and design teams more proactive.
As previously mentioned, most commercial
software programs focuses on estimating reliability
or RPN values using MIL Standards or empirical
data. This may result in erroneous conclusions
regarding the system reliability. The RDS Defect
Tracker, on the other hand, uses defect data from
testing of the RDS, thereby providing a more
accurate measure of the system MTTF and
reliability.
Some of the limitations of the RDS Defect
Tracker are discussed below.
Data Accuracy – Requires accurate data for
performing the FMEA and predicting the
system reliability. Potential “noise” in the data
would have an adverse impact on the
reliability measures.
Scalability – As more data becomes available,
the overall response time of the RDS Defect
Tracker is expected to decrease, as a result of
the increasing complexity. Reconstructability
Analysis (RA) or similar techniques should be
employed to reduce this complexity by
monitoring only key variables that are
required to estimate the reliability or to
conduct an FMEA.
The following are the potential extensions to this
research:
Simulation of failure modes – A simulation of
the various failure modes can help analyze the
system performance under stress. An
accelerated stress testing module would be
beneficial to the design team to study the
impact of design changes.
Obtain field data – In order to obtain the
performance of the RDS in the field, the RDS
Defect Tracker should be integrated in the
RDS, sending any defect information to a
central data warehouse. This would help in
determining whether any updates to the design
or engineering changes are necessary.
The application of the RDS Defect Tracker is not
limited to the domain discussed in this paper. The
methodology presented can be applied to other
domain fields especially if introducing a new
product, or during a design for six sigma (or DFSS)
process.
REFERENCES
Bevilacqua, M., Braglia, M., Frosolini, M., Montanari, R.,
2005. Failure rate prediction with artificial neural
networks, Journal of Quality in Maintenance
Engineering, 11(3), pp. 279-294.
Brietler, A. L., Sloan, C. D., 2005. System reliability
prediction: Towards a general approach using a neural
network. In Proceedings, U.S. Air Force T&E Days.
American Institute of Aeronautics and Astronautics,
Inc.
Chen, K. 2006. Forecasting systems reliability based on
support vector regression with genetic algorithms
Reliability Engineering and System Safety, In Press,
Corrected Proof, Available [28 Feb 2006].
Coit, D. W., Jin., T., 2001. Prioritizing system-reliability
prediction improvements. IEEE Transactions on
Reliability, 50(1), pp. 17-25.
Price, C. J., Taylor, N. S., 2002. Automated multiple
failure FMEA. Reliability Engineering and System
Safety, 76, pp. 1-10.
Puente, J., Pino, R., Priore, P., Fuente, D., 2002. A
Decision support system for applying failure mode and
effects analysis. International Journal of Quality and
Reliability Management, 19(2), pp. 137-150.
Ramakrishnan, S., Sturek, J., Nagula, P., Srihari, K., 2006.
A systems approach to predict the reliability of a
robotic dispensing system. International Journal of
General Systems, pending submission.
Theije, S, M., Sander, P, C., Brombacher, A, C., 1998.
Reliability tests to control design quality: A case
study. International Journal of Quality and Reliability
Management, 15(6), pp. 599-618.
ICEIS 2007 - International Conference on Enterprise Information Systems
296