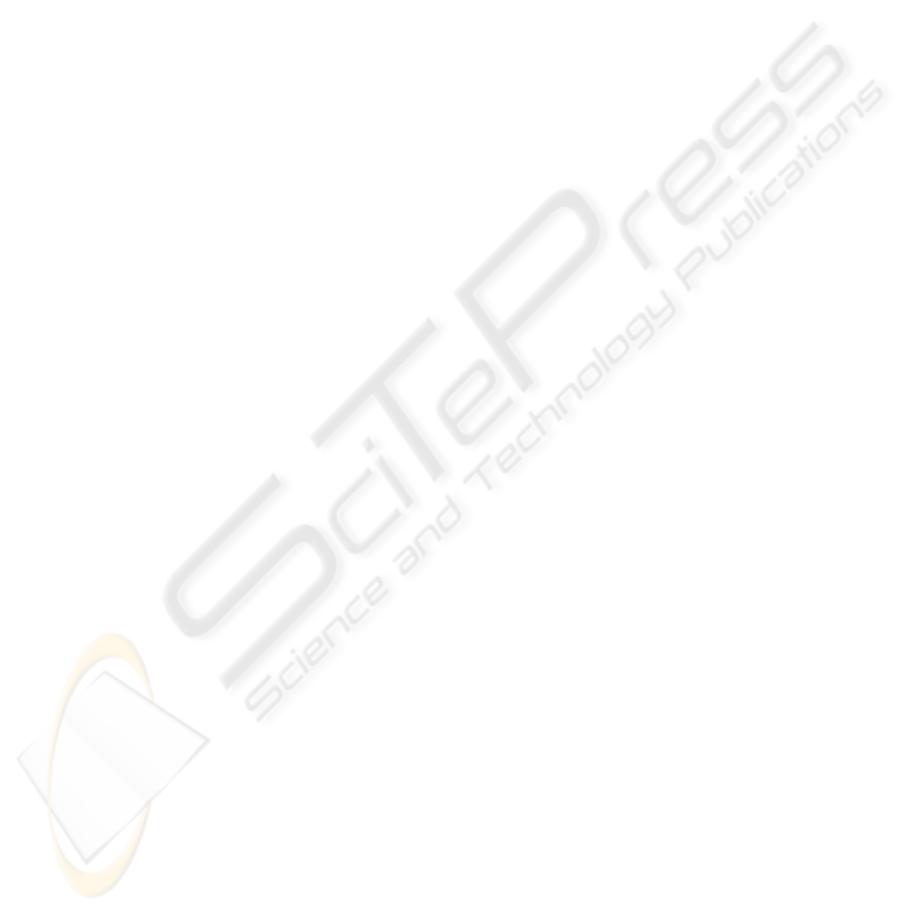
when ASR represents only one part of a complex (e.g. multi-modal) interaction system
computational facilities for adaptation are rather limited.
Addressing such interactive applications with computational restrictions we pre-
sented a rejection model based on the evaluation of confidence scores applying log-odd
scores for semi-continuous Hidden Markov Models. For this simple normalization tech-
nique, which can be applied in advance thus maximally limiting the additional compu-
tational effort, the ratio of actual acoustic scores as obtained by HMM evaluation to a
reasonable background model is computed. Based on these values hypotheses’ scores
can directly be compared to an absolute threshold and rejected for adaptation if neces-
sary. Two variants of the background model based either on a uniform distribution of
mixture coefficients involved or their prior probabilities have been investigated.
The effectiveness of our approach has been demonstrated by means of experimental
evaluations on two challenging tasks. Therefore, MLLR adaptation has been applied
to speaker-independent base systems processing data sets containing both in-domain
and out-of domain utterances. This corresponds to a very common scenario in e.g.
human-robot interaction where often adaptation data out-of-the-scope of the recognizer
(lexicon etc.) need to be processed. When applying the rejection model the adaptation
process for interactive speech recognition applications can be improved substantially.
References
1. Huang, X., Acero, A., Hon, H.: Spoken Language Processing – A Guide to Theory, Algo-
rithm, and System Development. Prentice Hall PTR (2001)
2. Leggetter, C.J., Woodland, P.C.: Maximum likelihood linear regression for speaker adapta-
tion of continuous density Hidden Markov Models. Computer Speech & Language (1995)
171–185
3. Pitz, M., et al.: Improved MLLR speaker adaptation using confidence measures for conver-
sational speech recognition. In: Int. Conf. Spoken Lang. Proc. (2000)
4. Plötz, T., Fink, G.A.: Robust time-synchronous environmental adaptation for continuous
speech recognition systems. In: Int. Conf. Spoken Lang. Proc. Volume 2. (2002) 1409–1412
5. Zhang, Z., Furui, S., Ohtsuki, K.: On-line incremental speaker adaptation with automatic
speaker change detection. In: Proc. Int. Conf. on Acoustics, Speech, and Signal Processing.
(2000)
6. Wessel, F., Schlüter, R., Macherey, K., Ney, H.: Confidence measures for large vocabulary
continuous speech recognition. IEEE Trans. on Speech and Audio Processing 91 (2001)
7. Chase, L.: Word and acoustic confidence annotation for large vocabulary speech recognition.
In: Proc. European Conf. on Speech Communication and Technology. (1997)
8. Feng, J., Sears, A.: Using confidence scores to improve hands-free speech-based navigation
in continuous dictation systems. ACM Transactions on Computer-Human Interaction 11
(2004) 329–356
9. Durbin, R., Eddy, S., Krogh, A., Mitchison, G.: Biological sequence analysis: Probabilistic
models of proteins and nucleic acids. Cambridge University Press (1998)
10. Huang, X.D., Jack, M.A.: Semi-continuous Hidden Markov Models for speech signals. Com-
puter Speech & Language 3 (1989) 239–251
11. Haasch, A., et al.: BIRON – The Bielefeld Robot Companion. In: Proc. Int. Workshop on
Advances in Service Robotics, Fraunhofer IRB Verlag (2004) 27–32
12. Schillo, C.: Der SLACC Korpus. Technical report, Faculty of Technology, Bielefeld Univer-
sity (2001)
117